Papers by Snehasis Mukherjee

Cornell University - arXiv, Aug 26, 2018
This paper proposes a novel technique for single image dehazing. Most of the state-of-the-art met... more This paper proposes a novel technique for single image dehazing. Most of the state-of-the-art methods for single image dehazing relies either on Dark Channel Prior (DCP) or on Color line. The proposed method combines the two different approaches. We initially compute the dark channel prior and then apply a Nearest-Neighbor (NN) based regularization technique to obtain a smooth transmission map of the hazy image. We consider the effect of airlight on the image by using the color line model to assess the commitment of airlight in each patch of the image and interpolate at the local neighborhood where the estimate is unreliable. The NN based regularization of the DCP can remove the haze, whereas, the color line based interpolation of airlight effect makes the proposed system robust against the variation of haze within an image due to multiple light sources. The proposed method is tested on benchmark datasets and shows promising results compared to the state-of-the-art, including the deep learning based methods, which require a huge computational setup. Moreover, the proposed method outperforms the recent deep learning based methods when applied on images with sky regions.
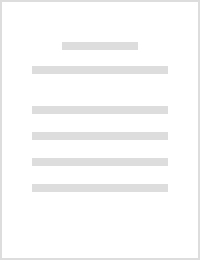
Proceedings of the 11th Indian Conference on Computer Vision, Graphics and Image Processing
In this paper we present a novel methodology for recognizing human activity in Egocentric video b... more In this paper we present a novel methodology for recognizing human activity in Egocentric video based on the Bag of Visual Features. The proposed technique is based on the assumption that, only a portion of the whole video can be sufficient to identify an activity. Rather, we argue that, for activity recognition in egocentric videos, the proposed approach performs better than any deep learning based method. Because, in egocentric videos, often the person wiring the sensor, becomes static for long time, or moves his head frequently. In both the cases, it becomes difficult to learn the spatiotemporal pattern of the video during action. The proposed approach divides the video into smaller video segments called Video Units. Spatio-temporal features extracted from the units, are clustered to construct the dictionary of Action Units (AU). The AUs are ranked based upon their score of likeliness. The scores are obtained by constructing a weighted graph with the AUs as vertices and edge weights calculated based on the frequencies of occurrences of the AUs during the activity. The less significant AUs are pruned out from the dictionary, and the revised dictionary of key AUs are used for activity classification. We test our approach on benchmark egocentric dataset and achieve a good accuracy.
Communications in Computer and Information Science, 2018
We propose a deep learning based technique to classify actions based on Long Short Term Memory (L... more We propose a deep learning based technique to classify actions based on Long Short Term Memory (LSTM) networks. The proposed scheme first learns spatial temporal features from the video, using an extension of the Convolutional Neural Networks (CNN) to 3D. A Recurrent Neural Network (RNN) is then trained to classify each sequence considering the temporal evolution of the learned features for each time step. Experimental results on the CMU MoCap, UCF 101, Hollywood 2 dataset show the efficacy of the proposed approach. We extend the proposed framework with an efficient motion feature, to enable handling significant camera motion. The proposed approach outperforms the existing deep models for each dataset.

2018 15th International Conference on Control, Automation, Robotics and Vision (ICARCV), 2018
Efficient and precise classification of histological cell nuclei is of utmost importance due to i... more Efficient and precise classification of histological cell nuclei is of utmost importance due to its potential applications in the field of medical image analysis. It would facilitate the medical practitioners to better understand and explore various factors for cancer treatment. The classification of histological cell nuclei is a challenging task due to the cellular heterogeneity. This paper proposes an efficient Convolutional Neural Network (CNN) based architecture for classification of histological routine colon cancer nuclei named as RCCNet. The main objective of this network is to keep the CNN model as simple as possible. The proposed RCCNet model consists of 1, 512, 868 learnable parameters which are significantly less compared to the popular CNN models such as AlexNet, CIFAR-VGG, GoogLeNet, and WRN. The experiments are conducted over publicly available routine colon cancer histological dataset "CRCHistoPhenotypes". The results of the proposed RCCNet model are compared with five state-ofthe-art CNN models in terms of the accuracy, weighted average F1 score and training time. The proposed method has achieved a classification accuracy of 80.61% and 0.7887 weighted average F1 score. The proposed RCCNet is more efficient and generalized in terms of the training time and data over-fitting, respectively.

