Papers by Harini Veeraraghavan

arXiv (Cornell University), May 27, 2020
We implemented and evaluated a multiple resolution residual network (MRRN) for multiple normal or... more We implemented and evaluated a multiple resolution residual network (MRRN) for multiple normal organs-at-risk (OAR) segmentation from computed tomography (CT) images for thoracic radiotherapy treatment (RT) planning. Our approach simultaneously combines feature streams computed at multiple image resolutions and feature levels through residual connections. The feature streams at each level are updated as the images are passed through various feature levels. We trained our approach using 206 thoracic CT scans of lung cancer patients with 35 scans held out for validation to segment the left and right lungs, heart, esophagus, and spinal cord. This approach was tested on 60 CT scans from the open-source AAPM Thoracic Auto-Segmentation Challenge dataset. Performance was measured using the Dice Similarity Coefficient (DSC). Our approach outperformed the best-performing method in the grand challenge for hard-to-segment structures like the esophagus and achieved comparable results for all other structures. Median DSC using our method was 0.97 (interquartile range [IQR]: 0.97-0.98) for the left and right lungs, 0.93 (IQR: 0.93-0.95) for the heart, 0.78 (IQR: 0.76-0.80) for the esophagus, and 0.88 (IQR: 0.86-0.89) for the spinal cord.

IEEE Transactions on Medical Imaging
Accurate and robust segmentation of lung cancers from CT, even those located close to mediastinum... more Accurate and robust segmentation of lung cancers from CT, even those located close to mediastinum, is needed to more accurately plan and deliver radiotherapy and to measure treatment response. Therefore, we developed a new cross-modality educed distillation (CMEDL) approach, using unpaired CT and MRI scans, whereby an informative teacher MRI network guides a student CT network to extract features that signal the difference between foreground and background. Our contribution eliminates two requirements of distillation methods: (i) paired image sets by using an image to image (I2I) translation and (ii) pre-training of the teacher network with a large training set by using concurrent training of all networks. Our framework uses an end-to-end trained unpaired I2I translation, teacher, and student segmentation networks. Architectural flexibility of our framework is demonstrated using 3 segmentation and 2 I2I networks. Networks were trained with 377 CT and 82 T2w MRI from different sets of patients, with independent validation (N = 209 tumors) and testing (N = 609 tumors) datasets. Network design, methods to combine MRI with CT information, distillation learning under informative (MRI to CT), weak (CT to MRI) and equal teacher (MRI to MRI), and ablation tests were performed. Accuracy was measured using Dice similarity (DSC), surface Dice (sDSC), and Hausdorff distance at the 95 th percentile (HD95). The CMEDL approach was significantly (p < 0.001) more accurate (DSC of 0.77 vs. 0.73) than non-CMEDL methods with an informative teacher for CT lung tumor, with a weak teacher (DSC of 0.84 vs. 0.81) for MRI lung tumor, and with equal teacher (DSC of 0.90 vs. 0.88) for MRI multi-organ segmentation. CMEDL also reduced inter-rater lung tumor segmentation variabilities.
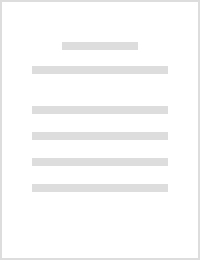
Physics and Imaging in Radiation Oncology, 2022
Background and purpose Stereotactic body radiation therapy (SBRT) of locally advanced pancreatic ... more Background and purpose Stereotactic body radiation therapy (SBRT) of locally advanced pancreatic cancer (LAPC) is challenging due to significant motion of gastrointestinal (GI) organs. The goal of our study was to quantify inter and intrafraction deformations and dose accumulation of upper GI organs in LAPC patients. Materials and methods Five LAPC patients undergoing five-fraction magnetic resonance-guided radiation therapy (MRgRT) using abdominal compression and daily online plan adaptation to 50 Gy were analyzed. A pre-treatment, verification, and post-treatment MR imaging (MRI) for each of the five fractions (75 total) were used to calculate intra and interfraction motion. The MRIs were registered using Large Deformation Diffeomorphic Metric Mapping (LDDMM) deformable image registration (DIR) method and total dose delivered to stomach_duodenum, small bowel (SB) and large bowel (LB) were accumulated. Deformations were quantified using gradient magnitude and Jacobian integral of the Deformation Vector Fields (DVF). Registration DVFs were geometrically assessed using Dice and 95th percentile Hausdorff distance (HD95) between the deformed and physician’s contours. Accumulated doses were then calculated from the DVFs. Results Median Dice and HD95 were: Stomach_duodenum (0.9, 1.0 mm), SB (0.9, 3.6 mm), and LB (0.9, 2.0 mm). Median (max) interfraction deformation for stomach_duodenum, SB and LB was 6.4 (25.8) mm, 7.9 (40.5) mm and 7.6 (35.9) mm. Median intrafraction deformation was 5.5 (22.6) mm, 8.2 (37.8) mm and 7.2 (26.5) mm. Accumulated doses for two patients exceeded institutional constraints for stomach_duodenum, one of whom experienced Grade1 acute and late abdominal toxicity. Conclusion LDDMM method indicates feasibility to measure large GI motion and accumulate dose. Further validation on larger cohort will allow quantitative dose accumulation to more reliably optimize online MRgRT.

