Papers by Roman Goldenberg
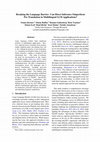
Large language models hold significant promise in multilingual applications. However, inherent bi... more Large language models hold significant promise in multilingual applications. However, inherent biases stemming from predominantly English-centric pre-training have led to the widespread practice of pre-translation, i.e., translating non-English inputs to English before inference, leading to complexity and information loss. This study re-evaluates the need for pre-translation in the context of PaLM2 models (Anil et al., 2023), which have been established as highly performant in multilingual tasks. We offer a comprehensive investigation across 108 languages and 6 diverse benchmarks, including open-end generative tasks, which were excluded from previous similar studies. Our findings challenge the pre-translation paradigm established in prior research, highlighting the advantages of direct inference in PaLM2. Specifically, PaLM2-L consistently outperforms pretranslation in 94 out of 108 languages. These findings pave the way for more efficient and effective multilingual applications, alleviating the limitations associated with pre-translation and unlocking linguistic authenticity.
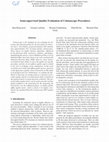
ICCV'23 - 2023 IEEE/CVF International Conference on Computer Vision Workshops (ICCVW), 2023
Colonoscopy is the standard of care technique for detecting and removing polyps for the preventio... more Colonoscopy is the standard of care technique for detecting and removing polyps for the prevention of colorectal cancer. Nevertheless, gastroenterologists (GI) routinely miss approximately 25% of polyps during colonoscopies. These misses are highly operator dependent, influenced by the physician skills, experience, vigilance, and fatigue. Standard quality metrics, such as Withdrawal Time or Cecal Intubation Rate, have been shown to be well correlated with Adenoma Detection Rate (ADR). However, those metrics are limited in their ability to assess the quality of a specific procedure, and they do not address quality aspects related to the style or technique of the examination. In this work we design novel online and offline quality metrics, based on visual appearance quality criteria learned by an ML model in an unsupervised way. Furthermore, we evaluate the likelihood of detecting an existing polyp as a function of procedure quality and use it to demonstrate high correlation of the proposed metric to polyp detection sensitivity. The proposed online quality metric can be used to provide real time quality feedback to the performing GI. By integrating the local metric over the withdrawal phase, we build a global, offline quality metric, which is shown to be highly correlated to the standard Polyp Per Colonoscopy (PPC) quality metric.
MICCAI'23 - Medical Image Computing and Computer Assisted Intervention, 2023
Computer-aided polyp detection (CADe) is becoming a standard, integral part of any modern colonos... more Computer-aided polyp detection (CADe) is becoming a standard, integral part of any modern colonoscopy system. A typical colonoscopy CADe detects a polyp in a single frame and does not track it through the video sequence. Yet, many downstream tasks including polyp characterization (CADx), quality metrics, automatic reporting, require aggregating polyp data from multiple frames. In this work we propose a robust long term polyp tracking method based on re-identification by visual appearance. Our solution uses an attention-based self-supervised ML model, specifically designed to leverage the temporal nature of video input. We quantitatively evaluate method's performance and demonstrate its value for the CADx task.
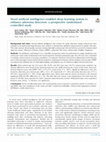
iGIE, 2023
Background and Aims: Several artificial intelligence (AI) systems for polyp detection during colo... more Background and Aims: Several artificial intelligence (AI) systems for polyp detection during colonoscopy have
emerged in the gastroenterology literature and continue to demonstrate significant improvements in quality outcomes.
This study assesses clinical quality outcomes during white-light colonoscopy with and without a novel AI computer-aided
detection system, DEtection of Elusive Polyps (DEEP2
), using Fuji 7000 series colonoscopes (Fujifilm, Singapore).
Methods: An unblinded, randomized (1:1), controlled, prospective study was performed at a single ambulatory care
endoscopy center under institutional review board approval. Included participants ages 40 to 85 years were scheduled
to undergo colonoscopy for screening, surveillance, or symptoms. Exclusion criteria were inflammatory bowel disease,
prior colorectal surgery, known polyp referral, pregnancy, inadequate bowel prep, and incomplete colonoscopies. DEEP2
was trained and validated only on white-light imaging, excluding the use of continuous digital chromoendoscopy.