Proceedings of the Twelfth Indian Conference on Computer Vision, Graphics and Image Processing, 2021
Micro-expression has emerged as a promising modality in affective computing due to its high objec... more Micro-expression has emerged as a promising modality in affective computing due to its high objectivity in emotion detection. Despite the higher recognition accuracy provided by the deep learning models, there are still significant scope for improvements in micro-expression recognition techniques. The presence of microexpressions in small-local regions of the face, as well as the limited size of available databases, continue to limit the accuracy in recognizing micro-expressions. In this work, we propose a facial microexpression recognition model using 3D residual attention network named MERANet to tackle such challenges. The proposed model takes advantage of spatial-temporal attention and channel attention together, to learn deeper fine-grained subtle features for classification of emotions. Further, the proposed model encompasses both spatial and temporal information simultaneously using the 3D kernels and residual connections. Moreover, the channel features and spatio-temporal features are re-calibrated using the channel and spatio-temporal attentions, respectively in each residual module. Our attention mechanism enables the model to learn to focus on different facial areas of interest. The experiments are conducted on benchmark facial micro-expression datasets. A superior performance is observed as compared to the state-of-the-art for facial micro-expression recognition on benchmark data. CCS CONCEPTS • Computing methodologies → Activity recognition and understanding.

2018 15th International Conference on Control, Automation, Robotics and Vision (ICARCV), 2018
Face recognition in images is an active area of interest among the computer vision researchers. H... more Face recognition in images is an active area of interest among the computer vision researchers. However, recognizing human face in an unconstrained environment, is a relatively less-explored area of research. Multiple face recognition in unconstrained environment is a challenging task, due to the variation of viewpoint , scale, pose, illumination and expression of the face images. Partial occlusion of faces makes the recognition task even more challenging. The contribution of this paper is two-folds: introducing a challenging multiface dataset (i.e., IIITS MFace Dataset) for face recognition in unconstrained environment and evaluating the performance of state-of-the-art hand-designed and deep learning based face descriptors on the dataset. The proposed IIITS MFace dataset contains faces with challenges like pose variation, occlusion, mask, spectacle, expressions, change of illumination, etc. We experiment with several state-of-the-art face descriptors, including recent deep learning based face descriptors like VGGFace, and compare with the existing benchmark face datasets. Results of the experiments clearly show that the difficulty level of the proposed dataset is much higher compared to the benchmark datasets.
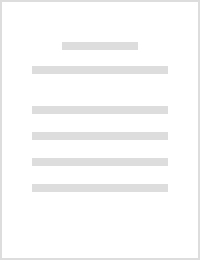
Digital Techniques for Heritage Presentation and Preservation, 2021
In this chapter, we propose a technique for the classification of yoga poses/asanas by learning t... more In this chapter, we propose a technique for the classification of yoga poses/asanas by learning the 3D landmark points in human poses obtained from a single image. We apply an encoder architecture followed by a regression layer to estimate pose parameters like shape, gesture, and camera position, which are later mapped to 3D landmark points by the SMPL (Skinned Multi-Person Linear) model. The 3D landmark points of each image are the features used for the classification of poses. We experiment with different classification models, including k-nearest neighbors (kNN), support vector machine (SVM), and some popular deep neural networks such as AlexNet, VGGNet, and ResNet. Since this is the first attempt to classify yoga asanas, no dataset is available in the literature. We propose an annotated dataset containing images of yoga poses and validate the proposed method on the newly introduced dataset.