Neuro-Oncology, 2017
of adult patients in whom pretreatment CT images and subsequent WHO 2016 diagnoses of infiltratin... more of adult patients in whom pretreatment CT images and subsequent WHO 2016 diagnoses of infiltrating glioma were available. Presence and pattern of tumor calcification were determined by consensus. RESULTS: A total of 492 patients met inclusion criteria. Median age was 59 years and 61.8% were male. Tumor types included glioblastoma IDH wild-type (IDHwt) in 272/492 patients (55%), glioblastoma IDH mutant (IDHmut) in 18/492 (4%), WHO grade II/III astrocytoma IDHwt (71/492; 14%), WHO grade II/III astrocytoma IDHmut (56/492; 11%), and WHO grade II/III oligodendroglioma (69/492; 14%). The remaining six tumors (1%) could not be full characterized. Tumor calcification was present in 47/492 patients (10%). The majority of patients with calcification (27/47; 57%) were diagnosed with WHO grade II or III oligodendroglioma, followed by glioblastoma IDHwt (8/47, 17%). The presence of calcification was significantly associated with IDH mutation and 1p/19q codeletion (each p<0.001). A pattern of calcification we termed "classic varicoid" was observed in 18 patients (16 oligodendroglioma, one IDHmut GBM, and one IDHmut astrocytoma). Only 14/343 (4%) of patients with IDHwt WHO grade II/III astrocytoma or IDHwt glioblastoma demonstrated calcification and none showed the "classic varicoid" pattern. DISCUSSION: Tumor calcification at CT strongly predicts oligodendroglial pathology, despite the far greater frequency of astrocytoma/glioblastoma. The "classic varicoid" pattern of calcification is highly specific for IDH mutant gliomas.

Medical Image Computing and Computer Assisted Intervention – MICCAI 2018, 2018
We present an adversarial domain adaptation based deep learning approach for automatic tumor segm... more We present an adversarial domain adaptation based deep learning approach for automatic tumor segmentation from T2-weighted MRI. Our approach is composed of two steps: (i) a tumor-aware unsupervised cross-domain adaptation (CT to MRI), followed by (ii) semi-supervised tumor segmentation using Unet trained with synthesized and limited number of original MRIs. We introduced a novel target specific loss, called tumor-aware loss, for unsupervised cross-domain adaptation that helps to preserve tumors on synthesized MRIs produced from CT images. In comparison, state-of-the art adversarial networks trained without our tumor-aware loss produced MRIs with ill-preserved or missing tumors. All networks were trained using labeled CT images from 377 patients with non-small cell lung cancer obtained from the Cancer Imaging Archive and unlabeled T2w MRIs from a completely unrelated cohort of 6 patients with pre-treatment and 36 on-treatment scans. Next, we combined 6 labeled pre-treatment MRI scans with the synthesized MRIs to boost tumor segmentation accuracy through semi-supervised learning. Semi-supervised training of cycle-GAN produced a segmentation accuracy of 0.66 computed using Dice Score Coefficient (DSC). Our method trained with only synthesized MRIs produced an accuracy of 0.74 while the same method trained in semi-supervised setting produced the best accuracy of 0.80 on test. Our results show that tumor-aware adversarial domain adaptation helps to achieve reasonably accurate cancer segmentation from limited MRI data by leveraging large CT datasets.
