Papers by Jose Contreras-Vidal

When holding a coffee mug filled to the brim, we strive to avoid spilling the coffee. This abilit... more When holding a coffee mug filled to the brim, we strive to avoid spilling the coffee. This ability relies on the neural processes underlying the control of finger forces on a moment-to-moment basis. The brain activity lateralized to the contralateral hemisphere averaged over a trial and across the trials is known to be associated with the magnitude of grip force applied on an object. However, the mechanistic involvement of the variability in neural signals during grip force control remains unclear. In this study, we examined the dependence of neural variability over the frontal, central, and parietal regions assessed using noninvasive electroencephalography (EEG) on grip force magnitude during an isometric force control task. We hypothesized laterally specific modulation in EEG variability with higher magnitude of the grip force exerted during grip force control. We utilized an existing EEG dataset (64 channel) comprised of healthy young adults, who performed an isometric force cont...
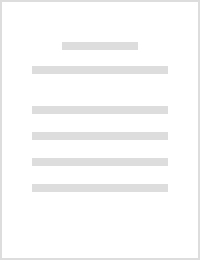
2017 IEEE International Conference on Systems, Man, and Cybernetics (SMC), 2017
Advanced powered lower-limb prosthetic devices require an intuitive and flexible user control int... more Advanced powered lower-limb prosthetic devices require an intuitive and flexible user control interface to work in a dynamic environment. This study investigated the feasibility of inferring muscle activation patterns (electromyography, EMG, envelope) from non-invasive electroencephalography (EEG) signals. Six healthy individuals participated in this study; the subjects were instructed to walk at a comfortable speed across various terrains (e.g. level-ground, up/down slope, up/down stair walking). An unscented kalman filter (UKF) was used to predict the EMG envelope from fluctuations in the amplitude of slow cortical potentials of EEG in the delta band (0.1–3 Hz). The highest decoding accuracy obtained was an r-value (Pearson's correlation r-value) of 0.57 in the medial gastrocnemius of a single subject. In the same subject, the mean r-value across all the muscle groups exceeded 0.4. The mean accuracy across all subjects and muscle group corresponded to an r-value of 0.236. As for the Signal to Noise Ratio (SNR), 79.3% of the obtained results were more than 0 dB with mean performance SNR of 0.8 (max: 2.8 to min: −1.7). The highest accuracy was obtained using a lag of 50ms with a window length (tap) of 500ms. In conclusion, this is the first study to show offline continuous decoding of the EMG envelope during over-ground walking on multiple terrains. The results show the feasibility of such neural decoding. This method could be coupled with EMG-based terrain prediction techniques to further improve the neural control interface with powered lower-limb prostheses.
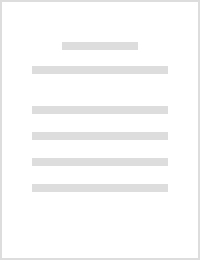
2017 International Symposium on Wearable Robotics and Rehabilitation (WeRob), 2017
Non-invasive scalp electroencephalogram (EEG) measurements allow for the development of biomedica... more Non-invasive scalp electroencephalogram (EEG) measurements allow for the development of biomedical devices that can be controlled via Brain-Machine Interface (BMI) systems. There are various applications of such systems for scientific, diagnostic, therapeutic, or restorative purposes. However, EEG recordings are often considered as prone to physiological and non-physiological artifacts of different types and frequency characteristics. Motion related non-physiological artifacts can be considered as one of the major contaminants of EEG recordings. Motion artifacts manifest themselves especially for mobile EEG recordings (i.e., Mobi applications) due to the movement of one or several EEG sensors, which may be time-locked with the actual motion that the subjects execute. Artifacts with these characteristics can hinder the true performance of BMI applications, especially when real-time mobile applications are considered (i.e., wearable robotic systems and exoskeletons). Although there several published research efforts to investigate the motion artifacts, there is currently no consensus on the exact characteristics and suitable real-time and/or offline removal methodologies of such artifacts.
