Papers by MUHAMMAD SUKRI BIN RAMLI
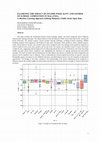
This study examines the relationship between income inequality, gender, and school completion rat... more This study examines the relationship between income inequality, gender, and school completion rates in Malaysia using machine learning techniques. The dataset utilized is from the Malaysia's Public Sector Open Data Portal, covering the period 2016-2022. The analysis employs various machine learning techniques, including K-means clustering, ARIMA modeling, Random Forest regression, and Prophet for time series forecasting. These models are used to identify patterns, trends, and anomalies in the data, and to predict future school completion rates. Key findings reveal significant disparities in school completion rates across states, genders, and income levels. The analysis also identifies clusters of states with similar completion rates, suggesting potential regional factors influencing educational outcomes. Furthermore, time series forecasting models accurately predict future completion rates, highlighting the importance of ongoing monitoring and intervention strategies. The study concludes with recommendations for policymakers and educators to address the observed disparities and improve school completion rates in Malaysia. These recommendations include targeted interventions for specific states and demographic groups, investment in early childhood education, and addressing the impact of income inequality on educational opportunities. The findings of this study contribute to the understanding of the factors influencing school completion in Malaysia and provide valuable insights for policymakers and educators to develop effective strategies to improve educational outcomes.
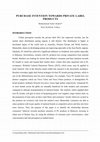
Global perception towards the private label (PL) has improved recently, but the market share dist... more Global perception towards the private label (PL) has improved recently, but the market share distribution among regions is still distinct. The distribution is higher in developed region of the world such as Australia, Western Europe and North America. Meanwhile, shares in developing nations are improving especially in the Asia Pacific regions. Similarly, PL brand has achieved a significant influence in Southeast Asia market especially in Malaysia. Nevertheless, retailers with PL products face strong competition from national brands. Retailers are looking for factors that influence consumer purchase intention towards PL brands to create and expand their market share. Culture does play important role in PL acceptance. Hofstede Cultural Dimension Theory (2015), which some, may be applied in retail research. One of the theories tested within this research is the uncertainty avoidance. Retailers nowadays apply dual format strategies for their PL brands pricing schemes, which is one of the differentiation and low price strategies. For example, Tesco PL brands have two distinct categories which are Tesco Value and Tesco Finest products. It distinguishes PL brand products attributes between low-price versus high quality respectively. Outspread PL or store brands across the globe are mutually beneficial for both retailers and consumers compared to ultimate monopolization of national brands. The retailers, which supplied their own PL brands, will gain in terms of bargaining power over supplier, larger market share on the proportion of item sales on their retail floors and customers’ loyalty which are created as the products are exclusive only from the retailer's store. Meanwhile, customers are able to enjoy products with in-par quality of national brands at competitive prices and they also have more choices. Thus, the purpose of this paper is to identify the relationship between consumers’ behavior towards purchase intention of PL products in Malaysia, focusing in urban area of Kelana Jaya, by using the attributes of consumer price consciousness, value consciousness and risk perception as studied factor.
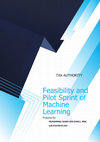
In the modern era of data-driven governance, the ability to develop and maintain robust machine l... more In the modern era of data-driven governance, the ability to develop and maintain robust machine learning (ML) models is crucial to the efficiency and success of governmental institutions like the especially for Tax Authority. The proposed ML pre-training architecture will play a critical role in optimizing tax operations, from data processing to model training and actionable insights generation. Leveraging cloud-based environments and cutting-edge ML ecosystems, this architecture will enable the tax authority to process large datasets, build intelligent models, and enhance decision-making. This proposal outlines the architecture that integrates Google Drive for secure data storage, Google Colab for computational power, and GenAI (Gemini), an AI assistant that helps human data scientists with natural language guidance. Additionally, this architecture emphasizes organizational control and ownership over the data and algorithms developed, which is essential for privacy, security, and long-term strategic advantage.