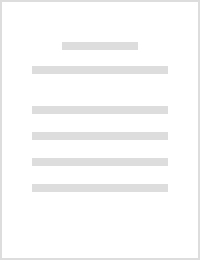
Physics and Imaging in Radiation Oncology, 2021
Background and Purpose Reducing trismus in radiotherapy for head and neck cancer (HNC) is importa... more Background and Purpose Reducing trismus in radiotherapy for head and neck cancer (HNC) is important. Automated deep learning (DL) segmentation and automated planning was used to introduce new and rarely segmented masticatory structures to study if trismus risk could be decreased. Materials and Methods Auto-segmentation was based on purpose-built DL, and automated planning used our in-house system, ECHO. Treatment plans for ten HNC patients, treated with 2 Gy × 35 fractions, were optimized (ECHO0). Six manually segmented OARs were replaced with DL auto-segmentations and the plans re-optimized (ECHO1). In a third set of plans, mean doses for auto-segmented ipsilateral masseter and medial pterygoid (MIMean, MPIMean), derived from a trismus risk model, were implemented as dose-volume objectives (ECHO2). Clinical dose-volume criteria were compared between the two scenarios (ECHO0vs. ECHO1; ECHO1vs. ECHO2; Wilcoxon signed-rank test; significance: p < 0.01). Results Small systematic differences were observed between the doses to the six auto-segmented OARs and their manual counterparts (median: ECHO1 = 6.2 (range: 0.4, 21) Gy vs. ECHO0 = 6.6 (range: 0.3, 22) Gy; p = 0.007), and the ECHO1 plans provided improved normal tissue sparing across a larger dose-volume range. Only in the ECHO2 plans, all patients fulfilled both MIMean and MPIMean criteria. The population median MIMean and MPIMean were considerably lower than those suggested by the trismus model (ECHO0: MIMean = 13 Gy vs. ≤42 Gy; MPIMean = 29 Gy vs. ≤68 Gy). Conclusions Automated treatment planning can efficiently incorporate new structures from DL auto-segmentation, which results in trismus risk sparing without deteriorating treatment plan quality. Auto-planning and deep learning auto-segmentation together provide a powerful platform to further improve treatment planning.

JCO Precision Oncology, 2019
PURPOSE To determine if radiomic measures of tumor heterogeneity derived from baseline contrast-e... more PURPOSE To determine if radiomic measures of tumor heterogeneity derived from baseline contrast-enhanced computed tomography (CE-CT) are associated with durable clinical benefit and time to off-treatment in patients with recurrent ovarian cancer (OC) enrolled in prospective immunotherapeutic trials. MATERIALS AND METHODS This retrospective study included 75 patients with recurrent OC who were enrolled in prospective immunotherapeutic trials (n = 74) or treated off-label (n = 1) and had baseline CE-CT scans. Disease burden (total tumor volume, number of disease sites), radiomic measures of intertumor heterogeneity (cluster-site entropy, cluster-site dissimilarity), and intratumor heterogeneity of the largest lesion (Haralick texture features) were computed. Associations of clinical, conventional imaging, and radiomic measures with durable clinical benefit and time to off-treatment were examined. RESULTS In univariable analysis, fewer disease sites, lower intertumor heterogeneity (low...

Medical Physics, 2019
Accurate tumor segmentation is a requirement for magnetic resonance (MR)-based radiotherapy. Lack... more Accurate tumor segmentation is a requirement for magnetic resonance (MR)-based radiotherapy. Lack of large expert annotated MR datasets makes training deep learning models difficult. Therefore, a cross-modality (MR-CT) deep learning segmentation approach that augments training data using pseudo MR images produced by transforming expert-segmented CT images was developed. Methods: Eighty-One T2-weighted MRI scans from 28 patients with non-small cell lung cancers (9 with pre-treatment and weekly MRI and the remainder with pre-treatment MRI scans) were analyzed. Cross-modality prior encoding the transformation of CT to pseudo MR images resembling T2w MRI was learned as a generative adversarial deep learning model. This model augmented training data arising from 6 expert-segmented T2w MR patient scans with 377 pseudo MRI from non-small cell lung cancer CT patient scans with obtained from the Cancer Imaging Archive. A two-dimensional Unet implemented with batch normalization was trained to segment the tumors from T2w MRI. This method was benchmarked against (a) standard data augmentation and two state-of-the art cross-modality pseudo MR-based augmentation and (b) two segmentation networks. Segmentation accuracy was computed using Dice similarity coefficient (DSC), Hausdroff distance metrics, and volume ratio. The proposed approach produced the lowest statistical variability in the intensity distribution between pseudo and T2w MR images measured as Kullback-Leibler divergence of 0.069. This method produced the highest segmentation accuracy with a DSC of (0.75 ± 0.12) and the lowest Hausdroff distance of (9.36 mm ± 6.00mm) on the test dataset. This approach produced highly similar estimations of tumor growth as an expert (P = 0.37). A novel deep learning MR segmentation was developed that overcomes the limitation of learning robust models from small datasets by leveraging learned cross-modality priors to augment training. The results show the feasibility of the approach and the corresponding improvement over the state-of-the-art methods.

