Papers by A. J. M. M. Weijters
Systems Research and Behavioral Science, 2002
Batch processing is becoming more important in the process industries, because of the increasing ... more Batch processing is becoming more important in the process industries, because of the increasing product variety and the decreasing demand volumes for individual products. In batch process industries it is difficult to estimate the completion time, or makespan, of a set of jobs, because jobs interact at the shop floor. We assume a situation with hierarchical production control consisting of
The deployment of Worko w Management systems is a time- consuming and error-prone task. A possibl... more The deployment of Worko w Management systems is a time- consuming and error-prone task. A possible solution is process min- ing, which automatically extracts worko w models from event-data logs. However, the current research in process mining still has problems in mining some common constructs in worko w models. Among these con- structs are short loops, which are loops of
Neural Processing Letters, 1995
For some problems, the back-propagation learning rule often used for training multilayer feedforw... more For some problems, the back-propagation learning rule often used for training multilayer feedforward networks appears to have serious limitations. In this paper we describe BP-SOM, an alternative training procedure. In BP-SOM the traditional back-propagation learning rule is combined with unsupervised learning in self-organizing maps. While the multilayer feedforward network is trained, the hidden-unit activations of the feedforward network are used

European Journal of Operational Research, 2003
Batch processing is becoming more important in the process industries, because of the increasing ... more Batch processing is becoming more important in the process industries, because of the increasing product variety and the decreasing demand volumes for individual products. In batch process industries it is difficult to estimate the completion time, or makespan, of a set of jobs, because jobs interact at the shop floor. We assume a situation with hierarchical production control consisting of a planning level and a scheduling level. In this paper we focus on the planning level. We use two different techniques for estimating the makespan of job sets in batch process industries. The first technique estimates the makespan of a job set by developing regression models, the second technique by training neural networks. Both techniques use aggregate information. By using aggregate information the presented techniques are less time consuming in assessing the makespan of a job set compared with methods based on detailed information.
Reactivity of Solids, 2007
Although there has been a lot of progress in developing pro- cess mining algorithms in recent yea... more Although there has been a lot of progress in developing pro- cess mining algorithms in recent years, no eort has been put in devel- oping a common means of assessing the quality of the models discovered by these algorithms. In this paper, we outline elements of an evaluation framework that is intended to enable (a) process mining researchers to compare
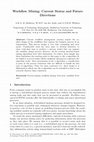
Lecture Notes in Computer Science, 2003
Current workflow management systems require the explicit design of the workflows that express the... more Current workflow management systems require the explicit design of the workflows that express the business process of an organization. This process design is very time consuming and error prone. Considerable work has been done to develop heuristics to mine event-data logs to produce a process model that can support the workflow design process. However, all the existing heuristic-based mining algorithms have their limitations. To achieve more insight into these limitations the starting point in this paper is the α-algorithm for which it is proved under which conditions and process constructs the algorithm works. After presentation of the α-algorithm, a classification is given of the process constructs that are difficult to handle for this type of algorithms. Then, for some constructs (i.e. short loops) it is illustrated in which way the α-algorithm can be extended so that it can correctly discover these constructs.
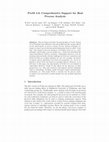
Lecture Notes in Computer Science, 2007
This tool paper describes the functionality of ProM. Version 4.0 of ProM has been released at the... more This tool paper describes the functionality of ProM. Version 4.0 of ProM has been released at the end of 2006 and this version reflects recent achievements in process mining. Process mining techniques attempt to extract non-trivial and useful information from so-called "event logs". One element of process mining is control-flow discovery, i.e., automatically constructing a process model (e.g., a Petri net) describing the causal dependencies between activities. Control-flow discovery is an interesting and practically relevant challenge for Petri-net researchers and ProM provides an excellent platform for this. For example, the theory of regions, genetic algorithms, free-choice-net properties, etc. can be exploited to derive Petri nets based on example behavior. However, as we will show in this paper, the functionality of ProM 4.0 is not limited to control-flow discovery. ProM 4.0 also allows for the discovery of other perspectives (e.g., data and resources) and supports related techniques such as conformance checking, model extension, model transformation, verification, etc. This makes ProM a versatile tool for process analysis which is not restricted to model analysis but also includes log-based analysis.