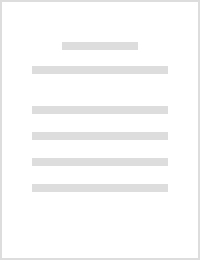
2017 IEEE International Conference on Systems, Man, and Cybernetics (SMC), 2017
Recent advancements in powered lower limb prostheses have led to the development of neural-machin... more Recent advancements in powered lower limb prostheses have led to the development of neural-machine interfaces for natural control during bipedal locomotion. In particular, electromyography (EMG) patterns recorded from the amputated limb can be leveraged to infer the intended gait pattern of the user. However, the optimal control strategy for translating the EMG patterns to kinematic space remains a challenge. In this study, six able bodied subjects were instrumented for mobile brain-body imaging and asked to walk on a multi-terrain gait course. A non-linear extension of the Kalman filter was used to predict knee and ankle joint kinematics from lower limb muscle activation patterns during overground locomotion. Specifically, muscles of the anterior and posterior thigh were used to predict both the knee and ankle joint position. The results revealed that muscles in the thigh can be used to predict the position of the knee and ankle with high accuracy. The highest mean r-value obtained for each of the six subjects was 0.92, 0.77, 0.38, 0.39, 0.63, and 0.77, with corresponding SNR values of 10.8 dB, 6.7 dB, 5.9 dB, 2.8 dB, 9.1 dB, and 9.2 dB, for each subject, respectively. This study is the first to demonstrate that continuous EMG can be used to predict the joint kinematics of the knee and ankle during overground locomotion. This approach may provide improvements during closed-loop control of a powered lower limb prosthesis when compared to other pattern-recognition based methods.
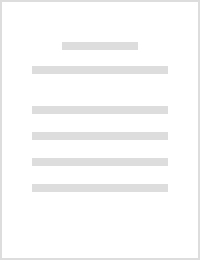
2017 IEEE International Conference on Systems, Man, and Cybernetics (SMC), 2017
Recent advances in brain-computer interface (BCI) technologies have shown the feasibility of neur... more Recent advances in brain-computer interface (BCI) technologies have shown the feasibility of neural decoding for both users' gait intent and continuous kinematics. However, the cortical adaptation and the dynamics of cortical involvement in human upright walking with a closed-loop BCI in virtual environment (VE) have yet to be demonstrated. To address explore this possibility, we designed a closed-loop BCI to allow users to control a virtual avatar to walk using their encephalography (EEG). Delta band EEG (0.1–3 Hz) was used as the main feature in prediction. Our results demonstrate the feasibility of using a closed-loop BCI to learn to control a walking avatar. The average decoding accuracies (Pearson's r values) across all subjects increased from (Hip: 0.18 ± 0.31; Knee: 0.23 ± 0.33; Ankle: 0.14 ± 0.22) on Day 1 to (Hip: 0.40 ± 0.24; Knee: 0.55 ± 0.20; Ankle: 0.29 ± 0.22) on Day 8. Source localization revealed significant differences in cortical network activity between walking with and without closed-loop BCI control of the virtual avatar. This current study demonstrates the feasibility of using a closed-loop EEG-based BCI-VR to trigger cortical adaptation, promoting cortical involvement, and monitoring cortical activity from non-invasive EEG. Our system may be relevant for neurological gait rehabilitation as a clinical tool for post-stroke physical training and clinical assessment.
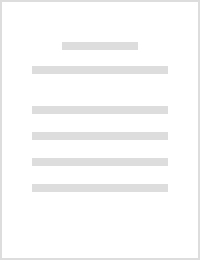
2020 42nd Annual International Conference of the IEEE Engineering in Medicine & Biology Society (EMBC), 2020
Brain Computer Interfaces (BCIs) allow individuals to control devices, machines and prostheses wi... more Brain Computer Interfaces (BCIs) allow individuals to control devices, machines and prostheses with their thoughts. Most feasibility studies with BCIs have utilized scalp electroencephalography (EEG), due to it being accessible, noninvasive, and portable. While BCIs have been studied with magnetoencephalography (MEG), the modality has limited applications due to the large immobile hardware. Here we propose that room-temperature, optically-pumped magnetometers (OPMs) can potentially serve a portable modality that can be used for BCIs. OPMs have the added advantage that low-frequency neuromagnetic fields are not affected by volume conduction, which is known to distort EEG signals. In this feasibility study, we tested an OPM system with a real-time BCI where able bodied participants controlled a cursor to reach two targets. This BCI system used alpha and beta-band power modulations associated with hand movements. Our preliminary results show significant alpha and beta-band desynchronization due to movement, as found in previous literature.
Biosystems & Biorobotics, 2021
Brain Machine Interfaces (BMI) combined with lower-limb exoskeletons can assist patients that hav... more Brain Machine Interfaces (BMI) combined with lower-limb exoskeletons can assist patients that have difficulties in walking. However, BMI need some calibration to adjust their parameters to each user. This process is time-consuming and can be fatiguing for the users. In this work, the optimal number of recordings needed to adjust a EEG-based BMI to distinguish between MI of gait and rest state has been studied based on three subjects. The results show that the BMI reaches its highest accuracy with 5 recordings.