Abdominal Radiology, 2018
Purpose To assess the associations between inter-site texture heterogeneity parameters derived fr... more Purpose To assess the associations between inter-site texture heterogeneity parameters derived from computed tomography (CT), survival, and BRCA mutation status in women with high-grade serous ovarian cancer (HGSOC). Materials and methods Retrospective study of 88 HGSOC patients undergoing CT and BRCA mutation status testing prior to primary cytoreductive surgery. Associations between texture metrics-namely inter-site cluster variance (SCV), intersite cluster prominence (SCP), inter-site cluster entropy (SE)-and overall survival (OS), progression-free survival (PFS) as well as BRCA mutation status were assessed. Results Higher inter-site cluster variance (SCV) was associated with lower PFS (p = 0.006) and OS (p = 0.003). Higher inter-site cluster prominence (SCP) was associated with lower PFS (p = 0.02) and higher inter-site cluster entropy (SE) correlated with lower OS (p = 0.01). Higher values of all three metrics were significantly associated with lower complete surgical resection status in BRCA-negative patients (SE p = 0.039, SCV p = 0.006, SCP p = 0.02), but not in BRCApositive patients (SE p = 0.7, SCV p = 0.91, SCP p = 0.67). None of the metrics were able to distinguish between BRCA mutation carrier and non-mutation carrier. Conclusion The assessment of tumoral heterogeneity in the era of personalized medicine is important, as increased heterogeneity has been associated with distinct genomic abnormalities and worse patient outcomes. A radiomics approach using standard-of-care CT scans might have a clinical impact by offering a non-invasive tool to predict outcome and therefore improving treatment effectiveness. However, it was not able to assess BRCA mutation status in women with HGSOC.

Medical physics, Jan 24, 2018
This report presents the methods and results of the Thoracic Auto-Segmentation Challenge organize... more This report presents the methods and results of the Thoracic Auto-Segmentation Challenge organized at the 2017 Annual Meeting of American Association of Physicists in Medicine. The purpose of the challenge was to provide a benchmark dataset and platform for evaluating performance of auto-segmentation methods of organs at risk (OARs) in thoracic CT images. Sixty thoracic CT scans provided by three different institutions were separated into 36 training, 12 offline testing, and 12 online testing scans. Eleven participants completed the offline challenge, and seven completed the online challenge. The OARs were left and right lungs, heart, esophagus and spinal cord. Clinical contours used for treatment planning were quality checked and edited to adhere to the RTOG 1106 contouring guidelines. Algorithms were evaluated using the Dice coefficient, Hausdorff distance, and mean surface distance. A consolidated score was computed by normalizing the metrics against inter-rater variability and a...

Medical physics, Jan 26, 2017
The growing use of magnetic resonance imaging (MRI) as a substitute for computed tomography-based... more The growing use of magnetic resonance imaging (MRI) as a substitute for computed tomography-based treatment planning requires the development of effective algorithms to generate electron density maps for treatment planning and patient setup verification. The purpose of this work was to develop a method to synthesize computerized tomography (CT) for MR-only radiotherapy of head and neck cancer patients. The algorithm is based on registration of multiple patient datasets containing both MRI and CT images (a "multiatlas" algorithm). Twelve matched pairs of good quality CT and MRI scans (those without apparent motion and blurring artifacts) were selected from a pool of head and neck cancer patients to form the atlas. All atlas MRI scans were preprocessed to reduce scanner- and patient-induced intensity inhomogeneities and to standardize their intensity histograms. Atlas CT and MRIs were coregistered using a novel bone-to-air replacement technique applied to the CT scans that i...

