Papers by Mohamed Chetouani
Lecture Notes in Computer Science, 2009
In recent years, the established link between the various human communication production domains ... more In recent years, the established link between the various human communication production domains has become more widely utilised in the field of speech processing. In this work, a state of the art Semi Adaptive Appearance Model (SAAM) approach developed by the authors is used for automatic lip tracking, and an adapted version of our vowel based speech segmentation system is employed to automatically segment speech. Canonical Correlation Analysis (CCA) on segmented and non segmented data in a range of noisy speech environments finds that segmented speech has a significantly better audiovisual correlation, demonstrating the feasibility of our techniques for further development as part of a proposed audiovisual speech enhancement system.
Lecture Notes in Computer Science, 2008
This paper is dedicated to the description and the study of a new feature extraction approach for... more This paper is dedicated to the description and the study of a new feature extraction approach for emotion recognition. Our contribution is based on the extraction and the characterization of phonemic units such as vowels and consonants, which are provided by a pseudo-phonetic speech segmentation phase combined with a vowel detector. The segmentation algorithm is evaluated on both emotional (Berlin) and non-emotional (TIMIT, NTIMIT) databases. Concerning the emotion recognition task, we propose to extract MFCC acoustic features from these pseudo-phonetic segments (vowels, consonants) and we compare this approach with traditional voice and unvoiced segments. The classification is achieved by the well-known k-nn classifier (k nearest neighbors) on the Berlin corpus.
2009 3rd International Conference on Signals, Circuits and Systems (SCS), 2009
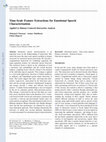
Cognitive Computation, 2009
Emotional speech characterization is an important issue for the understanding of interaction. Thi... more Emotional speech characterization is an important issue for the understanding of interaction. This article discusses the time-scale analysis problem in feature extraction for emotional speech processing. We describe a computational framework for combining segmental and supra-segmental features for emotional speech detection. The statistical fusion is based on the estimation of local a posteriori class probabilities and the overall decision employs weighting factors directly related to the duration of the individual speech segments. This strategy is applied to a real-world application: detection of Italian motherese in authentic and longitudinal parent-infant interaction at home. The results suggest that short-and long-term information, respectively, represented by the short-term spectrum and the prosody parameters (fundamental frequency and energy) provide a robust and efficient time-scale analysis. A similar fusion methodology is also investigated by the use of a phonetic-specific characterization process. This strategy is motivated by the fact that there are variations across emotional states at the phoneme level. A timescale based on both vowels and consonants is proposed and it provides a relevant and discriminant feature space for acted emotion recognition. The experimental results on two different databases Berlin (German) and Aholab (Basque) show that the best performance are obtained by our phoneme-dependent approach. These findings demonstrate the relevance of taking into account phoneme dependency (vowels/consonants) for emotional speech characterization.
Neuropsychiatrie de l'Enfance et de l'Adolescence, 2012
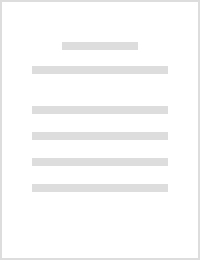
Emotional speech characterization is an important issue for the understanding of interaction. Thi... more Emotional speech characterization is an important issue for the understanding of interaction. This article discusses the time-scale analysis problem in feature extraction for emotional speech processing. We describe a computational framework for combining segmental and supra-segmental features for emotional speech detection. The statistical fusion is based on the estimation of local a posteriori class probabilities and the overall decision employs weighting factors directly related to the duration of the individual speech segments. This strategy is applied to a real-world application: detection of Italian motherese in authentic and longitudinal parent-infant interaction at home. The results suggest that short- and long-term infor- mation, respectively, represented by the short-term spec- trum and the prosody parameters (fundamental frequency and energy) provide a robust and efficient time-scale analysis. A similar fusion methodology is also investigated by the use of a phonetic-spec...
energy
This paper is devoted to the study of a pseudo-phonetic approach to characterize prosodic disorde... more This paper is devoted to the study of a pseudo-phonetic approach to characterize prosodic disorders of children with impaired communication skills. To this purpose, we have designed with the help of the clinicians' staff a database containing autistic children. Another database with non disordered speech is used as a control one. Concerning the characterization of the prosodic disorders, we extract the features from phonemic units such as vowels. These segments are provided by a pseudo-phonetic speech segmentation phase combined with a vowel detector. Since the pseudo-phonetic segments convey a lot of prosodic features, such as duration and rhythm, many differentiations can be made between children from the two studied databases. As a conclusion, correlations between prosodic particularities got in this study and those described in the literature are given.
... a permis de caractériser deux groupes de langue décrites dans la littérature : accentuelle (a... more ... a permis de caractériser deux groupes de langue décrites dans la littérature : accentuelle (anglais, allemand et mandarin) et syllabique (français et espagnol). ... ils sont à nouveau supérieurs à ceux obtenus sur les segments voisés (table 4). A titre de comparaison, Shami et al. ...