2019 IEEE International Conference on Systems, Man and Cybernetics (SMC), 2019
The use of a brain-machine interface (BMI) in combination with powered exoskeletons can assist pa... more The use of a brain-machine interface (BMI) in combination with powered exoskeletons can assist patients with lower limb disabilities to walk again. These neurorobotic systems are commonly based on motor imagery, but their performance may suffer from lack of user engagement in the task or from cognitive load due to multi-tasking. The present paper shows a novel algorithm based on the gamma spectral band, using the Stockwell transform and a set of smoothing filters, to assess the quality of and improve the decoding of motor imagery during the use of a BMI-Rex exoskeleton system. The results computed in a pseudo-online scenario reveal a high accuracy with a very low false positive ratio.
Biosystems & Biorobotics, 2021
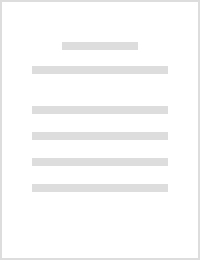
2019 IEEE International Conference on Systems, Man and Cybernetics (SMC), 2019
Powered exoskeletons for gait rehabilitation and mobility assistance are currently available for ... more Powered exoskeletons for gait rehabilitation and mobility assistance are currently available for the adult population and hold great promise for children with mobility limiting conditions. Described here is the development and key features of a modular, lightweight and customizable powered exoskeleton for assist-as-needed overground walking and gait rehabilitation. The pediatric lower-extremity gait system (PLEGS) exoskeleton contains bilaterally active hip, knee and ankle joints and assist-as-needed shared control for young children with lower-limb disabilities such as those present in the Cerebral Palsy, Spina Bifida and Spinal Cord Injured populations. The system is comprised of six joint control modules, one at each hip, knee and ankle joint. The joint control module, features an actuator and motor driver, microcontroller, torque sensor to enable assist-as-needed control, inertial measurement unit and system monitoring sensors. Bench-testing results for the proposed joint control module are also presented and discussed.
Frontiers in Bioengineering and Biotechnology, 2020
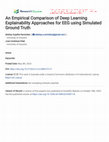
Recent advancements in machine learning and deep learning (DL) based neural decoders have signifi... more Recent advancements in machine learning and deep learning (DL) based neural decoders have significantly improved decoding capabilities using scalp electroencephalography (EEG). However, the interpretability of DL models remains an under-explored area. In this study, we compared multiple model explanation methods to identify the most suitable method for EEG and understand when some of these approaches might fail. A simulation framework was developed to evaluate the robustness and sensitivity of twelve back-propagation-based visualization methods by comparing to ground truth features. Multiple methods tested here showed reliability issues after randomizing either model weights or labels: e.g., the saliency approach, which is the most used visualization technique in EEG, was not class or model-specific. We found that DeepLift was consistently accurate as well as robust to detect the three key attributes tested here (temporal, spatial, and spectral precision). Overall, this study provid...
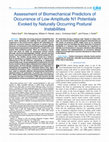
IEEE Transactions on Neural Systems and Rehabilitation Engineering, 2022
Naturally occurring postural instabilities that occur while standing and walking elicit specific ... more Naturally occurring postural instabilities that occur while standing and walking elicit specific cortical responses in the fronto-central regions (N1 potentials) followed by corrective balance responses to prevent falling. However, no framework could simultaneously track different biomechanical parameters preceding N1s, predict N1s, and assess their predictive power. Here, we propose a framework and show its utility by examining cortical activity (through electroencephalography [EEG]), ground reaction forces, and head acceleration in the anterior-posterior (AP) direction. Ten healthy young adults carried out a balance task of standing on a support surface with or without sway referencing in the AP direction, amplifying, or dampening natural body sway. Using independent components from the fronto-central cortical region obtained from subject-specific head models, we first robustly validated a prior approach on identifying low-amplitude N1 potentials before early signs of balance corrections. Then, a machine learning algorithm was used to evaluate different biomechanical parameters obtained before N1 potentials, to predict the occurrence of N1s. When different biomechanical parameters were directly compared, the time to boundary (TTB) was found to be the best predictor of the occurrence of upcoming low-amplitude
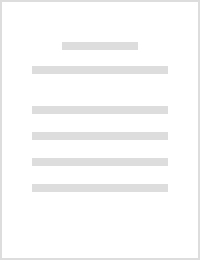
Wearable robotic devices are being designed to assist the elderly population and other patients w... more Wearable robotic devices are being designed to assist the elderly population and other patients with locomotion disabilities. However, wearable robotics increases the risk from falling. Neuroimaging studies have provided evidence for the involvement of frontocentral and parietal cortices in postural control and this opens up the possibility of using decoders for early detection of balance loss by using electroencephalography (EEG). This study investigates the presence of commonly identified components of the perturbation evoked responses (PEP) when a person is in an exoskeleton. We also evaluated the feasibility of using single-trial EEG to predict the loss of balance using a convolution neural network. Overall, the model achieved a mean 5-fold cross-validation test accuracy of 75.2 % across six subjects with 50% as the chance level. We employed a gradient class activation map-based visualization technique for interpreting the decisions of the CNN and demonstrated that the network learns from PEP components present in these single trials. The high localization ability of Grad-CAM demonstrated here, opens up the possibilities for deploying CNN for ERP/PEP analysis while emphasizing on model interpretability.