Results: Mean patient age was 62.4 years (SD, 10.29), and 49% were men. Of 674 colonoscopies analyzed, significant differences were found in the adenoma detection rate (ADR) between the 2 arms of the study, those performed without versus with DEEP2 (10% vs 27%-37%, respectively; P Z .0057). Significant differences were also
found for adenomas per colonoscopy (APCs; .62 vs .39, respectively; P < .001) and polyp detection rate (17% vs
39%-56%, respectively; P < .001). In the right-sided colon, where most interval cancers are found, it also showed
significant ADR and APC differences (P < .01). The false alert rate (mean, 4 per examination) was lower than the
mean of >20 false alerts reported for other computer-aided detection systems. Withdrawal times were equivalent
between arms (mean, 7.2 minutes; not significant).
Conclusions: Seven enrolled physicians and 5 participating nurses reported a unanimous desire to continue using DEEP2 after the completion of the study and after commercial availability. (Clinical trial registration number:
MYTRIALS.) (iGIE 2023;2:52-8.)
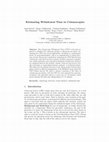
ECCV 2022 Workshops. ECCV 2022. Lecture Notes in Computer Science, Springer, Cham., 2022
The Colonoscopic Withdrawal Time (CWT) is the time required to withdraw the endoscope during a co... more The Colonoscopic Withdrawal Time (CWT) is the time required to withdraw the endoscope during a colonoscopy procedure. Estimating the CWT has several applications, including as a performance metric for gastroenterologists, and as an augmentation to polyp detection systems. We present a method for estimating the CWT directly from colonoscopy video based on three separate modules: egomotion computation; depth estimation; and anatomical landmark classification. Features are computed based on the modules’ outputs, which are then used to classify each frame as representing forward, stagnant, or backward motion. This allows for the optimal detection of the change points between these phases based on efficient maximization of the likelihood; from which the CWT follows directly. We collect a dataset consisting of 788 videos of colonoscopy procedures, with the CWT for each annotated by gastroenterologists. Our algorithm achieves a mean error of 1.20 min, which nearly matches the inter-rater disagreement of 1.17 min.
ECCV'22, "What Is Motion for?" workshop, 2022
We propose a two-stage unsupervised approach for parsing videos into phases. We use motion cues t... more We propose a two-stage unsupervised approach for parsing videos into phases. We use motion cues to divide the video into coarse segments. Noisy segment labels are then used to weakly supervise an appearance-based classifier. We show the effectiveness of the method for phase detection in colonoscopy videos.
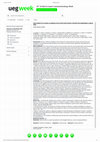
United European Gastroenterology Week (UEG), 2022
Introduction: Several artificial intelligence (AI) systems for polyp detection during colonoscopy... more Introduction: Several artificial intelligence (AI) systems for polyp detection during colonoscopy have emerged in the gastroenterology literature and continue to demonstrate significant improvements in quality outcomes. Aims & Methods: This study aimed to assess clinical quality outcomes during white light colonoscopy with and without a novel AI system. Fuji 7000 series colonoscopes were used for all exams. This was a randomized (1:1), controlled, prospective, IRB-approved, monitored trial. This clinical trial occurred at a single ambulatory care endoscopy center. Inclusion criteria included participants ages 40-85 who were previously scheduled to undergo colonoscopy for screening, surveillance, or symptoms. Exclusion criteria included suspected or active inflammatory bowel disease, past colorectal surgery, referral for known polyp removal, pregnancy, Boston bowel prep score below 6, and incomplete colonoscopy. The AI system (DEEP2) has undergone previously published feasibility and safety testing (1). The DEEP2AI system was trained and validated on white light sources, excluding the use of digital continuous chromoendoscopy during withdrawals. Results: Mean age was 62.41 years (SD 10.29), 49% were males. Of 674 colonoscopies performed, significant differences were found in ADR between the two arms of the study, those performed without vs. with AI assistance, (10%, from 27% to 37%. χ2(1)=7.65, P=0.0057, ). Significant differences were also found for APC (P = 0.0017) and PDR (15%, from 33-48%; χ2(1)=16.45, P<0.0001). When evaluated by segment of the colon, the right colon showed the largest ADR and APC differences (p=0.01). The false alert rate (mean=4/exam) was lower than the mean of 25 false alerts reported for two previously approved and available AI systems (2). Withdrawal times with vs. without the system were essentially equivalent, (mean 7.2 minutes, p=NS). Thumbnail frozen images of suspected lesions facilitated decision-making when shown alongside the real-time image, saving time. Endoscopy cuffs and caps were tested and found not to interfere with the effectiveness of the AI system. Seven enrolling physicians had varying baseline ADRs from 25-40% and reported on satisfaction exit surveys unanimous desire for continuing to use the AI system, when available. Qualitative interviews with endoscopy nurses likewise revealed very positive reactions overall to the AI system. Conclusion: The 10% improvement in ADR found when applying this novel AI system for polyp detection is on par with other highly effective systems compared in the literature (3). Specifically, the increased ADR in the right colon suggests that such AI systems can compensate for the Achilles's heel region of colonoscopy, where most interval cancers have been reported. User experience will be critical to the adoption of this technology, and the uniquely low false alert rate of the DEEP2system may contribute to reduced alert fatigue, a likely subject of future studies. Training and validating AI on electronic chromoendoscopy images such as linked color imaging and/or narrow band imaging may also enable future AI systems to be further improved by combining the quality improvement gains of chromoendoscopy and AI. Further aspirations remain that these AI systems may impact confidence in the clearance of colonic adenomas, and thus potentially increase intervals between endoscopies. Additional AI system functionalities may help save time in documentation, including photo and video documentation. Overall, AI-assisted colonoscopy shows great promise at improving colonoscopy outcomes.