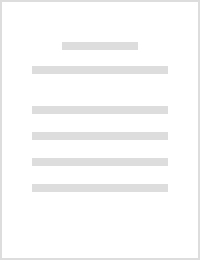
Multimedia Tools and Applications, 2017
The attractiveness of a baby face image depends on the perception of the perceiver. However, seve... more The attractiveness of a baby face image depends on the perception of the perceiver. However, several recent studies advocate the idea that human perceptual analysis can be approximated by statistical models. We believe that the cuteness of baby faces depends on the low level facial features extracted from different parts (e.g., mouth, eyes, nose) of the faces. In this paper, we introduce a new problem of classifying baby face images based on their cuteness level using supervised learning techniques. The proposed learning model finds the potential of a deep learning technique in measuring the level of cuteness of baby faces. Since no datasets are available to validate the proposed technique, we construct a dataset of images of baby faces, downloaded from the internet. The dataset consists of several challenges like different view-point, orientation, lighting condition, contrast and background. We annotate the data using some well-known statistical tools inherited from Reliability the...
2019 28th IEEE International Conference on Robot and Human Interactive Communication (RO-MAN), 2019
The problem of Scene flow estimation in depth videos has been attracting attention of researchers... more The problem of Scene flow estimation in depth videos has been attracting attention of researchers of robot vision, due to its potential application in various areas of robotics. The conventional scene flow methods are difficult to use in reallife applications due to their long computational overhead. We propose a conditional adversarial network SceneFlowGAN for scene flow estimation. The proposed SceneFlowGAN uses loss function at two ends: both generator and descriptor ends. The proposed network is the first attempt to estimate scene flow using generative adversarial networks, and is able to estimate both the optical flow and disparity from the input stereo images simultaneously. The proposed method is experimented on a huge RGB-D benchmark sceneflow dataset.
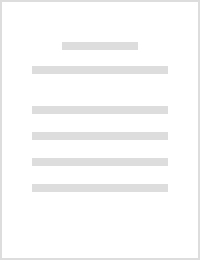
2021 National Conference on Communications (NCC), 2021
Early action prediction in video is a challenging task where the action of a human performer is e... more Early action prediction in video is a challenging task where the action of a human performer is expected to be predicted using only the initial few frames. We propose a novel technique for action prediction based on Deep Reinforcement learning, employing a Deep Q-Network (DQN) and the ResNext as the basic CNN architecture. The proposed DQN can predict the actions in videos from features extracted from the first few frames of the video, and the basic CNN model is adjusted by tuning the hyperparameters of the CNN network. The ResNext model is adjusted based on the reward provided by the DQN, and the hyperparameters are updated to predict actions. The agent's stopping criteria is higher or equal to the validation accuracy value. The DQN is rewarded based on the sequential input frames and the transition of action states (i.e., prediction of action class for an incremental 10 percent of the video). The visual features extracted from the first 10 percent of the video is forwarded to the next 10 percent of the video for each action state. The proposed method is tested on the UCF101 dataset and has outperformed the state-of-the-art in action prediction.
Journal of Visual Communication and Image Representation, 2021

Neural Networks, 2021
Transfer learning enables solving a specific task having limited data by using the pre-trained de... more Transfer learning enables solving a specific task having limited data by using the pre-trained deep networks trained on large-scale datasets. Typically, while transferring the learned knowledge from source task to the target task, the last few layers are fine-tuned (re-trained) over the target dataset. However, these layers are originally designed for the source task which might not be suitable for the target task. In this paper, we introduce a mechanism for automatically tuning the Convolutional Neural Networks (CNN) for improved transfer learning. The CNN layers are tuned with the knowledge from target data using Bayesian Optimization. Initially, we train the final layer of the base CNN model by replacing the number of neurons in the softmax layer with the number of classes involved in the target task. Next, the CNN is tuned automatically by observing the classification performance on the validation data (greedy criteria). To evaluate the performance of the proposed method, experiments are conducted on three benchmark datasets, e.g., CalTech-101, CalTech-256, and Stanford Dogs. The classification results obtained through the proposed AutoTune method outperforms the standard baseline transfer learning methods over the three datasets by achieving 95.92%, 86.54%, and 84.67% accuracy over CalTech-101, CalTech-256, and Stanford Dogs, respectively. The experimental results obtained in this study depict that tuning of the pre-trained CNN layers with the knowledge from the target dataset confesses better transfer learning ability.
