Papers by Hamid Soltanianzadeh
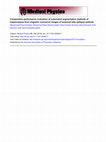
Medical Physics, 2016
Purpose: Segmentation of the hippocampus from magnetic resonance (MR) images is a key task in the... more Purpose: Segmentation of the hippocampus from magnetic resonance (MR) images is a key task in the evaluation of mesial temporal lobe epilepsy (mTLE) patients. Several automated algorithms have been proposed although manual segmentation remains the benchmark. Choosing a reliable algorithm is problematic since structural definition pertaining to multiple edges, missing and fuzzy boundaries, and shape changes varies among mTLE subjects. Lack of statistical references and guidance for quantifying the reliability and reproducibility of automated techniques has further detracted from automated approaches. The purpose of this study was to develop a systematic and statistical approach using a large dataset for the evaluation of automated methods and establish a method that would achieve results better approximating those attained by manual tracing in the epileptogenic hippocampus. Methods: A template database of 195 (81 males, 114 females; age range 32-67 yr, mean 49.16 yr) MR images of mTLE patients was used in this study. Hippocampal segmentation was accomplished manually and by two well-known tools (FreeSurfer and ) and two previously published methods developed at their institution [Automatic brain structure segmentation (ABSS) and Lo-calInfo]. To establish which method was better performing for mTLE cases, several voxel-based, distance-based, and volume-based performance metrics were considered. Statistical validations of the results using automated techniques were compared with the results of benchmark manual segmentation. Extracted metrics were analyzed to find the method that provided a more similar result relative to the benchmark. Results: Among the four automated methods, ABSS generated the most accurate results. For this method, the Dice coefficient was 5.13%, 14.10%, and 16.67% higher, Hausdorff was 22.65%, 86.73%, and 69.58% lower, precision was 4.94%, −4.94%, and 12.35% higher, and the root mean square (RMS) was 19.05%, 61.90%, and 65.08% lower than LocalInfo, FreeSurfer, and , respectively. The Bland-Altman similarity analysis revealed a low bias for the ABSS and LocalInfo techniques compared to the others. Conclusions: The ABSS method for automated hippocampal segmentation outperformed other methods, best approximating what could be achieved by manual tracing. This study also shows that four categories of input data can cause automated segmentation methods to fail. They include incomplete studies, artifact, low signal-to-noise ratio, and inhomogeneity. Different scanner platforms
2009 International Conference on Digital Image Processing, 2009
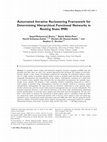
Human Brain Mapping, 2015
To spatially cluster resting state-functional magnetic resonance imaging (rs-fMRI) data into pote... more To spatially cluster resting state-functional magnetic resonance imaging (rs-fMRI) data into potential networks, there are only a few general approaches that determine the number of networks/ clusters, despite a wide variety of techniques proposed for clustering. For individual subjects, extraction of a large number of spatially disjoint clusters results in multiple small networks that are spatiotemporally homogeneous but irreproducible across subjects. Alternatively, extraction of a small number of clusters creates spatially large networks that are temporally heterogeneous but spatially reproducible across subjects. We propose a fully automatic, iterative reclustering framework in which a small number of spatially large, heterogeneous networks are initially extracted to maximize spatial reproducibility. Subsequently, the large networks are iteratively subdivided to create spatially reproducible subnetworks until the overall within-network homogeneity does not increase substantially. The proposed approach discovers a rich network hierarchy in the brain while simultaneously optimizing spatial reproducibility of networks across subjects and individual network homogeneity. We also propose a novel metric to measure the connectivity of brain regions, and in a simulation study show that our connectivity metric and framework perform well in the face of low signal to noise and initial segmentation errors. Experimental results generated using real fMRI data show that the proposed metric improves stability of network clusters across subjects, and generates a meaningful pattern for spatially hierarchical structure of the brain.
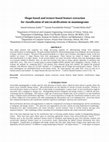
SPIE Proceedings, 2001
This paper presents and compares two image processing methods for differentiating benign from mal... more This paper presents and compares two image processing methods for differentiating benign from malignant microcalcifications in mammograms. The gold standard method for differentiating benign from malignant microcalcifications is biopsy, which is invasive. The goal of the proposed methods is to reduce rate of biopsies with negative results. In the first method, we extract 17 shape features from each mammogram. These features are related to shapes of individual microcalcifications or to their clusters. In the second method, we extract 44 texture features from each mammogram using co-occurrence method of Haralick. Next, we select best features from each set using a genetic algorithm, to maximize area under ROC curve. This curve is created using a k-nearest neighbor (kNN) classifier and a malignancy criterion. Finally, we evaluate the methods by comparing ROC's with greatest areas obtained using each method. We applied the proposed methods, with different values of k in kNN classifier, to 74 malignant and 29 benign microcalcification clusters. Truth for each mammogram was established based on the biopsy results. We found greatest area under ROC curve for each set of features used in each method. For shape features this area was 0.82 (k = 7) and for Haralick features it was 0.72 (k = 9).