Proceedings of the IEEE/CVF Winter Conference on Applications of Computer Vision, 2022
We revisit existing ensemble diversification approaches and present two novel diversification met... more We revisit existing ensemble diversification approaches and present two novel diversification methods tailored for open-set scenarios. The first method uses a new loss, designed to encourage models disagreement on outliers only, thus alleviating the intrinsic accuracy-diversity trade-off. The second method achieves diversity via automated feature engineering, by training each model to disregard input features learned by previously trained ensemble models. We conduct an extensive evaluation and analysis of the proposed techniques on seven datasets that cover image classification, re-identification and recognition domains. We compare to and demonstrate accuracy improvements over the existing state-of-the-art ensemble diversification methods.
Proceedings of the IEEE/CVF International Conference on Computer Vision, 2021
Reconstructing shapes from partial and noisy 3D data is a well-studied problem, which in recent y... more Reconstructing shapes from partial and noisy 3D data is a well-studied problem, which in recent years has been dominated by data-driven techniques. Yet in a low data regime, these techniques struggle to provide fine and accurate reconstructions. Here we focus on the relaxed problem of estimating shape coverage, i.e. asking "how much of the shape was seen?" rather than "what was the original shape?" We propose a method for unsupervised shape coverage estimation, and validate that this task can be performed accurately in a low data regime. Shape coverage estimation can provide valuable insights which pave the way for innovative applications, as we demonstrate for the case of deficient coverage detection in colonoscopy screenings.
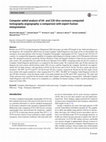
The International Journal of Cardiovascular Imaging, 2018
Routine use of CCTA to triage Emergency Department (ED) chest pain can reduce ED length of stay w... more Routine use of CCTA to triage Emergency Department (ED) chest pain can reduce ED length of stay while providing accurate diagnoses. We evaluated the effectiveness of using Computer Aided Diagnosis in the triage of low to intermediate risk emergency chest pain patients with Coronary Computed Tomographic Angiography (CCTA). Using 64 and 320 slice CT scanners, we compared the diagnostic capability of computer aided diagnosis to human readers in 923 ED patients with chest pain. We calculated sensitivity, specificity, Positive Predictive Value and Negative Predictive Value for cases performed on each scanner. We calculated the area under the Receiver Operator Curve (ROC) comparing results for the two scanners to Computer Aided Diagnosis performance as compared to the human reader. We examined index and 30 Day outcomes by diagnosis for each scanner and the human reader. 60% of cases could be triaged by the computer. Sensitivity was approximately 85% for both scanners, with specificity at 50.6% for the 64 slice and at 56.5% for the 320 slice scanner (per person measures). The NPV was 97.8 and 97.1 for the 64 and 320 slice scanners, respectively. Results for the four major vessels were similar with negative predictive values ranging from 97 to 100%. The ROC for Computer Aided Diagnosis for the 64 and 320 Slice Scanners, using the human reader as the gold standard was 0.6794 and 0.7097 respectively. The index and 30 day outcomes were consistent for the human reader and Computer Aided Diagnosis interpretation. Although Computer Aided Diagnosis with CCTA cannot serve completely as a substitute for human reading, it offers excellent potential as a triage tool in busy EDs.
