Papers by Daniel Fernandez
Recent research has developed a group of likelihood-based finite mixture models for a data matrix... more Recent research has developed a group of likelihood-based finite mixture models for a data matrix with ordinal data, establishing
likelihood-based multivariate methods which applies fuzzy clustering via finite mixtures to the ordered stereotype model.
There are many visualisation tools which depict reduction of dimensionality in matrices of ordinal data. This technical report introduces the spaced mosaic plot which
is one new graphical tool for ordinal data when the ordinal stereotype model is used.
It takes advantage of the fitted score parameters to determine the spacing between
two adjacent ordinal categories.
We develop a function in R and its documentation is presented.
Finally, the description of a spaced mosaic plot is shown.
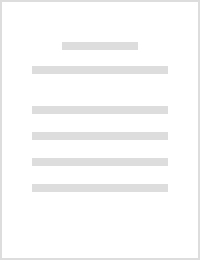
Argáez-Sosa, J., Christen, J. A., Nakamura, M., Fernandez, D., Blaauw, M. and Gomez, C. O. (2005). BioP: Biological Prediction. Research Centre of Mathematics (CIMAT). All species of animals and plants occupy a more or less well-defined geographical region, called ... more All species of animals and plants occupy a more or less well-defined geographical region, called their areas, or ranges, of distribution. It is one of the most fundamental expressions of its ecology and evolutionary history (Udvardy, 1969; Brown, Stevens and Kaufman, 1996; Gaston and Blackburn, 2000). A species distribution is the product of a complex combination of processes (Gaston and Blackburn, 2000), that begin with the apparition of the species by evolution, and its movements in ecological and geological times. The distribution is determined by factors affecting: (1) the regions in space where the right autoecological conditions for the survival of the species (climate, soil, energy availability, etc.) are met, (2) the regions of space that are available to the dispersal of the species, and (3) the presence or absence of crucial population interactions, that is, key competitors, predators, diseases, and mutualists (seed dispersers, pollinators, and so on). It is quite likely that at different spatial and temporal scales this complex of factors operate with different intensity.
Detailed knowledge of distribution areas is relevant to address basic questions in biogeography and ecology, but it is also useful in the management of biodiversity for conservation or sustainable use. Problems like the relative roles of ecological and historical factors in shaping them, how the shape of the area changes with the spatial scale of observation, and the relative importance of the local (or alpha) and the turnover (or beta) components of biodiversity, depend on being able to estimate in detail such areas. Moreover, such detailed knowledge can be used to determine the areas best suited for conservation, the regions where given activities can endanger protected species, and so on.
Unfortunately, for most species, the knowledge biologists have about distribution areas is very rough and often reduced to the few localities where a species has been observed. Ecologists and biogeographers determine the area of distribution by starting with ‘‘points’’ (in practice, localities) where the species has been registered or observed. A number of informal procedures are used to extrapolate from a cloud of points in the geographical space to a set of polygons that represent the area of distribution (Jennrich and Turner, 1969; Udvardy, 1969; Rapoport, 1975). Generally speaking, such extrapolation is entirely based on the field experience of the researchers and it is done at a very rough scale. The fundamental data that biogeographers use to base their extrapolations are the presence points. Detailed faunistic or floristic studies yield lists of observations of species in localities. Absences are mostly inferred from knowledge of the biology of the species, or from the experience of field biologists. Thus, an important feature of the data available in this setting is that one may only be certain of sites of presence, whereas sites of absence are not readily available. The problem in this paper is to infer zones of high probability for the habitat of species, using only reported sites of presence. When legitimate sites of absence are also available, this problem can be approached from many directions, such as generalized linear models (Austin, 2002), autologistic models (Pettitt, Weir and Hart, 2002), or Kriging (Heagerty and Lele, 1998).
Conference Presentations by Daniel Fernandez
Uploads
Papers by Daniel Fernandez
likelihood-based multivariate methods which applies fuzzy clustering via finite mixtures to the ordered stereotype model.
There are many visualisation tools which depict reduction of dimensionality in matrices of ordinal data. This technical report introduces the spaced mosaic plot which
is one new graphical tool for ordinal data when the ordinal stereotype model is used.