Springer eBooks, Jan 25, 2006
An architecture based on neural modules of the Adaptive Resonance Theory (ART) is proposed, for r... more An architecture based on neural modules of the Adaptive Resonance Theory (ART) is proposed, for recognizing handwritten symbols employed in an on-llne mathematical editor. The dynamic information generated during the handwriting process is used by the system, thus defining a run-on time discrete symbol as a sequence of strokes. An ART2 module is used to classify each individual stroke, while a Recurrent Competitive Field (RCF) is employed in order to classify the sequence of the strokes. ARTMAP modules are also proposed for the association of the different versions of strokes and symbols. Pre]Lminary results of the application are very encouraging.
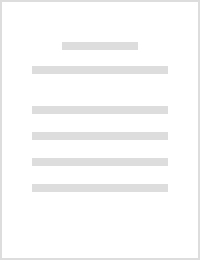
Current brain-machine interfaces (BMIs) allow upper limb amputees to position robotic arms with a... more Current brain-machine interfaces (BMIs) allow upper limb amputees to position robotic arms with a high degree of accuracy, but lack the ability to control hand pre-shaping for grasping different objects. We have previously shown that low frequency (0.1-1 Hz) time domain cortical activity recorded at the scalp via electroencephalography (EEG) encodes information about grasp pre-shaping. To transfer this technology to clinical populations such as amputees, the challenge lies in constructing BMI models in the absence of overt training hand movements. Here we show that it is possible to train BMI models using observed grasping movements performed by a robotic hand attached to amputees' residual limb. Three transradial amputees controlled the grasping motion of an attached robotic hand via their EEG, following the action-observation training phase. Over multiple sessions, subjects successfully grasped the presented object (a bottle or a credit card) in 53±16 % of trials, demonstrating the validity of the BMI models. Importantly, the validation of the BMI model was through closed-loop performance, which demonstrates generalization of the model to unseen data. These results suggest `mirror neuron system' properties captured by delta band EEG that allows neural representation for action observation to be used for action control in an EEG-based BMI system.
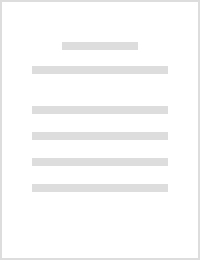
IEEE Systems Journal, Jun 1, 2021
Neurotechnology has traditionally been central to the diagnosis and treatment of neurological dis... more Neurotechnology has traditionally been central to the diagnosis and treatment of neurological disorders. While these devices have initially been utilized in clinical and research settings, recent advancements in neurotechnology have yielded devices that are more portable, user-friendly, and less expensive. These improvements allow laypeople to monitor their brain waves and interface their brains with external devices. Such improvements have led to the rise of wearable neurotechnology that is marketed to the consumer. While many of the consumer devices are marketed for innocuous applications, such as use in video games, there is potential for them to be repurposed for medical use. How do we manage neurotechnologies that skirt the line between medical and consumer applications and what can be done to ensure consumer safety? Here, we characterize neurotechnology based on medical and consumer applications and summarize currently marketed uses of consumer-grade wearable headsets. We lay out concerns that may arise due to the similar claims associated with both medical and consumer devices, the possibility of consumer devices being repurposed for medical uses, and the potential for medical uses of neurotechnology to influence commercial markets related to employment and self-enhancement.
