Papers by Andrei Zinovyev
2022 International Joint Conference on Neural Networks (IJCNN)
Intrinsic dimensionality (ID) is one of the most fundamental characteristics of multi-dimensional... more Intrinsic dimensionality (ID) is one of the most fundamental characteristics of multi-dimensional data point clouds. Knowing ID is crucial to choose the appropriate machine learning approach as well as to understand its behavior and validate it. ID can be computed globally for the whole data point distribution, or computed locally in different regions of the data space. In this paper, we introduce new local estimators of ID based on linear separability of multi-dimensional data point clouds, which is one of the manifestations of concentration of measure. We empirically study the properties of these estimators and compare them with other recently introduced ID estimators exploiting various effects of measure concentration. Observed differences between estimators can be used to anticipate their behaviour in practical applications.
ABSTRACT 1. Three main approaches to chemical kinetics simplification: 1a. Quasi steady state app... more ABSTRACT 1. Three main approaches to chemical kinetics simplification: 1a. Quasi steady state approximation (“fast reagents” or “radicals”); 1b. Quasi-equilibrium approximation (“fast reactions”); 1c. Limiting steps 2. Kinetic of linear networks 3. Idea of limitation. Simple examples 4. Model reduction before model creation: constant ordering versus constant values 5. Catalytic cycle with limiting step 6. Auxiliary discrete dynamic systems 7. Cycles surgery 8. Example: prism of reactions
Tumor development is characterized by a compromised balance between cell life and death decision ... more Tumor development is characterized by a compromised balance between cell life and death decision mechanisms, which are tighly regulated in normal cells. Understanding this process provides insights for developing new treatments for fighting with cancer. We present a study of a mathematical model describing cellular choice between survival and two alternative cell death modalities: apoptosis and necrosis. The model is implemented in discrete modeling formalism and allows to predict probabilities of having a particular cellular phenotype in response to engagement of cell death receptors. Using an original parameter sensitivity analysis developed for discrete dynamic systems, we determine the critical parameters affecting cellular fate decision variables that appear to be critical in the cellular fate decision and discuss how they are exploited by existing cancer therapies.
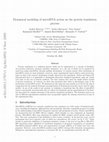
Protein translation is a multistep process which can be represented as a cascade of biochemical r... more Protein translation is a multistep process which can be represented as a cascade of biochemical reactions (initiation, ribosome assembly, elongation, etc.), the rate of which can be regulated by small non-coding microRNAs through multiple mechanisms. It remains unclear what mechanisms of microRNA action are most dominant: moreover, many experimental reports deliver controversal messages on what is the concrete mechanism actually observed in the experiment. Parker and Nissan (Parker and Nissan, RNA, 2008) demonstrated that it is impossible to distinguish alternative biological hypotheses using the steady state data on the rate of protein synthesis. For their analysis they used two simple kinetic models of protein translation. In contrary, we show that dynamical data allow to discriminate some of the mechanisms of microRNA action. We demonstrate this using the same models as in (Parker and Nissan, RNA, 2008) for the sake of comparison but the methods developed (asymptotology of bioche...
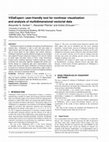
ViDaExpert is a tool for visualization and analysis of multidimensional vectorial data. ViDaExper... more ViDaExpert is a tool for visualization and analysis of multidimensional vectorial data. ViDaExpert is able to work with data tables of "object-feature" type that might contain numerical feature values as well as textual labels for rows (objects) and columns (features). ViDaExpert implements several statistical methods such as standard and weighted Principal Component Analysis (PCA) and the method of elastic maps (non-linear version of PCA), Linear Discriminant Analysis (LDA), multilinear regression, K-Means clustering, a variant of decision tree construction algorithm. Equipped with several user-friendly dialogs for configuring data point representations (size, shape, color) and fast 3D viewer, ViDaExpert is a handy tool allowing to construct an interactive 3D-scene representing a table of data in multidimensional space and perform its quick and insightfull statistical analysis, from basic to advanced methods.
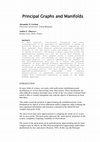
In many physical, statistical, biological and other investigations it is desirable to approximate... more In many physical, statistical, biological and other investigations it is desirable to approximate a system of points by objects of lower dimension and/or complexity. For this purpose, Karl Pearson invented principal component analysis in 1901 and found 'lines and planes of closest fit to system of points'. The famous k-means algorithm solves the approximation problem too, but by finite sets instead of lines and planes. This chapter gives a brief practical introduction into the methods of construction of general principal objects, i.e. objects embedded in the 'middle' of the multidimensional data set. As a basis, the unifying framework of mean squared distance approximation of finite datasets is selected. Principal graphs and manifolds are constructed as generalisations of principal components and k-means principal points. For this purpose, the family of expectation/maximisation algorithms with nearest generalisations is presented. Construction of principal graphs wit...