It takes advantage of the fitted score parameters to determine the spacing between
two adjacent ordinal categories.
We develop a function in R and its documentation is presented.
Finally, the description of a spaced mosaic plot is shown.
Detailed knowledge of distribution areas is relevant to address basic questions in biogeography and ecology, but it is also useful in the management of biodiversity for conservation or sustainable use. Problems like the relative roles of ecological and historical factors in shaping them, how the shape of the area changes with the spatial scale of observation, and the relative importance of the local (or alpha) and the turnover (or beta) components of biodiversity, depend on being able to estimate in detail such areas. Moreover, such detailed knowledge can be used to determine the areas best suited for conservation, the regions where given activities can endanger protected species, and so on.
Unfortunately, for most species, the knowledge biologists have about distribution areas is very rough and often reduced to the few localities where a species has been observed. Ecologists and biogeographers determine the area of distribution by starting with ‘‘points’’ (in practice, localities) where the species has been registered or observed. A number of informal procedures are used to extrapolate from a cloud of points in the geographical space to a set of polygons that represent the area of distribution (Jennrich and Turner, 1969; Udvardy, 1969; Rapoport, 1975). Generally speaking, such extrapolation is entirely based on the field experience of the researchers and it is done at a very rough scale. The fundamental data that biogeographers use to base their extrapolations are the presence points. Detailed faunistic or floristic studies yield lists of observations of species in localities. Absences are mostly inferred from knowledge of the biology of the species, or from the experience of field biologists. Thus, an important feature of the data available in this setting is that one may only be certain of sites of presence, whereas sites of absence are not readily available. The problem in this paper is to infer zones of high probability for the habitat of species, using only reported sites of presence. When legitimate sites of absence are also available, this problem can be approached from many directions, such as generalized linear models (Austin, 2002), autologistic models (Pettitt, Weir and Hart, 2002), or Kriging (Heagerty and Lele, 1998).
Conference Presentations by Daniel Fernandez
likelihood-based multivariate methods which applies fuzzy clustering via finite mixtures to the ordered stereotype model.
There are many visualisation tools which depict reduction of dimensionality in matrices of ordinal data. This technical report introduces the spaced mosaic plot which
is one new graphical tool for ordinal data when the ordinal stereotype model is used.
It takes advantage of the fitted score parameters to determine the spacing between
two adjacent ordinal categories.
We develop a function in R and its documentation is presented.
Finally, the description of a spaced mosaic plot is shown.
Detailed knowledge of distribution areas is relevant to address basic questions in biogeography and ecology, but it is also useful in the management of biodiversity for conservation or sustainable use. Problems like the relative roles of ecological and historical factors in shaping them, how the shape of the area changes with the spatial scale of observation, and the relative importance of the local (or alpha) and the turnover (or beta) components of biodiversity, depend on being able to estimate in detail such areas. Moreover, such detailed knowledge can be used to determine the areas best suited for conservation, the regions where given activities can endanger protected species, and so on.
Unfortunately, for most species, the knowledge biologists have about distribution areas is very rough and often reduced to the few localities where a species has been observed. Ecologists and biogeographers determine the area of distribution by starting with ‘‘points’’ (in practice, localities) where the species has been registered or observed. A number of informal procedures are used to extrapolate from a cloud of points in the geographical space to a set of polygons that represent the area of distribution (Jennrich and Turner, 1969; Udvardy, 1969; Rapoport, 1975). Generally speaking, such extrapolation is entirely based on the field experience of the researchers and it is done at a very rough scale. The fundamental data that biogeographers use to base their extrapolations are the presence points. Detailed faunistic or floristic studies yield lists of observations of species in localities. Absences are mostly inferred from knowledge of the biology of the species, or from the experience of field biologists. Thus, an important feature of the data available in this setting is that one may only be certain of sites of presence, whereas sites of absence are not readily available. The problem in this paper is to infer zones of high probability for the habitat of species, using only reported sites of presence. When legitimate sites of absence are also available, this problem can be approached from many directions, such as generalized linear models (Austin, 2002), autologistic models (Pettitt, Weir and Hart, 2002), or Kriging (Heagerty and Lele, 1998).