2020 IEEE/CVF Conference on Computer Vision and Pattern Recognition Workshops (CVPRW), 2020
Auto-annotation by an ensemble of models is an efficient method of learning on unlabeled data. Ho... more Auto-annotation by an ensemble of models is an efficient method of learning on unlabeled data. However, wrong or inaccurate annotations generated by the ensemble may lead to performance degradation of the trained model. We propose filtering the auto-labeled data using a trained model that predicts the quality of the annotation from the degree of consensus between ensemble models. Using semantic segmentation as an example, we demonstrate the advantage of the proposed auto-annotation filtering over training on data contaminated with inaccurate labels. We show that the performance of a state-of-the-art model can be achieved by training it with only a fraction (30%) of the original manually labeled samples, and replacing the rest with auto-annotated, quality filtered labels.
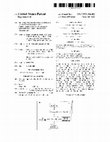
US Patent US7873194, 2011
method of automatically performing a triple rule-out procedure using imaging data is provided. I... more method of automatically performing a triple rule-out procedure using imaging data is provided. Imaging data is received that includes a heart region, a pulmonary artery region, an ascending aorta region, and a thoraco-abdominal aorta region of a patient. The heart region, an ascending aorta object, an abdominal aorta object, a left main pulmonary artery object, and a right main pulmonary artery object are identified from the imaging data. The identified heart region is analyzed to detect a coronary pathology. The identified ascending aorta object and the identified abdominal aorta object are analyzed to detect an aortic dissection. The identified left main pulmonary artery object and the identified right main pulmonary artery object are analyzed to detect a pulmonary embolism. A report is generated that includes any detected coronary pathology, any detected aortic dissection, and/or any detected pulmonary embolism.
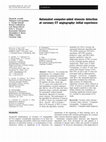
European Radiology, 2010
Objective: To evaluate the performance of a computer-aided algorithm for automated stenosis detec... more Objective: To evaluate the performance of a computer-aided algorithm for automated stenosis detection at coronary CT angiography (cCTA). Methods: We investigated 59 patients (38 men, mean age 58±12 years) who underwent cCTA and quantitative coronary angiography (QCA). All cCTA data sets were analyzed using a software algorithm for automated, without human interaction, detection of coronary artery stenosis. The performance of the algorithm for detection of stenosis of 50% or more was compared with QCA. Results: QCA revealed a total of 38 stenoses of 50% or more of which the algorithm correctly identified 28 (74%). Overall, the automated detection algorithm had 74%/100% sensitivity, 83%/65% specificity, 46%/58% positive predictive value, and 94%/100% negative predictive value for diagnosing stenosis of 50% or more on per-vessel/per-patient analysis, respectively. There were 33 false positive detection marks (average 0.56/patient), of which 19 were associated with stenotic lesions of less than 50% on QCA and 14 were not associated with an atherosclerotic surrogate. Conclusion: Compared with QCA, the automated detection algorithm evaluated has relatively high accuracy for diagnosing significant coronary artery stenosis at cCTA. If used as a second reader, the high negative predictive value may further enhance the confidence of excluding significant stenosis based on a normal or near-normal cCTA study.
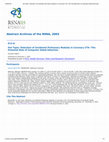
RSNA'03, 2003
Purpose: We routinely perform coronary artery calcium scoring as part of coronary artery disease ... more Purpose: We routinely perform coronary artery calcium scoring as part of coronary artery disease evaluation and also view the lunch fields for associated pathology. Our aim was to evaluate detection of lunch nodules using an automatic computer aided detection (CAD) system in patients referred for coronary CT Angiography. Materials and Methods: 3 senior radiologists and a CAD system independently assessed 65 (45 male, 20 female, mean age 53) unenhanced low dose lung MDCT studies (gate 16x1.5mm, 3mm reconstructed slice thickness) that were acquired for coronary artery calcium scoring. We used an automatic CAD system (Netzview, RCADIA Ltd., Haifa, Israel). Results: Based on all 4 evaluations a ground truth of 127 lesions in 45 patients was formed. 23/127 (19%) lesions were definite nodules in 17/45 (38%) patients. The remaining lesions: calcifications, scars, etc., 104/127 (81%) were excluded. Nodule size ranged from 1.3 – 10.0 mm. Three radiologists GB, US, NO and CAD found: 37/127 (29%), 31/127 (24%), 49/127 (39%), 85/127 (67%) of lesions respectively. Nodule detection rates were: GB 10/23 (43%), US 9/23 (39%), NP 9/23 (39%) and CAD 21/23 (91%). Taking into account all 23 definite nodules, CAD sensitivity was 91% with 2.1 FP/patient. Average sensitivity of radiologists using CAD reached 96%, showing a statistically significant improvement (p<0.003). Conclusions: Coronary CTA carries potentially significant non-coronary information. Efficient early detection and evaluation of these lung lesions becomes practical when experienced radiologists are armed with advanced CAD tools.