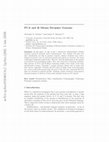
In this paper, we aim to give a tutorial for undergraduate students studying statistical methods ... more In this paper, we aim to give a tutorial for undergraduate students studying statistical methods and/or bioinformatics. The students will learn how data visualization can help in genomic sequence analysis. Students start with a fragment of genetic text of a bacterial genome and analyze its structure. By means of principal component analysis they ``discover'' that the information in the genome is encoded by non-overlapping triplets. Next, they learn how to find gene positions. This exercise on PCA and K-Means clustering enables active study of the basic bioinformatics notions. Appendix 1 contains program listings that go along with this exercise. Appendix 2 includes 2D PCA plots of triplet usage in moving frame for a series of bacterial genomes from GC-poor to GC-rich ones. Animated 3D PCA plots are attached as separate gif files. Topology (cluster structure) and geometry (mutual positions of clusters) of these plots depends clearly on GC-content.
The concept of the limiting step is extended to the asymptotology of multiscale reaction networks... more The concept of the limiting step is extended to the asymptotology of multiscale reaction networks. Complete theory for linear networks with well separated reaction rate constants is developed. We present algorithms for explicit approximations of eigenvalues and eigenvectors of kinetic matrix. Accuracy of estimates is proven. Performance of the algorithms is demonstrated on simple examples. Application of algorithms to nonlinear systems is discussed.
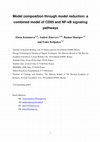
We propose a new approach to model composition, based on reducing several models to the same leve... more We propose a new approach to model composition, based on reducing several models to the same level of complexity and subsequent combining them together. Firstly, we suggest a set of model reduction tools that can be systematically applied to a given model. Secondly, we suggest a notion of a minimal complexity model. This model is the simplest one that can be obtained from the original model using these tools and still able to approximate experimental data. Thirdly, we propose a strategy for composing the reduced models together. Connection with the detailed model is preserved, which can be advantageous in some applications. A toolbox for model reduction and composition has been implemented as part of the BioUML software and tested on the example of integrating two previously published models of the CD95 (APO-1/Fas) signaling pathways. We show that the reduced models lead to the same dynamical behavior of observable species and the same predictions as in the precursor models. The com...
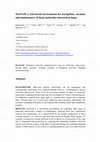
Molecular biology knowledge can be systematically represented in a computer-readable form as a co... more Molecular biology knowledge can be systematically represented in a computer-readable form as a comprehensive map of molecular interactions. There exist a number of maps of molecular interactions containing detailed description of various cell mechanisms. It is difficult to explore these large maps, to comment their content and to maintain them. Though there exist several tools addressing these problems individually, the scientific community still lacks an environment that combines these three capabilities together. NaviCell is a web-based environment for exploiting large maps of molecular interactions, created in CellDesigner, allowing their easy exploration, curation and maintenance. NaviCell combines three features: (1) efficient map browsing based on Google Maps engine; (2) semantic zooming for viewing different levels of details or of abstraction of the map and (3) integrated web-based blog for collecting the community feedback. NaviCell can be easily used by experts in the fiel...
Two blind source separation methods (Independent Component Analysis and Non-negative Matrix Facto... more Two blind source separation methods (Independent Component Analysis and Non-negative Matrix Factorization), developed initially for signal processing in engineering, found recently a number of applications in analysis of large-scale data in molecular biology. In this short review, we present the common idea behind these methods, describe ways of implementing and applying them and point out to the advantages compared to more traditional statistical approaches. We focus more specifically on the analysis of gene expression in cancer. The review is finalized by listing available software implementations for the methods described.
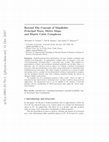
Multidimensional data distributions can have complex topologies and variable local dimensions. To... more Multidimensional data distributions can have complex topologies and variable local dimensions. To approximate complex data, we propose a new type of low-dimensional ``principal object'': a principal cubic complex. This complex is a generalization of linear and non-linear principal manifolds and includes them as a particular case. To construct such an object, we combine a method of topological grammars with the minimization of an elastic energy defined for its embedment into multidimensional data space. The whole complex is presented as a system of nodes and springs and as a product of one-dimensional continua (represented by graphs), and the grammars describe how these continua transform during the process of optimal complex construction. The simplest case of a topological grammar (``add a node'', ``bisect an edge'') is equivalent to the construction of ``principal trees'', an object useful in many practical applications. We demonstrate how it can be ...
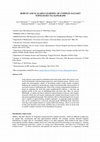
Large datasets represented by multidimensional data point clouds often possess non-trivial distri... more Large datasets represented by multidimensional data point clouds often possess non-trivial distributions with branching trajectories and excluded regions, with the recent single-cell transcriptomic studies of developing embryo being notable examples. Reducing the complexity and producing compact and interpretable representations of such data remains a challenging task. Most of the existing computational methods are based on exploring the local data point neighbourhood relations, a step that can perform poorly in the case of multidimensional and noisy data. Here we present ElPiGraph, a scalable and robust method for approximation of datasets with complex structures which does not require computing the complete data distance matrix or the data point neighbourhood graph. This method is able to withstand high levels of noise and is capable of approximating complex topologies via principal graph ensembles that can be combined into a consensus principal graph. ElPiGraph deals efficiently ...
