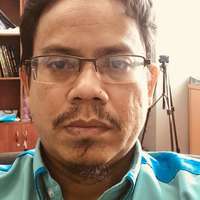
Kamarul Hawari
Dr Kamarul Hawari is a Professor at Faculty of Electrical and Electronics Engineering, Universiti Malaysia Pahang. His research interest is on imaging analysis and data analytic using deep learning.
Phone: +60177712224
Address: Faculty of Electrical and Electronics Engineering, Universiti Malaysia Pahang.
Phone: +60177712224
Address: Faculty of Electrical and Electronics Engineering, Universiti Malaysia Pahang.
less
Related Authors
Boyd Evans
Sewanee: The University of the South
Ralph Mobbs
The University of New South Wales
Leocadio Rodríguez-mañas
Universidad Europea de Madrid
Uploads
Papers by Kamarul Hawari
Gait data collection from overweight individuals walking on irregular surfaces is a challenging task that can be addressed using inertial measurement unit (IMU) sensors. However, it is unclear how many IMUs are needed, particularly when body attachment locations are not standardized. In this study, we analysed data collected from six body locations, including the torso, upper and lower limbs, to determine which locations exhibit significant variation across different real-world irregular surfaces. We then used deep learning method to verify whether the IMU data recorded from the identified body locations could classify walk patterns across the surfaces. Our results revealed two combinations of body locations, including the thigh and shank (i.e., the left and right shank, and the right thigh and right shank), from which IMU data should be collected to accurately classify walking patterns over real-world irregular surfaces (with classification accuracies of 97.24 and 95.87%, respectively). Our findings suggest that the identified numbers and locations of IMUs could potentially reduce the amount of data recorded and processed to develop a fall prevention system for overweight individuals. © 2023, Springer Nature Limited.
Gait data collection from overweight individuals walking on irregular surfaces is a challenging task that can be addressed using inertial measurement unit (IMU) sensors. However, it is unclear how many IMUs are needed, particularly when body attachment locations are not standardized. In this study, we analysed data collected from six body locations, including the torso, upper and lower limbs, to determine which locations exhibit significant variation across different real-world irregular surfaces. We then used deep learning method to verify whether the IMU data recorded from the identified body locations could classify walk patterns across the surfaces. Our results revealed two combinations of body locations, including the thigh and shank (i.e., the left and right shank, and the right thigh and right shank), from which IMU data should be collected to accurately classify walking patterns over real-world irregular surfaces (with classification accuracies of 97.24 and 95.87%, respectively). Our findings suggest that the identified numbers and locations of IMUs could potentially reduce the amount of data recorded and processed to develop a fall prevention system for overweight individuals. © 2023, Springer Nature Limited.