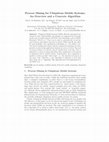
Lecture Notes in Computer Science, 2004
Ubiquitous Mobile Systems (UMSs) allow for automated capturing of events. Both mobility and ubiqu... more Ubiquitous Mobile Systems (UMSs) allow for automated capturing of events. Both mobility and ubiquity are supported by electronic means such as mobile phones and PDAs and technologies such as RFID, Bluetooth, WLAN, etc. These can be used to automatically record human behavior and business processes in detail. UMSs typically also allow for more flexibility. The combination of flexibility (i.e., the ability to deviate from standard procedures) and the automated capturing of events, provides an interesting application domain for process mining. The goal of process mining is to discover process models from event logs. The αalgorithm is a process mining algorithm whose application is not limited to ubiquitous and/or mobile systems. Unfortunately, the α-algorithm is unable to tackle so-called "short loops", i.e., the repeated occurrence of the same event. Therefore, a new algorithm is proposed to deal with short loops: the α + -algorithm. This algorithm has been implemented in the EMiT tool.

Lecture Notes in Computer Science, 2005
Under the umbrella of buzzwords such as "Business Activity Monitoring" (BAM) and "Business Proces... more Under the umbrella of buzzwords such as "Business Activity Monitoring" (BAM) and "Business Process Intelligence" (BPI) both academic (e.g., EMiT, Little Thumb, InWoLvE, Process Miner, and MinSoN) and commercial tools (e.g., ARIS PPM, HP BPI, and ILOG JViews) have been developed. The goal of these tools is to extract knowledge from event logs (e.g., transaction logs in an ERP system or audit trails in a WFM system), i.e., to do process mining. Unfortunately, tools use different formats for reading/storing log files and present their results in different ways. This makes it difficult to use different tools on the same data sets and to compare the mining results. Furthermore, some of these tools implement concepts that can be very useful in the other tools but it is often difficult to combine tools. As a result, researchers working on new process mining techniques are forced to build a mining infrastructure from scratch or test their techniques in an isolated way, disconnected from any practical applications. To overcome these kind of problems, we have developed the ProM framework, i.e., an "plugable" environment for process mining. The framework is flexible with respect to the input and output format, and is also open enough to allow for the easy reuse of code during the implementation of new process mining ideas. This paper introduces the ProM framework and gives an overview of the plug-ins that have been developed.
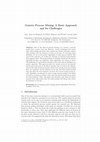
Lecture Notes in Computer Science, 2006
One of the aims of process mining is to retrieve a process model from a given event log. However,... more One of the aims of process mining is to retrieve a process model from a given event log. However, current techniques have problems when mining processes that contain non-trivial constructs and/or when dealing with the presence of noise in the logs. To overcome these problems, we try to use genetic algorithms to mine process models. The non-trivial constructs are tackled by choosing an internal representation that supports them. The noise problem is naturally tackled by the genetic algorithm because, per definition, these algorithms are robust to noise. The definition of a good fitness measure is the most critical challenge in a genetic approach. This paper presents the current status of our research and the pros and cons of the fitness measure that we used so far. Experiments show that the fitness measure leads to the mining of process models that can reproduce all the behavior in the log, but these mined models may also allow for extra behavior. In short, the current version of the genetic algorithm can already be used to mine process models, but future research is necessary to always ensure that the mined models do not allow for extra behavior. Thus, this paper also discusses some ideas for future research that could ensure that the mined models will always only reflect the behavior in the log.
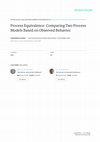
Lecture Notes in Computer Science, 2006
In various application domains there is a desire to compare process models, e.g., to relate an or... more In various application domains there is a desire to compare process models, e.g., to relate an organization-specific process model to a reference model, to find a web service matching some desired service description, or to compare some normative process model with a process model discovered using process mining techniques. Although many researchers have worked on different notions of equivalence (e.g., trace equivalence, bisimulation, branching bisimulation, etc.), most of the existing notions are not very useful in this context. First of all, most equivalence notions result in a binary answer (i.e., two processes are equivalent or not). This is not very helpful, because, in real-life applications, one needs to differentiate between slightly different models and completely different models. Second, not all parts of a process model are equally important. There may be parts of the process model that are rarely activated while other parts are executed for most process instances. Clearly, these should be considered differently. To address these problems, this paper proposes a completely new way of comparing process models. Rather than directly comparing two models, the process models are compared with respect to some typical behavior. This way we are able to avoid the two problems. Although the results are presented in the context of Petri nets, the approach can be applied to any process modeling language with executable semantics.
Lecture Notes in Computer Science, 2002
Workflow management technology requires the existence of explicit process models, i.e. a complete... more Workflow management technology requires the existence of explicit process models, i.e. a completely specified workflow design needs to be developed in order to enact a given workflow process. Such a workflow design is time consuming and often subjective and incomplete. We propose a learning method that uses the workflow log, which contains information about the process as it is actually being executed. In our method we will use a logistic regression model to discover the direct connections between events of a realistic not complete workflow log with noise. Experimental results are used to show the usefulness and limitations of the presented method.