European radiology, Jan 5, 2016
To investigate whether qualitative magnetic resonance (MR) features can distinguish leiomyosarcom... more To investigate whether qualitative magnetic resonance (MR) features can distinguish leiomyosarcoma (LMS) from atypical leiomyoma (ALM) and assess the feasibility of texture analysis (TA). This retrospective study included 41 women (ALM = 22, LMS = 19) imaged with MRI prior to surgery. Two readers (R1, R2) evaluated each lesion for qualitative MR features. Associations between MR features and LMS were evaluated with Fisher's exact test. Accuracy measures were calculated for the four most significant features. TA was performed for 24 patients (ALM = 14, LMS = 10) with uniform imaging following lesion segmentation on axial T2-weighted images. Texture features were pre-selected using Wilcoxon signed-rank test with Bonferroni correction and analyzed with unsupervised clustering to separate LMS from ALM. Four qualitative MR features most strongly associated with LMS were nodular borders, haemorrhage, "T2 dark" area(s), and central unenhanced area(s) (p ≤ 0.0001 each feature/...
IEEE International Conference on Robotics and Automation, 2004. Proceedings. ICRA '04. 2004, 2004
This paper presents a camera-based system for tracking vehicles in outdoor scenes such as traffic... more This paper presents a camera-based system for tracking vehicles in outdoor scenes such as traffic intersections. Two different tracking systems, namely, a blob tracker and a Mean Shift tracker provide the position of each target. These results are then fused sequentially using an Extended Kalman filter. The tracking reliability of the blob tracker is improved by using oriented bounding boxes (which provide a much tighter fit than axis aligned boxes) to represent the blobs and a Joint Probabilistic Data Association filter for dealing with data association ambiguity. The Mean Shift tracker is as proposed by Comaniciu et al. [3]. We show that the above tracking formulation can provide reasonable tracking despite the stop-and-go motion of vehicles and clutter in traffic intersections.
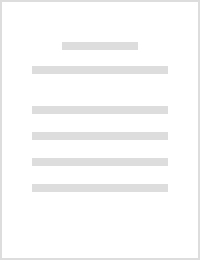
Proceedings. The IEEE 5th International Conference on Intelligent Transportation Systems
ABSTRACT The goal of this project is to develop a passive vision-based sensing system. The system... more ABSTRACT The goal of this project is to develop a passive vision-based sensing system. The system will be capable of monitoring an intersection by observing the vehicle and pedestrian flow, and predicting situations that might give rise to accidents. A single camera looking at an intersection from an arbitrary position is used. However, for extended applications, multiple cameras will be needed. Some of the key elements are camera calibration, motion tracking, vehicle classification, and situations giving rise to collisions. In this paper, we focus on motion tracking. Motion segmentation is performed using an adaptive background model that models each pixel as a mixture of Gaussians. The method used is similar to the method of Stauffer et al. for motion segmentation. Tracking of objects is performed by computing the overlap between oriented bounding boxes. The oriented boxes are computed by vector quantization of the blobs in the scene. The principal angles computed during vector quantization along with other cues of the object are used for classification of detected entities into vehicles and pedestrians.

Journal of Magnetic Resonance Imaging, 2016
Purpose-To use features extracted from magnetic resonance (MR) images and a machinelearning metho... more Purpose-To use features extracted from magnetic resonance (MR) images and a machinelearning method to assist in differentiating breast cancer molecular subtypes. Act (HIPAA)-compliant study received Institutional Review Board (IRB) approval. We identified 178 breast cancer patients between 2006-2011 with: 1) ERPR + (n = 95, 53.4%), ERPR-/HER2 + (n = 35, 19.6%), or triple negative (TN, n = 48, 27.0%) invasive ductal carcinoma (IDC), and 2) preoperative breast MRI at 1.5T or 3.0T. Shape, texture, and histogram-based features were extracted from each tumor contoured on pre-and three postcontrast MR images using in-house software. Clinical and pathologic features were also collected. Machine-learning-based (support vector machines) models were used to identify significant imaging features and to build models that predict IDC subtype. Leave-one-out cross-validation (LOOCV) was used to avoid model overfitting. Statistical significance was determined using the Kruskal-Wallis test. Results-Each support vector machine fit in the LOOCV process generated a model with varying features. Eleven out of the top 20 ranked features were significantly different between IDC subtypes with P < 0.05. When the top nine pathologic and imaging features were incorporated, the predictive model distinguished IDC subtypes with an overall accuracy on LOOCV of 83.4%. The combined pathologic and imaging model's accuracy for each subtype was 89.2% (ERPR+), 63.6% (ERPR-/HER2+), and 82.5% (TN). When only the top nine imaging features were incorporated, the predictive model distinguished IDC subtypes with an overall accuracy on LOOCV of 71.2%. The combined pathologic and imaging model's accuracy for each subtype was 69.9% (ERPR+), 62.9% (ERPR-/HER2+), and 81.0% (TN). We developed a machine-learning-based predictive model using features extracted from MRI that can distinguish IDC subtypes with significant predictive power.