We present several applications of non-linear data modeling, using principal manifolds and princi... more We present several applications of non-linear data modeling, using principal manifolds and principal graphs constructed using the metaphor of elasticity (elastic principal graph approach). These approaches are generalizations of the Kohonen's self-organizing maps, a class of artificial neural networks. On several examples we show advantages of using non-linear objects for data approximation in comparison to the linear ones. We propose four numerical criteria for comparing linear and non-linear mappings of datasets into the spaces of lower dimension. The examples are taken from comparative political science, from analysis of high-throughput data in molecular biology, from analysis of dynamical systems.
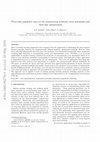
Most of machine learning approaches have stemmed from the application of minimizing the mean squa... more Most of machine learning approaches have stemmed from the application of minimizing the mean squared distance principle, based on the computationally efficient quadratic optimization methods. However, when faced with high-dimensional and noisy data, the quadratic error functionals demonstrated many weaknesses including high sensitivity to contaminating factors and dimensionality curse. Therefore, a lot of recent applications in machine learning exploited properties of non-quadratic error functionals based on L_1 norm or even sub-linear potentials corresponding to quasinorms L_p (0<p<1). The back side of these approaches is increase in computational cost for optimization. Till so far, no approaches have been suggested to deal with arbitrary error functionals, in a flexible and computationally efficient framework. In this paper, we develop a theory and basic universal data approximation algorithms (k-means, principal components, principal manifolds and graphs, regularized and sp...
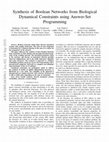
Boolean networks model finite discrete dynamical systems with complex behaviours. The state of ea... more Boolean networks model finite discrete dynamical systems with complex behaviours. The state of each component is determined by a Boolean function of the state of (a subset of) the components of the network. This paper addresses the synthesis of these Boolean functions from constraints on their domain and emerging dynamical properties of the resulting network. The dynamical properties relate to the existence and absence of trajectories between partially observed configurations, and to the stable behaviours (fixpoints and cyclic attractors). The synthesis is expressed as a Boolean satisfiability problem relying on Answer-Set Programming with a parametrized complexity, and leads to a complete non-redundant characterization of the set of solutions. Considered constraints are particularly suited to address the synthesis of models of cellular differentiation processes, as illustrated on a case study. The scalability of the approach is demonstrated on random networks with scale-free struct...
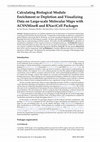
Biological pathways or modules represent sets of interactions or functional relationships occurri... more Biological pathways or modules represent sets of interactions or functional relationships occurring at the molecular level in living cells. A large body of knowledge on pathways is organized in public databases such as the KEGG, Reactome, or in more specialized repositories, the Atlas of Cancer Signaling Network (ACSN) being an example. All these open biological databases facilitate analyses, improving our understanding of cellular systems. We hereby describe ACSNMineR for calculation of enrichment or depletion of lists of genes of interest in biological pathways. ACSNMineR integrates ACSN molecular pathways gene sets, but can use any gene set encoded as a GMT file, for instance sets of genes available in the Molecular Signatures Database (MSigDB). We also present RNaviCell, that can be used in conjunction with ACSNMineR to visualize different data types on web-based, interactive ACSN maps. We illustrate the functionalities of the two packages with biological data taken from large-s...
The most common result of analysis of highthroughput data in molecular biology represents a globa... more The most common result of analysis of highthroughput data in molecular biology represents a global list of genes, ranked accordingly to a certain score. The score can be a measure of differential expression. Recent work proposed a new method for selecting a number of genes in a ranked gene list from microarray gene expression data such that this set forms the Optimally Functionally Enriched Network (OFTEN), formed by known physical interactions between genes or their products. Here we present calculation results of relative connectivity of genes from META-OFTEN network and tentative biological interpretation of the most reproducible signal. The relative connectivity and inbetweenness values of genes from META-OFTEN network were estimated.
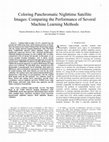
Artificial light-at-night (ALAN), emitted from the ground and visible from space, marks human pre... more Artificial light-at-night (ALAN), emitted from the ground and visible from space, marks human presence on Earth. Since the launch of the Suomi National Polar Partnership satellite with the Visible Infrared Imaging Radiometer Suite Day/Night Band (VIIRS/DNB) onboard, global nighttime images have significantly improved; however, they remained panchromatic. Although multispectral images are also available, they are either commercial or free of charge, but sporadic. In this paper, we use several machine learning techniques, such as linear, kernel, random forest regressions, and elastic map approach, to transform panchromatic VIIRS/DBN into Red Green Blue (RGB) images. To validate the proposed approach, we analyze RGB images for eight urban areas worldwide. We link RGB values, obtained from ISS photographs, to panchromatic ALAN intensities, their pixel-wise differences, and several land-use type proxies. Each dataset is used for model training, while other datasets are used for the model...
Uploads
Papers by Andrei Zinovyev