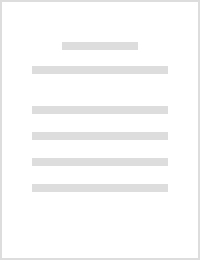
Before 2009, the feasibility of applying brain-machine interfaces (BMIs) to control prosthetic de... more Before 2009, the feasibility of applying brain-machine interfaces (BMIs) to control prosthetic devices had been limited to upper limb prosthetics such as the DARPA modular prosthetic limb. Until recently, it was believed that the control of bipedal locomotion involved central pattern generators with little supraspinal control. Analysis of cortical dynamics with electroencephalography (EEG) was also prevented by the lack of analysis tools to deal with excessive signal artifacts associated with walking. Recently, Nicolelis and colleagues paved the way for the decoding of locomotion showing that chronic recordings from ensembles of cortical neurons in primary motor (M1) and primary somatosensory (S1) cortices can be used to decode bipedal kinematics in rhesus monkeys. However, neural decoding of bipedal locomotion in humans has not yet been demonstrated. This study uses non-invasive EEG signals to decode human walking in six nondisabled adults. Participants were asked to walk on a treadmill at their self-selected comfortable speed while receiving visual feedback of their lower limbs, to repeatedly avoid stepping on a strip drawn on the treadmill belt. Angular kinematics of the left and right hip, knee and ankle joints and EEG were recorded concurrently. Our results support the possibility of decoding human bipedal locomotion with EEG. The average of the correlation values (r) between predicted and recorded kinematics for the six subjects was 0.7 (± 0.12) for the right leg and 0.66 (± 0.11) for the left leg. The average signal-to-noise ratio (SNR) values for the predicted parameters were 3.36 (± 1.89) dB for the right leg and 2.79 (± 1.33) dB for the left leg. These results show the feasibility of developing non-invasive neural interfaces for volitional control of devices aimed at restoring human gait function.
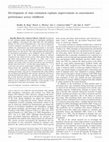
Journal of Neurophysiology, Jun 1, 2012
Previous developmental research examining sensorimotor control of the arm in school-age children ... more Previous developmental research examining sensorimotor control of the arm in school-age children has demonstrated age-related improvements in movement kinematics. However, the mechanisms that underlie these age-related improvements are still unclear. This study hypothesized that changes in sensorimotor performance across childhood can be attributed, in part, to the development of state estimation, defined as estimates computed by the central nervous system, which specify both current and future hand positions and velocities (i.e., hand "state"). Two behavioral experiments were conducted, in which 6-to 12-year-old children and young adults executed goal-directed arm movements. Results from Experiment 1 revealed that young children (i.e., ϳ6-8 years) have less precise proprioceptive feedback for static (i.e., stationary) hand state estimation compared with older children (i.e., ϳ10-12 years), resulting in increased variability of target-directed reaching movements. Experiment 2 demonstrated that young children rely on delayed and unreliable state estimates during the execution of goal-directed hand movements (i.e., dynamic state estimation), resulting in both increased movement errors and directional variability. Collectively, these results suggest that improvements in sensorimotor behavior across childhood can be attributed, at least partially, to the development of both static and dynamic state estimation. motor development; visuomotor; multisensory; reaching ADULTS EXECUTE GOAL-DIRECTED arm movements with remarkable smoothness, consistency, and accuracy (e.g., Flash and Hogan 1985; Morasso 1981). However, changes in the developing sensorimotor system and in the physical characteristics of the body during childhood can impact the control and coordination of target-directed reaches. Indeed, previous developmental research examining sensorimotor control of the arm in school-age children has demonstrated age-related differences in movement straightness and smoothness, temporal and spatial variability, and movement speed (

The control of human bipedal locomotion is of great interest to the field of lower-body brain com... more The control of human bipedal locomotion is of great interest to the field of lower-body brain computer interfaces (BCIs) for rehabilitation of gait. While the feasibility of a closed-loop BCI system for the control of a lower body exoskeleton has been recently shown, multi-day closedloop neural decoding of human gait in a virtual reality (BCI-VR) environment has yet to be demonstrated. In this study, we propose a real-time closed-loop BCI that decodes lower limb joint angles from scalp electroencephalography (EEG) during treadmill walking to control the walking movements of a virtual avatar. Moreover, virtual kinematic perturbations resulting in asymmetric walking gait patterns of the avatar were also introduced to investigate gait adaptation using the closed-loop BCI-VR system over a period of eight days. Our results demonstrate the feasibility of using a closed-loop BCI to learn to control a walking avatar under normal and altered visuomotor perturbations, which involved cortical adaptations. These findings have implications for the development of BCI-VR systems for gait rehabilitation after stroke and for understanding cortical plasticity induced by a closed-loop BCI system.
Uploads
Papers by Jose Contreras-Vidal