新品:
-12% ¥21,418¥21,418 税込
ポイント: 214pt
(1%)
配送料 ¥600 2月25日-3月5日にお届け
発送元: BD SG 販売者: BD SG
中古品 - 良い
¥17,517¥17,517 税込
発送元: Amazon 販売者: 猫象書店 在庫確実!!年中無休で、超!迅速発送!!買います!ご不要の雑誌/本/洋書等を着払で!
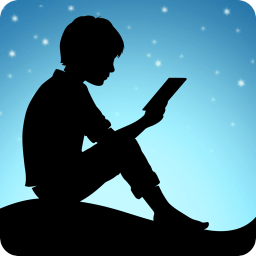
無料のKindleアプリをダウンロードして、スマートフォン、タブレット、またはコンピューターで今すぐKindle本を読むことができます。Kindleデバイスは必要ありません。
ウェブ版Kindleなら、お使いのブラウザですぐにお読みいただけます。
携帯電話のカメラを使用する - 以下のコードをスキャンし、Kindleアプリをダウンロードしてください。
Learning to Rank for Information Retrieval ハードカバー – 2011/5/6
購入オプションとあわせ買い
Due to the fast growth of the Web and the difficulties in finding desired information, efficient and effective information retrieval systems have become more important than ever, and the search engine has become an essential tool for many people.
The ranker, a central component in every search engine, is responsible for the matching between processed queries and indexed documents. Because of its central role, great attention has been paid to the research and development of ranking technologies. In addition, ranking is also pivotal for many other information retrieval applications, such as collaborative filtering, definition ranking, question answering, multimedia retrieval, text summarization, and online advertisement. Leveraging machine learning technologies in the ranking process has led to innovative and more effective ranking models, and eventually to a completely new research area called “learning to rank”.
Liu first gives a comprehensive review of the major approaches to learning to rank. For each approach he presents the basic framework, with example algorithms, and he discusses its advantages and disadvantages. He continues with some recent advances in learning to rank that cannot be simply categorized into the three major approaches – these include relational ranking, query-dependent ranking, transfer ranking, and semisupervised ranking. His presentation is completed by several examples that apply these technologies to solve real information retrieval problems, and by theoretical discussions on guarantees for ranking performance.
This book is written for researchers and graduate students in both information retrieval and machine learning. They will find here the only comprehensive description of the state of the art in a field that has driven the recent advances in search engine development.
- 本の長さ302ページ
- 言語英語
- 出版社Springer
- 発売日2011/5/6
- 寸法15.75 x 2.03 x 23.37 cm
- ISBN-103642142664
- ISBN-13978-3642142666
商品の説明
レビュー
From the reviews:
“The book treats a very hot research topic: that of ranking great amounts of documents based on their relation to a given query, i.e., the examination of the inner mechanics of the search engines. The text is especially addressed to information retrieval and machine learning specialists and graduate students, but it might appeal to scientists from other related fields, too.” (Ruxandra Stoean, Zentralblatt MATH, Vol. 1227 2012)
著者について
Tie-Yan Liu is a lead researcher at Microsoft Research Asia. He leads a team working on learning to rank for information retrieval, and graph-based machine learning. So far, he has more than 70 quality papers published in referred conferences and journals, including SIGIR(9), WWW(3), ICML(3), KDD, NIPS, ACM MM, IEEE TKDE, SIGKDD Explorations, etc. He has about 40 filed US / international patents or pending applications on learning to rank, general Web search, and multimedia signal processing. He is the co-author of the Best Student Paper for SIGIR 2008, and the Most Cited Paper for the Journal of Visual Communication and Image Representation (2004~2006). He is an Area Chair of SIGIR 2009, a Senior Program Committee member of SIGIR 2008, and Program Committee members for many other international conferences, such as WWW, ICML, ACL, and ICIP. He is the co-chair of the SIGIR workshop on learning to rank for information retrieval (LR4IR) in 2007 and 2008. He has been on the Editorial Board of the Information Retrieval Journal (IRJ) since 2008, and is the guest editor of the special issue on learning to rank of IRJ. He has given tutorials on learning to rank at WWW 2008 and SIGIR 2008. Prior to joining Microsoft, he obtained his Ph.D. from Tsinghua University, where his research efforts were devoted to video content analysis.
登録情報
- 出版社 : Springer; 第2011版 (2011/5/6)
- 発売日 : 2011/5/6
- 言語 : 英語
- ハードカバー : 302ページ
- ISBN-10 : 3642142664
- ISBN-13 : 978-3642142666
- 寸法 : 15.75 x 2.03 x 23.37 cm
- Amazon 売れ筋ランキング: - 472,558位洋書 (洋書の売れ筋ランキングを見る)
- - 293位Data Warehousing
- - 328位Pattern Recognition Algorithms
- - 409位Computer Network Administration
- カスタマーレビュー:
著者について
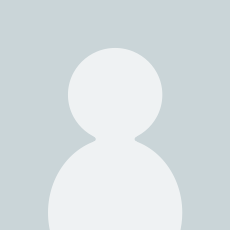
著者の本をもっと見つけたり、似たような著者を調べたり、おすすめの本を読んだりできます。
カスタマーレビュー
- 星5つ星4つ星3つ星2つ星1つ星5つ50%0%50%0%0%50%
- 星5つ星4つ星3つ星2つ星1つ星4つ50%0%50%0%0%0%
- 星5つ星4つ星3つ星2つ星1つ星3つ50%0%50%0%0%50%
- 星5つ星4つ星3つ星2つ星1つ星2つ50%0%50%0%0%0%
- 星5つ星4つ星3つ星2つ星1つ星1つ50%0%50%0%0%0%
上位レビュー、対象国: 日本
他の国からのトップレビュー
-
greg mcmahan2021年12月29日にアメリカ合衆国でレビュー済み
5つ星のうち5.0 Excellent Overview
Amazonで購入This book is written by the authors of Microsoft's lightgbm. It gives an overview of major mathematical developments in learning to rank while being easy to read.
Also introduces standard learning to rank datasets and how many standard ltr models performed on each.
After reading, I see learning to rank as a separate problem from classification and regression. I will write different code due to this book.
It is a great compliment to Intro to Statistical Learning in R or Elements of Statistical Learning. Buy this book!