Cognitive Systems Research, 2020
This is a PDF file of an article that has undergone enhancements after acceptance, such as the ad... more This is a PDF file of an article that has undergone enhancements after acceptance, such as the addition of a cover page and metadata, and formatting for readability, but it is not yet the definitive version of record. This version will undergo additional copyediting, typesetting and review before it is published in its final form, but we are providing this version to give early visibility of the article. Please note that, during the production process, errors may be discovered which could affect the content, and all legal disclaimers that apply to the journal pertain.
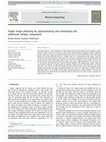
Neurocomputing, 2020
This paper proposes a novel technique for single image dehazing using adaptive nearest neighbor r... more This paper proposes a novel technique for single image dehazing using adaptive nearest neighbor regularization to obtain a haze-free transmission map and then approximating the additional airlight component present in the hazy image. The proposed method relies on the intensity distribution in the small image patches of the image, exhibited from the Y channel of the YC b C r representation of the image, in order to preserve the texture information of the image. We substitute the commonly used soft matting technique in assessing the refined transmission map for haze removal, by adaptive nearest neighbor classifier. We assume that the actual color of the haze-free pixels in the image is approximated by a set of discrete colors. We discover the haze-free pixels using the Nearest-Neighbor (NN) regularization. Finally, unlike the state-of-the-art methods, we approximate the additional airlight present in the image patch and eliminate that to clear the haze, instead of estimating the transmission of the medium. The proposed nearest neighbor regularization technique automatically changes the patch size, which helps in dealing with the high depth region (e.g., sky region) of the image. We experimented on standard synthetic and real hazy image datasets and observed that, the proposed method outperforms the state-of-the-art, especially for images with sky regions.

Multimedia Tools and Applications, 2020
Blind Image Quality Assessment (BIQA) has been an enticing research problem in image processing, ... more Blind Image Quality Assessment (BIQA) has been an enticing research problem in image processing, during the last few decades. In spite of the introduction of several BIQA algorithms, quantifying image quality without the help of a reference image still remains an unsolved problem. We propose a method for BIQA, combining Natural Scene Statistics (NSS) feature and Probabilistic Quality representation by a CNN. A certain number of features are considered for each image. We also propose to increase the NSS feature set alongside with the same CNN architecture and compare its results accordingly. Support Vector Machine (SVM) regression is applied on these features to get a quality score for that particular image. The results obtained by applying the proposed quality score on benchmark datasets, show the effectiveness of the proposed quality metric compared to the state-of-the-art metrics.

Multimedia Tools and Applications, 2020
Human Activity Recognition in RGB-D videos has been an active research topic during the last deca... more Human Activity Recognition in RGB-D videos has been an active research topic during the last decade. However, no efforts have been found in the literature, for recognizing human activity in RGB-D videos where several performers are performing simultaneously. In this paper we introduce such a challenging dataset with several performers performing the activities. We present a novel method for recognizing human activities in such videos. The proposed method aims in capturing the motion information of the whole video by producing a dynamic image corresponding to the input video. We use two parallel ResNext-101 to produce the dynamic images for the RGB video and depth video separately. The dynamic images contain only the motion information and hence, the unnecessary background information are eliminated. We send the two dynamic images extracted from the RGB and Depth videos respectively, through a fully connected layer of neural networks. The proposed dynamic image reduces the complexity of the recognition process by extracting a sparse matrix from a video. However, the proposed system maintains the required motion information for recognizing the activity. The proposed method has been tested on the MSR Action 3D dataset and has shown comparable performances with respect to the state-of-the-art. We also apply the proposed method on our own dataset, where the proposed method outperforms the state-of-the-art approaches.

Multimedia Tools and Applications, 2019
The local descriptors have gained wide range of attention due to their enhanced discriminative ab... more The local descriptors have gained wide range of attention due to their enhanced discriminative abilities. It has been proved that the consideration of multi-scale local neighborhood improves the performance of the descriptor, though at the cost of increased dimension. This paper proposes a novel method to construct a local descriptor using multi-scale neighborhood by finding the local directional order among the intensity values at different scales in a particular direction. Local directional order is the multi-radius relationship factor in a particular direction. The proposed local directional order pattern (LDOP) for a particular pixel is computed by finding the relationship between the center pixel and local directional order indexes. It is required to transform the center value into the range of neighboring orders. Finally, the histogram of LDOP is computed over whole image to construct the descriptor. In contrast to the state-of-the-art descriptors, the dimension of the proposed descriptor does not depend upon the number of neighbors involved to compute the order; it only depends upon the number of directions. The introduced descriptor is evaluated over the image retrieval framework and compared with the state-of-the-art descriptors over challenging face databases such as PaSC, LFW, PubFig, FERET, AR, AT&T, and ExtendedYale. The experimental results confirm the superiority and robustness of the LDOP descriptor.