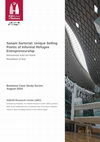
This case study focuses on Nadia Sanam, a traditional Afghani women's tailor and founder of a hom... more This case study focuses on Nadia Sanam, a traditional Afghani women's tailor and founder of a home-tailoring business, Sanam Sartorial. She faces significant difficulties as a refugee entrepreneur in Kuala Lumpur, which prohibits her from legally registering the business and accessing formal financial support, by extension limiting her ability to exploit the burgeoning Malaysian apparel market. Sanam Sartorial's key challenges, tied to her refugee status, are as follows: (1) its need to create demand in its host market; (2) a lack of brand identity; (3) language barriers; (4) a lack of market knowledge and access; and (5) financial constraints. Broadly speaking, this case study argues that she has two potential avenues to growth: (1) creating a demand for Afghani fashion among Malaysian communities; and (2) overcoming other operational challenges, namely language barriers as well as a lack of production capacity or an online storefront, to meet potential supply.
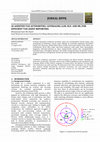
Jurnal BPPK Badan Pendidikan dan Pelatihan Keuangan, 2024
Penilaian kepatuhan tradisional memakan waktu dan membutuhkan banyak tenaga, yang menghambat prod... more Penilaian kepatuhan tradisional memakan waktu dan membutuhkan banyak tenaga, yang menghambat produktivitas otoritas pajak. Makalah ini membahas bagaimana Kecerdasan Buatan (AI), khususnya Model Bahasa Besar (LLMs), Pemrosesan Bahasa Alami (NLP), dan Pembelajaran Mesin (ML), dapat merevolusi pelaporan audit pajak. Kami menunjukkan bagaimana teknologi-teknologi ini dapat mempermudah analisis data, mengidentifikasi ketidaksesuaian, merangkum dokumen, dan bahkan mengotomatiskan pembuatan laporan, yang pada akhirnya meningkatkan efisiensi dan akurasi bagi departemen kepatuhan otoritas pajak. Traditional compliance assessment is time-consuming and labour-intensive, hindering tax authorities' productivity. This paper explores how Artificial Intelligence (AI), specifically Large Language Models (LLMs), Natural Language Processing (NLP), and Machine Learning (ML), can revolutionize tax audit reporting. We demonstrate how these technologies can streamline data analysis, identify discrepancies, summarize documents, and even automate report generation, ultimately enhancing efficiency and accuracy for tax authorities' compliance departments.
Public concern over safety and ethics hinders Tesla's autonomous vehicle fast adoption. This essa... more Public concern over safety and ethics hinders Tesla's autonomous vehicle fast adoption. This essay proposes a multiyear strategy to regain trust. Collaboration, transparency, and public education build the foundation. Data-driven, iterative improvement guided by the Kaizen philosophy ensures continuous progress. By actively monitoring public perception and engaging with stakeholders, Tesla can position itself as a leader in responsible self-driving car development.
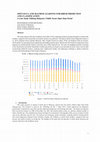
This study analyzes birth data in Malaysia from 2000 to 2023, employing machine learning techniqu... more This study analyzes birth data in Malaysia from 2000 to 2023, employing machine learning techniques to predict birth numbers, categorize birth rate periods, and explore newborn sex prediction. The analysis utilizes Linear Regression (James et al., 2013), Random Forest Classifier (Breiman, 2001), Prophet (Taylor & Letham, 2018), and XGBoost (Chen & Guestrin, 2016) models, incorporating feature engineering and handling missing values. Results show that XGBoost outperforms Linear Regression in predicting birth numbers, achieving a lower Mean Squared Error. The study also highlights potential overfitting in the classification task and the infeasibility of predicting newborn sex based on year and ethnicity alone. Future work includes incorporating additional features, exploring more sophisticated models, and addressing overfitting to enhance prediction accuracy and understanding of birth trends in Malaysia.
Uploads
Papers by MUHAMMAD SUKRI BIN RAMLI