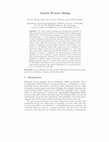
Lecture Notes in Computer Science, 2005
The topic of process mining has attracted the attention of both researchers and tool vendors in t... more The topic of process mining has attracted the attention of both researchers and tool vendors in the Business Process Management (BPM) space. The goal of process mining is to discover process models from event logs, i.e., events logged by some information system are used to extract information about activities and their causal relations. Several algorithms have been proposed for process mining. Many of these algorithms cannot deal with concurrency. Other typical problems are the presence of duplicate activities, hidden activities, non-free-choice constructs, etc. In addition, real-life logs contain noise (e.g., exceptions or incorrectly logged events) and are typically incomplete (i.e., the event logs contain only a fragment of all possible behaviors). To tackle these problems we propose a completely new approach based on genetic algorithms. As can be expected, a genetic approach is able to deal with noise and incompleteness. However, it is not easy to represent processes properly in a genetic setting. In this paper, we show a genetic process mining approach using the so-called causal matrix as a representation for individuals. We elaborate on the relation between Petri nets and this representation and show that genetic algorithms can be used to discover Petri net models from event logs.
Lecture Notes in Computer Science, 1997
Language learning has thus far not been a hot application for machine-learning (ML) research. Thi... more Language learning has thus far not been a hot application for machine-learning (ML) research. This limited attention for work on empirical learning of language knowledge and behaviour from text and speech data seems unjusti ed. After all, it is becoming apparent that empirical learning of Natural Language Processing (NLP) can alleviate NLP's all-time main problem, viz. the knowledge acquisition bottleneck: empirical ML methods such as rule induction, top down induction of decision trees, lazy learning, inductive logic programming, and some types of neural network learning, seem to be excellently suited to automatically induce exactly that knowledge that is hard to gather by hand. In this paper we address the question why NLP is an interesting application for empirical ML, and provide a brief overview of current work in this area.
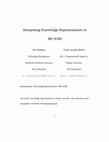
Behaviormetrika, 1999
Artificial Neural Networks (ANNs) are able, in general and in principle, to learn complex tasks. ... more Artificial Neural Networks (ANNs) are able, in general and in principle, to learn complex tasks. Interpretation of models induced by ANNs, however, is often extremely difficult due to the non-linear and non-symbolic nature of the models. To enable better interpretation of the way knowledge is represented in ANNs, we present BP-SOM, a neural network architecture and learning algorithm. BP-SOM is a combination of a multi-layered feed-forward network (MFN) trained with the back-propagation learning rule (BP), and Kohonen's self-organising maps (SOMs). The involvement of the SOM in learning leads to highly structured knowledge representations both at the hidden layer and on the SOMs. We focus on a particular phenomenon within trained BP-SOM networks, viz. that the SOM part acts as an organiser of the leaqrning material into instance subsets that tend to be homogeneous with respect to both class labelling and subsets of attribute values. We show that the structured knowledge representation can either be exploited directly for rule extraction, or be used to explain a generic type of checksum solution found by the network for learning M-of-N tasks.
Lecture Notes in Computer Science (including subseries Lecture Notes in Artificial Intelligence and Lecture Notes in Bioinformatics), 2008
Although there has been much progress in developing process mining algorithms in recent years, no... more Although there has been much progress in developing process mining algorithms in recent years, no effort has been put in developing a common means of assessing the quality of the models discovered by these algorithms. In this paper, we motivate the need for such an evaluation mechanism, and outline elements of an evaluation framework that is intended to enable (a) process mining researchers to compare the performance of their algorithms, and (b) end users to evaluate the validity of their process mining results.

Lecture Notes in Computer Science, 2008
Process mining techniques attempt to extract non-trivial and useful information from event logs r... more Process mining techniques attempt to extract non-trivial and useful information from event logs recorded by information systems. For example, there are many process mining techniques to automatically discover a process model based on some event log. Most of these algorithms perform well on structured processes with little disturbances. However, in reality it is difficult to determine the scope of a process and typically there are all kinds of disturbances. As a result, process mining techniques produce spaghetti-like models that are difficult to read and that attempt to merge unrelated cases. To address these problems, we use an approach where the event log is clustered iteratively such that each of the resulting clusters corresponds to a coherent set of cases that can be adequately represented by a process model. The approach allows for different clustering and process discovery algorithms. In this paper, we provide a particular clustering algorithm that avoids over-generalization and a process discovery algorithm that is much more robust than the algorithms described in literature . The whole approach has been implemented in ProM. . Example of an event log (with 300 process instances) for the process of a one-day conference. Each row refers to process instances that follow a similar pattern in terms of tasks being executed. The first row corresponds to 80 process instances that all followed the event sequence indicated.
Uploads
Papers by A. J. M. M. Weijters