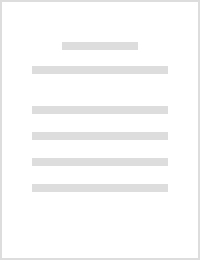
SCCT Annual Scientific Meeting (published in JCCT), 2010
Introduction: Computer‐aided detection (CAD) proved to be a useful tool for a number of medical i... more Introduction: Computer‐aided detection (CAD) proved to be a useful tool for a number of medical imaging applications. In this study we explore the applicability of CAD for coronary CT angiography (CCTA) and identify ways it can be used in different clinical scenarios. Methods: A retrospective study was conducted to assess the diagnostic performance of automated CCTA analysis system (COR Analyzer by Rcadia Medical Imaging). The study was performed on two sets of patients: 208 patients in the low risk category (as determined by the Framingham risk score) and 75 patients in the intermediate risk category (including diabetics). Both CCTA and cath lab results were available for the studies. The cath lab results were taken as the ground truth and the CT data was fed into the automatic system. The COR Analyzer automatically analyzed and reported significant coronary lesions (>50% stenosis) in 10 major coronary segments (LM, proximal, mid and distal sections of LAD, LCX and RCA). These findings were compared to the cath‐based ground truth to measure the diagnostic performance of the automatic system. Results: Each coronary segment was classified into one of the following seven categories by recording the most severe lesion reported for that segment – no obstruction, 0‐25%, 25‐50%, 40‐60%, 50‐75%, 75‐99% and total occlusion. We used the 40‐60% and 50‐75% as the significant lesions cutoff value for the low and intermediate risk groups respectively. The results comparing the COR Analyzer to the ground truth are displayed in table 1. Conclusions: The automatic CCTA analysis system exhibited very good sensitivity for significant lesions in both test groups, while maintaining the specificity at a clinically useful level. Due to the unique intrinsic properties of the CCTA exam and the exhibited CAD performance, it can be used to add value by providing a wet read for fast triage, reading sequence prioritization, workflow optimization and boosting reader’s confidence. In this way it is quite different and unique in comparison to other CAD systems.
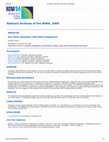
RSNA'04, 2004
PURPOSE Computer Aided Detection (CAD) systems commonly operate within a closed and separate envi... more PURPOSE Computer Aided Detection (CAD) systems commonly operate within a closed and separate environment from that of the general PACS. This limits workflow and accessibility. Our aim was to evaluate the benefits of operating a CAD system integrated with the routine PACS workflow. METHOD AND MATERIALS We used the lung nodule detection CAD system (Netzview, RCADIA Medical Imaging Ltd.) integrated with the CDP 5000 ENSEMBLE PACS system (CDP Ltd.). We ran this trial in 2 hospitals (4 multi-slice CT scanners). The PACS workflow management software was programmed to automatically forward any CT study containing lung fields to the CAD system. The CAD then analyzed and transferred the findings back to the PACS for review by the reporting radiologists. In case of positive CAD detection, a special warning is presented to the radiologist, who can toggle the CAD display at his discretion using standard PACS reading\viewing software. RESULTS The integrated CAD automatically analyzed all studies...
ICPR'02, 16th International Conference on Pattern Recognition, 2002
The paper describes a system for moving object classification. Being restricted by real-time syst... more The paper describes a system for moving object classification. Being restricted by real-time system constraints we found a small set of features, characterising object shape and motion dynamics. The system was tested on a large movies database including more than 100 images sequences showing people, animals, vehicles and plants in motion.The SVM classifier was used in our system, yielding very good classification results.