Neurocomputing, 2019
The Convolutional Neural Networks (CNNs), in domains like computer vision, mostly reduced the nee... more The Convolutional Neural Networks (CNNs), in domains like computer vision, mostly reduced the need for handcrafted features due to its ability to learn the problem-specific features from the raw input data. However, the selection of dataset-specific CNN architecture, which mostly performed by either experience or expertise is a time-consuming and error-prone process. To automate the process of learning a CNN architecture, this paper attempts at finding the relationship between Fully Connected (FC) layers with some of the characteristics of the datasets. The CNN architectures, and recently datasets also, are categorized as deep, shallow, wide, etc. This paper tries to formalize these terms along with answering the following questions. (i) What is the impact of deeper/shallow architectures on the performance of the CNN w.r.t.
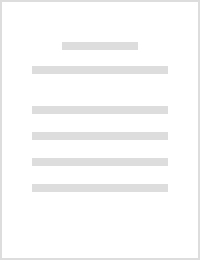
Neural Computing and Applications, 2019
Biomedical image retrieval is a challenging problem due to the varying contrast and size of struc... more Biomedical image retrieval is a challenging problem due to the varying contrast and size of structures in the images. The approaches for biomedical image retrieval generally rely on the feature descriptors to characterize the images. The feature descriptor of query image is compared with the descriptors of images from the database, to find the best matches. Several hand-crafted feature descriptors have been proposed so far for biomedical image retrieval by exploiting the local relationship of neighboring image pixels. It is observed in the literature that the local bit-plane decoded features are well suited for this retrieval task. Moreover, in recent past, it is also observed that the convolutional neural network-based features such as AlexNet, Vgg16, GoogleNet and ResNet perform well in many computer vision-related tasks. Motivated by the success of the deep learning-based approaches, this paper proposes a local bit-plane decoding-based AlexNet descriptor (LBpDAD) for biomedical image retrieval. The proposed LBpDAD is computed by max-fusing the ReLU operated feature maps of pre-trained AlexNet at a particular layer, obtained from the original and local bit-plane decoded images. The proposed approach is also compared with Vgg16, GoogleNet and ResNet models. The experiments on the proposed method over three benchmark biomedical databases of different modalities such as MRI, CT and microscopic show the efficacy of the proposed descriptor.
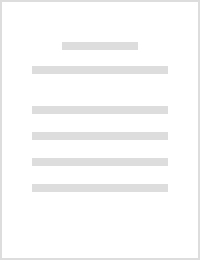
Proceedings of the Tenth Indian Conference on Computer Vision, Graphics and Image Processing - ICVGIP '16, 2016
This paper introduces two novel motion based features for recognizing human facial expressions. T... more This paper introduces two novel motion based features for recognizing human facial expressions. The proposed motion features are applied for recognizing facial expressions from a video sequence. The proposed bag-of-words based scheme represents each frame of a video sequence as a vector depicting local motion patterns during a facial expression. The local motion patterns are captured by an efficient derivation from optical flow. Motion features are clustered and stored as words in a dictionary. We further generate a reduced dictionary by ranking the words based on some ambiguity measure. We prune out the ambiguous words and continue with key words in the reduced dictionary. The ambiguity measure is given by applying a graph-based technique, where each word is represented as a node in the graph. Ambiguity measures are obtained by modelling the frequency of occurrence of the word during the expression. We form expression descriptors for each expression from the reduced dictionary, by applying an efficient kernel. The training of the expression descriptors are made following an adaptive learning technique. We tested the proposed approach with standard dataset. The proposed approach shows better accuracy compared to the state-of-the-art.
Uploads
Papers by Snehasis Mukherjee