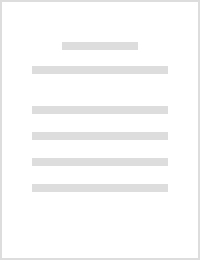
Medical Physics, 2015
ABSTRACT To present new tools for visualizing scans across multiple timepoints alongwith changes ... more ABSTRACT To present new tools for visualizing scans across multiple timepoints alongwith changes in critical metrics. MR scans for a Lung cancer patient were acquired at four different timepoints. A display consisting of 2×2 panels was developed to show the four scans in Transverse, Sagittal or Coronal cut-planes. In order to make sure the user is looking at the same scan-section at different timepoints, two kinds of registration modes were built in: (1) Anatomical registration: Scans at different time points are rigidly registered to the first timepoint. Here, regular anatomical structures like bones are used to drive the registration. (2) Matching tumor mid-plane plane: In this mode the mid-planes of the tumors are matched to register the scans. This mode is useful to users who are interested in looking at the tumor shrinkage/growth longitudinally. Once image registration is performed according to the selected mode, the user can scroll across scan slices together at different timepoints. It is possible to make fine adjustments to the image registration by nudging a particular timepoint by few slices. It is also possible to evaluate the accuracy of registration by using the spotlight tool that highlights the region selected on one of the timepoints, on all the timepoints. Linear interpolation from the neighboring scan slices is used to display a cut-plane located in between slices. The visualization is further improved using the sinc upsampling filter. Once the scans at different timepoints are registered, it is possible to extract metrics for the structures of interest. Currently, statistical features and the first and second order texture features could be extracted at different timepoints. The new features and updates are built into CERR. The tool developed would help visualize scans taken longitudinally along with providing insight into how the critical metrics change over time.
Medical Physics, 2015
ABSTRACT Purpose: Recently, radiomics has emerged as a new research field with the aim of (1) ide... more ABSTRACT Purpose: Recently, radiomics has emerged as a new research field with the aim of (1) identifying quantitative medical image features associated with outcomes, (2) building predictive models of outcomes, (3) and thereby better understanding the underlying mechanisms of outcomes. Our group has developed methods to extract quantitative features from medical images and to model the outcomes. In this work, we summarize our studies with magnetic resonance (MR) images, demonstrating the potential of using radiomics for outcomes research.
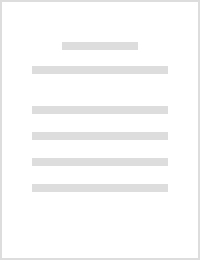
Medical Physics, 2015
ABSTRACT To present an open source and free platform to facilitate radiomics research - The &... more ABSTRACT To present an open source and free platform to facilitate radiomics research - The &quot;Radiomics toolbox&quot; in CERR. There is scarcity of open source tools that support end-to-end modeling of image features to predict patient outcomes. The &quot;Radiomics toolbox&quot; strives to fill the need for such a software platform. The platform supports (1) import of various kinds of image modalities like CT, PET, MR, SPECT, US. (2) Contouring tools to delineate structures of interest. (3) Extraction and storage of image based features like 1st order statistics, gray-scale co-occurrence and zonesize matrix based texture features and shape features and (4) Statistical Analysis. Statistical analysis of the extracted features is supported with basic functionality that includes univariate correlations, Kaplan-Meir curves and advanced functionality that includes feature reduction and multivariate modeling. The graphical user interface and the data management are performed with Matlab for the ease of development and readability of code and features for wide audience. Open-source software developed with other programming languages is integrated to enhance various components of this toolbox. For example: Java-based DCM4CHE for import of DICOM, R for statistical analysis. The Radiomics toolbox will be distributed as an open source, GNU copyrighted software. The toolbox was prototyped for modeling Oropharyngeal PET dataset at MSKCC. The analysis will be presented in a separate paper. The Radiomics Toolbox provides an extensible platform for extracting and modeling image features. To emphasize new uses of CERR for radiomics and image-based research, we have changed the name from the &quot;Computational Environment for Radiotherapy Research&quot; to the &quot;Computational Environment for Radiological Research&quot;.