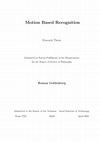
PhD Thesis - Technion - Israel Institute of Technology, Faculty of Computer Science, 2002
ABSTRACT In this work we explore how the motion based visual information can be used to solve a n... more ABSTRACT In this work we explore how the motion based visual information can be used to solve a number of well known computer vision problems such as segmentation, tracking, object recognition and classification and event detection. We consider three special cases for which the methods used are quite different: the rigid, non-rigid and articulated objects. For rigid objects we address the problem taken from the traffic domain and show how the relative velocity of nearby vehicles can be estimated from a video sequence taken by a camera installed on a moving car. For non-rigid objects we present a novel geometric variational framework for image segmentation using the active contour approach. The method is successfully used for moving non-rigid targets segmentation and tracking in color movies, as well as for a number of other applications such as cortical layer segmentation in 3-D MRI brain images, segmentation of defects in VLSI circuits on electronic microscope images, analysis of bullet traces in metals, and others. Relying on the high accuracy results of segmentation and tracking obtained by the fast geodesic contour approach, we present a framework for moving object classification based on the eigen-decomposition of the normalized binary silhouette sequence. We demonstrate the ability of the system to distinguish between various object classes by their static appearance and dynamic behavior. Finally we show how the observed articulated object motion can be used as a cue for the segmentation and detection of independently moving parts. The method is based on the analysis of normal flow vectors computed in color space and also relies on a number of geometric heuristics for edge segments grouping.
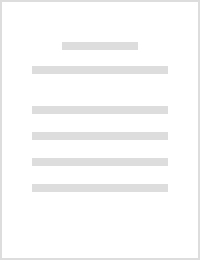
2006 International Conference on Information Technology: Research and Education, 2006
ABSTRACT Speaker identification/verification applications have progressed significantly during th... more ABSTRACT Speaker identification/verification applications have progressed significantly during the last few years. Performance levels of between 70% -99% success in speaker recognition systems are normal, depending on the type of application and quality of the signal. Several techniques for robust speaker recognition have been developed. Until now, however, the problem posed by variations in speech characteristics due to acoustical noise has not been thoroughly investigated in the context of speaker recognition. The change a noisy acoustic environment can produce in speech signal parameters is known as the &quot;Lombard effect.&quot; In this paper the Lombard effect&#39;s influence on speaker verification system performance is investigated and several compensation methods are proposed. The verification system is based on a 24 Gaussian mixture model (GMM) and speech feature orders of 12 to 60. It was found that, based on the mean Equal Error Rate (EER), verification performance deteriorated by 10.1% (from 3.8% to 13.9%) relative to speech verification in a normal environment due to the Lombard Effect. Two types of Lombard Effect compensation methods are proposed. The first is based on robust speech features that are resistant to the Lombard effect. The second is based on studying how the Lombard effect changes speech feature and then transforming the Lombard affected speech back to normal speech. The proposed methods significantly reduce speaker verification system error rates. An improvement in the EER of up to 5.4 % (from 13.5% to 8.5%) was achieved.
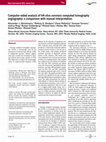
Heart International, 2013
Coronary computed tomography angiography (CCTA) is increasingly used for the assessment of corona... more Coronary computed tomography angiography (CCTA) is increasingly used for the assessment of coronary heart disease (CHD) in symptomatic patients. Software applications have recently been developed to facilitate efficient and accurate analysis of CCTA. This study aims to evaluate the clinical application of computer-aided diagnosis (CAD) software for the detection of significant coronary stenosis on CCTA in populations with low (8%), moderate (13%), and high (27%) CHD prevalence. A total of 341 consecutive patients underwent 64-slice CCTA at 3 clinical sites in the United States. CAD software performed automatic detection of significant coronary lesions (&amp;amp;amp;amp;amp;amp;amp;amp;amp;amp;amp;amp;amp;amp;amp;amp;amp;amp;amp;amp;amp;amp;amp;amp;amp;amp;amp;amp;amp;amp;amp;amp;amp;amp;amp;amp;amp;amp;gt;50% stenosis). CAD results were then compared to the consensus manual interpretation of 2 imaging experts. Data analysis was conducted for each patient and segment. The CAD had 100% sensitivity per patient across all 3 clinical sites. Specificity in the low, moderate, and high CHD prevalence populations was 64%, 41%, and 38%, respectively. The negative predictive value at the 3 clinical sites was 100%. The positive predictive value was 22%, 21%, and 38% for the low, moderate, and high CHD prevalence populations, respectively. This study demonstrates the utility of CAD software in 3 distinct clinical settings. In a low-prevalence population, such as seen in the emergency department, CAD can be used as a Computer-Aided Simple Triage tool to assist in diagnostic delineation of acute chest pain. In a higher prevalence population, CAD software is useful as an adjunct for both the experienced and inexperienced reader.