Lecture Notes in Computer Science, 2009
2004 2nd IEEE International Symposium on Biomedical Imaging: Macro to Nano (IEEE Cat No. 04EX821)
Medical Imaging 2005: Physiology, Function, and Structure from Medical Images, 2005
Mathematical Biosciences, 2011
This article appeared in a journal published by Elsevier. The attached copy is furnished to the a... more This article appeared in a journal published by Elsevier. The attached copy is furnished to the author for internal non-commercial research and education use, including for instruction at the authors institution and sharing with colleagues. Other uses, including reproduction and distribution, or selling or licensing copies, or posting to personal, institutional or third party websites are prohibited. In most cases authors are permitted to post their version of the article (e.g. in Word or Tex form) to their personal website or institutional repository. Authors requiring further information regarding Elsevier's archiving and manuscript policies are encouraged to visit: http://www.elsevier.com/copyright
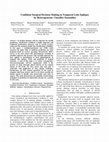
2011 IEEE 11th International Conference on Data Mining Workshops, 2011
In medical domains with low tolerance for invalid predictions, classification confidence is highl... more In medical domains with low tolerance for invalid predictions, classification confidence is highly important and traditional performance measures such as overall accuracy cannot provide adequate insight into classifications reliability. In this paper, a confident-prediction rate (CPR) which measures the upper limit of confident predictions has been proposed based on receiver operating characteristic (ROC) curves. It has been shown that heterogeneous ensemble of classifiers improves this measure. This ensemble approach has been applied to lateralization of focal epileptogenicity in temporal lobe epilepsy (TLE) and prediction of surgical outcomes. A goal of this study is to reduce extraoperative electrocorticography (eECoG) requirement which is the practice of using electrodes placed directly on the exposed surface of the brain. We have shown that such goal is achievable with application of data mining techniques. Furthermore, all TLE surgical operations do not result in complete relief from seizures and it is not always possible for human experts to identify such unsuccessful cases prior to surgery. This study demonstrates the capability of data mining techniques in prediction of undesirable outcome for a portion of such cases.
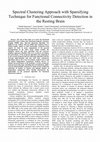
The aim of this study is to assess the functional connectivity from resting state functional magn... more The aim of this study is to assess the functional connectivity from resting state functional magnetic resonance imaging (fMRI) data. Spectral clustering algorithm was applied to the realistic and real fMRI data acquired from a resting healthy subject to find functionally connected brain regions. In order to make computation of the spectral decompositions of the entire brain volume feasible, the similarity matrix has been sparsified with the t-nearestneighbor approach. Realistic data were created to investigate the performance of the proposed algorithm and comparing it to the recently proposed spectral clustering algorithm with the Nystrom approximation and also with some well-known algorithms such as the Cross Correlation Analysis (CCA) and the spatial Independent Component Analysis (sICA). To enhance the performance of the methods, a variety of data pre and post processing steps, including data normalization, outlier removal, dimensionality reduction by using wavelet coefficients, estimation of number of clusters and optimal number of independent components (ICs). Results demonstrate the applicability of the proposed algorithm for functional connectivity analysis.
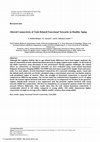
2013 20th Iranian Conference on Biomedical Engineering (ICBME), 2013
Although the cognitive deficits due to age-related brain differences have been largely analyzed, ... more Although the cognitive deficits due to age-related brain differences have been largely analyzed, the altered connectivity of task related functional networks in aging requires more studies. As the brain of old adults experience some alterations in task performance during cognitive challenges, the related effects on connectivity of functional networks are here evaluated using event-related functional Magnetic Resonance Imaging (fMRI). The fMRI data have been acquired for simple visual and motor tasks. For each subject, several Functional Connectivity (FC) networks including, motor, visual and the default mode networks are firstly calculated using a conventional voxel-wise correlation analysis with predefined region of interest. Then, the strength of functional connectivity is assessed and compared for different age groups. The current study has evaluated three hypotheses on FC of aging brain: the frontal regions involved with motor network try to compensate for declines in the posterior regions, default-mode network is less suppressed and, the posterior regions involved with visual network exhibit less connectivity. The first two hypotheses are accepted by analysis results but visual network behaves differently. Also, results show that the task related functional connectivity is considerably altered in old adults compared to young adults. Old adults demonstrate higher connectivity strength on average with a slightly smaller variance than young adults.