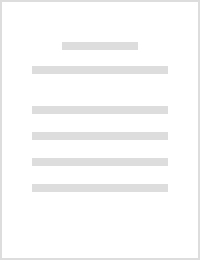
Medical physics, 2015
Development of technical methods to optimally classify tumors via magnetic resonance imaging. The... more Development of technical methods to optimally classify tumors via magnetic resonance imaging. The methods were developed and tested on unusual appearing leiomyomas (ULM) from leiomyosarcomas (LMS). We developed a fully automated method for distinguishing between ULM and LMS from T2-w MR images. Data consisted of 39 patients with histologically proven ULM(=22) and LMS(=17) who underwent preoperative ≥1.5 MRI. 13 MR images were obtained at our institution and rest from elsewhere. Our method consists of several steps. First, all the images were histogram matched, following which the manually segmented tumors were refined through automatic volumetric image segmentation. Next, several image features consisting of 5 Haralick textures, 4 Gabor edges at (0°,45°,90°,135°) and bandwidth 1.414 and Haralick textures computed on each Gabor image resulting in 25 different features was computed from inside the segmented tumors. The features were preweighted by their relevance determined using a pa...

Medical Physics, 2015
Purpose: Apparent diffusion coefficient (ADC), derived from diffusion-weighted magnetic resonance... more Purpose: Apparent diffusion coefficient (ADC), derived from diffusion-weighted magnetic resonance images (DW-MRI), measures the motion of water molecules in vivo and can be used to quantify tumor response to therapy. The accurate measurement of ADC can be adversely affected by organ motion and imaging artifacts. In this paper, the authors' goal was to develop an automated method for reducing artifacts and thereby improve the accuracy of ADC measurements in moving organs such as liver. Methods: The authors developed a novel method of computing ADC with fewer artifacts, through simultaneous image segmentation and iterative registration (SSIR) of multiple b-value DW-MRI. The authors' approach reduces artifacts by automatically finding the best possible alignment between the individual b-value images and a reference DW image using a sequence of transformations. It selects such a sequence by an iterative choice of b-value DW images based on the accuracy of their alignment with the reference DW image. The authors' approach quantifies the accuracy of alignment between a pair of images using modified Hausdroff distance computed between the structures of interest. The structures of interest are identified by a user through strokes drawn in one or more slices in the reference DW image, which are then volumetrically segmented using GrowCut. The same structures are segmented in the remaining b-value images by transforming the user-drawn strokes through registration. The ADC values are computed from all the aligned b-value images. The images are aligned by using affine registration followed by deformable B-spline registration with cubic B-spline resampling. Results: The authors compared the results of ADC computed using their approach with ADC computed (a) without registration and (b) with basic affine registration of all b-value images to a chosen reference. The authors' approach was the most effective in reducing artifacts compared to the other two methods. It resulted in a mean artifact ratio (fraction of voxels in a structure with negative ADC over total number of voxels in the structure) of 2.7% versus 5.4% for affine registration and 32% for no registration for >200 tumors. The authors' approach also resulted in the lowest median standard deviation in the computed mean ADC for all tumors [0.05,0.09,0.07,0.58] compared to those from affine image registration [0.02, 0.14, 0.58, 0.79] and no image registration [0.64, 0.83, 0.83, 1.09] on tests where random displacement [8,10,12,16] pixels were introduced in multiple trials in the b-value images. Conclusions: The authors developed a novel approach for reducing artifacts in ADC maps through simultaneous registration and segmentation of multiple b-value DW images. The authors' method explicitly employs a registration quality metric to align images. When compared to basic affine and no image registrations, the authors' approach produces registrations of greater accuracy with lowest artifact ratio and median standard deviation of the computed mean ADC values for a wide range of displacements.
Uploads
Papers by Harini Veeraraghavan