Uploads
Papers by Roman Goldenberg
emerged in the gastroenterology literature and continue to demonstrate significant improvements in quality outcomes.
This study assesses clinical quality outcomes during white-light colonoscopy with and without a novel AI computer-aided
detection system, DEtection of Elusive Polyps (DEEP2
), using Fuji 7000 series colonoscopes (Fujifilm, Singapore).
Methods: An unblinded, randomized (1:1), controlled, prospective study was performed at a single ambulatory care
endoscopy center under institutional review board approval. Included participants ages 40 to 85 years were scheduled
to undergo colonoscopy for screening, surveillance, or symptoms. Exclusion criteria were inflammatory bowel disease,
prior colorectal surgery, known polyp referral, pregnancy, inadequate bowel prep, and incomplete colonoscopies. DEEP2
was trained and validated only on white-light imaging, excluding the use of continuous digital chromoendoscopy.
Results: Mean patient age was 62.4 years (SD, 10.29), and 49% were men. Of 674 colonoscopies analyzed, significant differences were found in the adenoma detection rate (ADR) between the 2 arms of the study, those performed without versus with DEEP2 (10% vs 27%-37%, respectively; P Z .0057). Significant differences were also
found for adenomas per colonoscopy (APCs; .62 vs .39, respectively; P < .001) and polyp detection rate (17% vs
39%-56%, respectively; P < .001). In the right-sided colon, where most interval cancers are found, it also showed
significant ADR and APC differences (P < .01). The false alert rate (mean, 4 per examination) was lower than the
mean of >20 false alerts reported for other computer-aided detection systems. Withdrawal times were equivalent
between arms (mean, 7.2 minutes; not significant).
Conclusions: Seven enrolled physicians and 5 participating nurses reported a unanimous desire to continue using DEEP2 after the completion of the study and after commercial availability. (Clinical trial registration number:
MYTRIALS.) (iGIE 2023;2:52-8.)
emerged in the gastroenterology literature and continue to demonstrate significant improvements in quality outcomes.
This study assesses clinical quality outcomes during white-light colonoscopy with and without a novel AI computer-aided
detection system, DEtection of Elusive Polyps (DEEP2
), using Fuji 7000 series colonoscopes (Fujifilm, Singapore).
Methods: An unblinded, randomized (1:1), controlled, prospective study was performed at a single ambulatory care
endoscopy center under institutional review board approval. Included participants ages 40 to 85 years were scheduled
to undergo colonoscopy for screening, surveillance, or symptoms. Exclusion criteria were inflammatory bowel disease,
prior colorectal surgery, known polyp referral, pregnancy, inadequate bowel prep, and incomplete colonoscopies. DEEP2
was trained and validated only on white-light imaging, excluding the use of continuous digital chromoendoscopy.
Results: Mean patient age was 62.4 years (SD, 10.29), and 49% were men. Of 674 colonoscopies analyzed, significant differences were found in the adenoma detection rate (ADR) between the 2 arms of the study, those performed without versus with DEEP2 (10% vs 27%-37%, respectively; P Z .0057). Significant differences were also
found for adenomas per colonoscopy (APCs; .62 vs .39, respectively; P < .001) and polyp detection rate (17% vs
39%-56%, respectively; P < .001). In the right-sided colon, where most interval cancers are found, it also showed
significant ADR and APC differences (P < .01). The false alert rate (mean, 4 per examination) was lower than the
mean of >20 false alerts reported for other computer-aided detection systems. Withdrawal times were equivalent
between arms (mean, 7.2 minutes; not significant).
Conclusions: Seven enrolled physicians and 5 participating nurses reported a unanimous desire to continue using DEEP2 after the completion of the study and after commercial availability. (Clinical trial registration number:
MYTRIALS.) (iGIE 2023;2:52-8.)
relevant features from histology slide images has greatly increased
recently. However, as adoption of the technique becomes available for
more diseases, the demand for and expense of training data rises as
well. Digital pathology images are gigapixel (s) in size, and a single
slide can contain many features. These features are both very time
consuming for human annotations due to size alone, and need
trained experts, making annotations extremely expensive. To address
this problem, self-supervised pathology foundation models trained
on existing corpuses of unlabeled training data, such as The Cancer
Genome Atlas (TCGA) can be used. These foundation models can
serve as a module in the network architecture, reducing the labeled
data requirement.