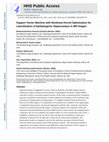
Conference proceedings : ... Annual International Conference of the IEEE Engineering in Medicine and Biology Society. IEEE Engineering in Medicine and Biology Society. Annual Conference, 2014
Surgical treatment is suggested for seizure control in medically intractable epilepsy patients. D... more Surgical treatment is suggested for seizure control in medically intractable epilepsy patients. Detailed pre-surgical evaluation and lateralization using Magnetic Resonance Images (MRI) is expected to result in a successful surgical outcome. In this study, an optimized pattern recognition approach is proposed for lateralization of mesial Temporal Lobe Epilepsy (mTLE) patients using asymmetry of imaging indices of hippocampus. T1-weighted and Fluid-Attenuated Inversion Recovery (FLAIR) images of 76 symptomatic mTLE patients are considered. First, hippocampus is segmented using automatic and manual segmentation methods; then, volumetric and intensity features are extracted from the MR images. A nonlinear Support Vector Machine (SVM) with optimized Gaussian Radial Basis Function (GRBF) kernel is used to classify the imaging features. Using leave-one-out cross validation, this method results in a correct lateralization rate of 82%, a probability of detection for the left side of 0.90 (w...
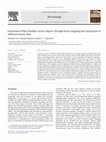
NeuroImage, 2011
This paper presents a visualization and analysis framework for evaluating changes in structural o... more This paper presents a visualization and analysis framework for evaluating changes in structural organization of fiber bundles in human brain white matter. Statistical analysis of fiber bundle organization is conducted using an anisotropy measure, volume ratio (VR), which is ratio of anisotropic and isotropic components. Initially fiber bundles are tracked using a probabilistic algorithm starting from seed voxels. To ensure accurate selection of seed voxels and to prevent operator bias, a reference brain (MNI_152) is used when marking ROIs. Individual structural MRI brain scans are mapped to the reference using volumetric conformal parameterization. This mapping preserves topology and aligns features perfectly making it a robust and accurate registration technique. One-to-one mapping to the template allows ROI selection and subsequent transfer of ROI to structural MRI of subject. Affine registration coregisters structural MRI and DTI. Seed voxels are mapped to DTI using the resulting transformation parameters. To evaluate the proposed approach, MRI and DTI of 12 normal volunteers and 15 medial temporal lobe epilepsy patients are used. First, a statistical hypothesis testing is conducted to test for anisotropy changes in cingulum and fornix fiber bundles of epileptic patients. Experimental results reveal a 40% decrease in anisotropy levels of cingulum in patients compared to volunteers. They also show a 25% overall decrease in anisotropy of fornix. Secondly, shapes of the bundles are visualized in 3D illustrating that the bundles of epileptic patients are bumpy while those of normal volunteers are smooth.
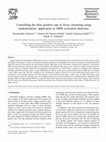
Magnetic Resonance Imaging, 2004
Despite its potential advantages for fMRI analysis, fuzzy C-means (FCM) clustering suffers from l... more Despite its potential advantages for fMRI analysis, fuzzy C-means (FCM) clustering suffers from limitations such as the need for a priori knowledge of the number of clusters, and unknown statistical significance and instability of the results. We propose a randomization-based method to control the false-positive rate and estimate statistical significance of the FCM results. Using this novel approach, we develop an fMRI activation detection method. The ability of the method in controlling the false-positive rate is shown by analysis of false positives in activation maps of resting-state fMRI data. Controlling the false-positive rate in FCM allows comparison of different fuzzy clustering methods, using different feature spaces, to other fMRI detection methods. In this article, using simulation and real fMRI data, we compare a novel feature space that takes the variability of the hemodynamic response function into account (HRF-based feature space) to the conventional cross-correlation analysis and FCM using the cross-correlation feature space. In both cases, the HRF-based feature space provides a greater sensitivity compared to the cross-correlation feature space and conventional cross-correlation analysis. Application of the proposed method to finger-tapping fMRI data, using HRF-based feature space, detected activation in sub-cortical regions, whereas both of the FCM with cross-correlation feature space and the conventional cross-correlation method failed to detect them.
IEEE Transactions on Medical Imaging, 2003
IEEE Transactions on Medical Imaging, 2003
Uploads
Papers by Hamid Soltanianzadeh