Papers by Fawzi Nashashibi
IEEE Conference Proceedings, 2016
HAL (Le Centre pour la Communication Scientifique Directe), 2018
Ce document est à remplir par le coordinateur en collaboration avec les partenaires du projet. L'... more Ce document est à remplir par le coordinateur en collaboration avec les partenaires du projet. L'ensemble des partenaires doit avoir une copie de la version transmise à l'ANR. Ce modèle doit être utilisé uniquement pour le compte-rendu de fin de projet.
IEEE Conference Proceedings, 2016
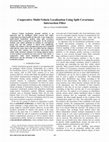
IEEE Intelligent Transportation Systems Magazine, 2013
Vehicle localization (ground vehicles) is an important task for intelligent vehicle systems and v... more Vehicle localization (ground vehicles) is an important task for intelligent vehicle systems and vehicle cooperation may bring benefits for this task. A new cooperative multi-vehicle localization method using split covariance intersection filter is proposed in this paper. In the proposed method, each vehicle maintains an estimate of a decomposed group state and this estimate is shared with neighboring vehicles; the estimate of the decomposed group state is updated with both the sensor data of the ego-vehicle and the estimates sent from other vehicles; the covariance intersection filter which yields consistent estimates even facing unknown degree of inter-estimate correlation has been used for data fusion. A comparative study based simulations demonstrate the effectiveness and the advantage of the proposed cooperative localization method.
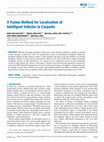
IEEE Access, 2020
With the increasing demand for urban space, more and more multistory carparks are needed as they ... more With the increasing demand for urban space, more and more multistory carparks are needed as they will play a central role in the city transportation system. An autonomous navigation solution for Intelligent Vehicles in these indoor scenarios is then required. One step to solve this problem is to localize Intelligent Vehicles in these specific environments. However, the lack of GPS due to signal obstruction (multipath, non-line of sight, interference, etc.) appears to be a significant concern for any localization system, let alone indoor ones. Hence, in this paper, a wireless sensor network based approach is proposed to replace the GPS (Global Positioning System) role for indoor environments. In addition, a fusion framework for multiple sensors such as Wi-Fi access points, Inertial Measurement Unit (IMU) or LiDAR is studied. Experiments in almost one-year duration yield a stable result of mean global localization error at 0.5m.
Precise positioning plays a key role in successful navigation of autonomous vehicles. A fusion ar... more Precise positioning plays a key role in successful navigation of autonomous vehicles. A fusion architecture of Global Positioning System (GPS) and Laser-SLAM (Simultaneous Localization and Mapping) is widely adopted. While Laser-SLAM is known for its highly accurate localization, GPS is still required to overcome accumulated error and give SLAM a required reference coordinate. However, there are multiple cases where GPS signal quality is too low or not available such as in multi-story parking, tunnel or urban area due to multipath propagation issue etc. This paper proposes an alternative approach for these areas with WiFi Fingerprinting technique to replace GPS. Result obtained from WiFi Fingerprinting will then be fused with Laser-SLAM to maintain the general architecture, allow seamless adaptation of vehicle to the environment.
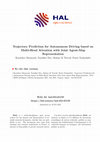
HAL (Le Centre pour la Communication Scientifique Directe), Jun 11, 2021
Predicting the trajectories of surrounding agents is an essential ability for autonomous vehicles... more Predicting the trajectories of surrounding agents is an essential ability for autonomous vehicles navigating through complex traffic scenes. The future trajectories of agents can be inferred using two important cues: the locations and past motion of agents, and the static scene structure. Due to the high variability in scene structure and agent configurations, prior work has employed the attention mechanism, applied separately to the scene and agent configuration to learn the most salient parts of both cues. However, the two cues are tightly linked. The agent configuration can inform what part of the scene is most relevant to prediction. The static scene in turn can help determine the relative influence of agents on each others motion. Moreover, the distribution of future trajectories is multimodal, with modes corresponding to the agent's intent. The agent's intent also informs what part of the scene and agent configuration is relevant to prediction. We thus propose a novel approach applying multi-head attention by considering a joint representation of the static scene and surrounding agents. We use each attention head to generate a distinct future trajectory to address multimodality of future trajectories. Our model achieves state of the art results on the nuScenes prediction benchmark and generates diverse future trajectories compliant with scene structure and agent configuration.
IEEE Conference Proceedings, 2016
IEEE Conference Proceedings, 2018
arXiv (Cornell University), Aug 2, 2018
Convolutional neural networks are designed for dense data, but vision data is often sparse (stere... more Convolutional neural networks are designed for dense data, but vision data is often sparse (stereo depth, point clouds, pen stroke, etc.). We present a method to handle sparse depth data with optional dense RGB, and accomplish depth completion and semantic segmentation changing only the last layer. Our proposal efficiently learns sparse features without the need of an additional validity mask. We show how to ensure network robustness to varying input sparsities. Our method even works with densities as low as 0.8% (8 layer lidar), and outperforms all published stateof-the-art on the Kitti depth completion benchmark.
Proceedings of SPIE, Dec 18, 1998
ABSTRACT
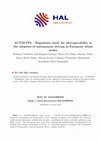
HAL (Le Centre pour la Communication Scientifique Directe), Apr 16, 2018
The technological advances of autonomous and connected road vehicles have been shown an accelerat... more The technological advances of autonomous and connected road vehicles have been shown an accelerating pace in the recent years. On the other hand, the regulations for autonomous, or driverless, road vehicles across Europe still deserve much attention and discussion. In this paper, we introduce the AUTOCITS project which has the main goals of conducting studies on the regulations for the adoption of autonomous cars in Europe, and also to carry out C-ITS Pilots in Madrid, Paris and Lisbon. AUTOCITS aims to contribute, directly or indirectly, to European related policy and reference documents on vehicle automation, regulations, connected and automated driving, and related road infrastructure issues due to the trend towards higher levels of connectivity and automation, where information provided via C-ITS can be truly catalyst for connected and autonomous driving. The project will specially focus on the communication links performance and connectivity between automated vehicles using C-ITS applications connectivity and automation;, in particular, applications increase surrounding environment awareness in relation to infrastructure and ensure both road and driver safety requirements issues and using the regulation framework. AUTOCITS is an innovation project (CEF Program) that aims to facilitate the deployment of autonomous vehicles in urban nodes by developing intelligent transport services based on cooperative systems (C-ITS) that will enable vehicles, users and infrastructures to communicate, exchange, and share information.
IEEE Conference Proceedings, 2016
IEEE Conference Proceedings, 2016
Uploads
Papers by Fawzi Nashashibi