Method: We take an SSL model based on vision transformers (arXiv
preprint arXiv:2310.13259 2023), which is trained on hematoxylin and
eosin (HandE) slides. The SSL model takes as input a 224 × 224 × 3
image patch and outputs an embedding vector. We apply this model
to predict features relevant to metabolic dysfunction-associated
steatohepatitis (MASH), including inflammation, steatosis, and
ballooning. The MASH-labeled data is from the CENTAUR study
(Hepatology, 72 (3), pp.892–905) which we previously analyzed (in
Modern Pathology 37.2 (2024): 100377) using fully supervised
models. Note that the original SSL model was not trained on the
images from this dataset. To apply the SSL model, we perform a twostage process. First, we use the SSL model as a component in an
architecture trained on the smaller set of labeled images to produce a
patch classification. Second, the patch classifications are aggregated
over a slide to make a slide-level prediction.
Results: While use of the SSL model produced patch-level results
similar to previous work on this dataset (Modern Pathology 37.2
(2024): 100377), superior results were obtained for grading of
ballooning and lobular inflammation slide level scores. Our model
had an AUC improved from 0.77 to 0.88 for ballooning, and 0.76 to
0.85 for lobular inflammation. Steatosis performance remained high
and consistent. We believe this reflects better generalizability of the
model, and increased resilience to messy patch-level labels. We also
show that the amount of data needed to segment features of MASH
on liver biopsy images is reduced, and in addition, training is made
simpler and faster.
Conclusion: Self-supervised foundation models can improve
machine learning performance in digital pathology, including for
liver diseases. These models can lead to better downstream
generalization, and allow training using smaller labeled datasets.
This may happen while inserting tools, or when a polyp is detected during intubation, while the treatment is postponed till the withdrawal. In both cases, a GI needs
to find and reidentify (re-id) the “lost” polyp. In such cases, if a GI fails to re-id the
polyp, precious time may be wasted searching for it. Incorrect re-id, on the other
hand, may result in missing a polyp the GI would have otherwise chosen to manage.
Polyp re-id is also required when clustering per-frame CADe detections into groups
corresponding to distinct polyps, e.g. to allow automatic reporting. Using AI to reidentify (ReID) polyps automatically, in real-time, during the colonoscopy, can
alleviate the problems outlined above.
Method: Each polyp is observed in multiple
frames in colonoscopy videos, which calls for a ReID method that leverages the
multitude of visual data coming from multiple views. One approach would be using
pairwise frame comparisons between two video segments, followed by aggregation
to reach the “same/not same polyp” decision (late fusion). Here we propose an
alternative method that ingests multiple frames all together (early fusion) and uses a
deep convolutional ML model to create a unified polyp representation (vector).
Vectors of the same polyp are expected to be close in the embedding space (see Fig.
1).
Data: To avoid labor-intensive manual annotation of duplicate polyps and increase the training set, we use contrastive learning on unlabeled data. The model is trained on 11,240 polyp video segments. Negative pairs (not the same polyp) are randomly selected over the whole set. For a positive pair (same polyp) we sample images from opposite edges of the same polyp segment. Performance is evaluated on 444 polyp segment pairs (198 positive, 246 negative). Each pair is extracted from the same procedure and manually labeled by an expert GI. Two expert GIs annotated additional 77 pairs to evaluate the interobserver agreement.
Results: Area under the ROC curve (AUC) over the test set pairs’ vector distances is shown in Fig. 2. The proposed ReID model achieved 0.8 AUC. We find this result encouraging, considering the human interrater reliability of 0.76 (Cohen’s kappa). The alternative late fusion method yields 0.7 AUC.
Conclusions: Polyp re-id is a new AI application for colonoscopy, addressing tasks that haven’t been automated before. The presented polyp ReID ML model, trained using unlabeled data, shows promising results. The proposed early fusion approach yields better accuracy than the late fusion alternative. This is likely since early fusion allows weighing multiple polyp views based on their relative quality and on how complimentary they are to each other. We believe AI-based polyp ReID can provide GIs more confidence in searching and managing polyps and lay the ground for report automation.