The ability to perceive and express emotions, through the expressivity of the face and the voice ... more The ability to perceive and express emotions, through the expressivity of the face and the voice is developped during the early stages of the children's life, and has an essential role in the development of the intersubjectivity. The access to intersubjectivity, communication and language is seriously failed in the autistic syndrom. The purpose of our work is to explore prosodic
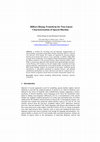
A method for non-linear and non-stationary characterisation of speech rhythm is presented using H... more A method for non-linear and non-stationary characterisation of speech rhythm is presented using Hilbert Huang Transform (HHT) of 'Speech Unit Intervals' (SUI) signals. SUI signals are supported by intervals duration between given speech units such as vowel, consonant, or syllable. While HHT is based on the combination of the Empirical Mode Decomposition (EMD) and the Hilbert transform of the provided Intrinsic Mode Functions (IMFs). Since EMD is a data-driven approach which includes both signal-dependent and timevariant filtering, HHT analysis on the SUI signals makes it possible non-linear and non-stationary characterisation of the speech rhythm. Investigations on the HHT based rhythmic features are presented in this paper: emotional speech classification is individually performed on rhythmic features, and obtained classification probabilities are fused with those provided by a typical state-ofthe-art emotion recognition system based on acoustic and prosodic features sets.
This paper is devoted to the description of a new approach for emotion recognition. Our contribut... more This paper is devoted to the description of a new approach for emotion recognition. Our contribution is based on both the extraction and the characterization of phonemic units such as vowels and consonants, which are provided by a pseudo- phonetic speech segmentation phase combined with a vowel detector. Concerning the emotion recognition task, we explore acoustic and prosodic features from these pseudo-phonetic segments (vowels and consonants), and we compare this approach with traditional voiced and unvoiced segments. The classification is realized by the well-known k-nn classifier (k nearest neighbors) from two different emotional speech databases: Berlin (German) and Aholab (Basque). Index Terms : emotion recognition, automatic speech segmentation, vowel detection
Whereas rhythmic speech analysis is known to bear great potential for the recognition of emotion,... more Whereas rhythmic speech analysis is known to bear great potential for the recognition of emotion, it is often omitted or reduced to the speaking rate or segmental durations. An obvious explanation is that the characterisation of speech rhythm is not an easy task itself and there exist many types of rhythmic information. In this paper, we study advanced methods to define novel metrics of speech rhythm. Their ability to characterise spontaneous emotions is demonstrated on the recent Audio/Visual Emotion Challenge ...
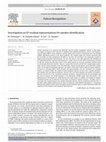
Pattern Recognition, 2009
Feature extraction is an essential and important step for speaker recognition systems. In this pa... more Feature extraction is an essential and important step for speaker recognition systems. In this paper, we propose to improve these systems by exploiting both conventional features such as mel frequency cepstral coding (MFCC), linear predictive cepstral coding (LPCC) and non-conventional ones. The method exploits information present in the linear predictive (LP) residual signal. The features extracted from the LP-residue are then combined to the MFCC or the LPCC. We investigate two approaches termed as temporal and frequential representations. The first one consists of an auto-regressive (AR) modelling of the signal followed by a cepstral transformation in a similar way to the LPC-LPCC transformation. In order to take into account the non-linear nature of the speech signals we used two estimation methods based on second and third-order statistics. They are, respectively, termed as R-SOS-LPCC (residual plus secondorder statistic based estimation of the AR model plus cepstral transformation) and R-HOS-LPCC (higher order). Concerning the frequential approach, we exploit a filter bank method called the power difference of spectra in sub-band (PDSS) which measures the spectral flatness over the sub-bands. The resulting features are named R-PDSS. The analysis of these proposed schemes are done over a speaker identification problem with two different databases. The first one is the Gaudi database and contains 49 speakers. The main interest lies in the controlled acquisition conditions: mismatch between the microphones and the interval sessions. The second database is the well-known NTIMIT corpus with 630 speakers. The performances of the features are confirmed over this larger corpus. In addition, we propose to compare traditional features and residual ones by the fusion of recognizers (feature extractor + classifier). The results show that residual features carry speaker-dependent features and the combination with the LPCC or the MFCC shows global improvements in terms of robustness under different mismatches. A comparison between the residual features under the opinion fusion framework gives us useful information about the potential of both temporal and frequential representations.
Lecture Notes in Computer Science, 2015
Lecture Notes in Computer Science, 2015
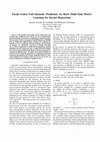
2015 11th IEEE International Conference and Workshops on Automatic Face and Gesture Recognition (FG), 2015
The problem of learning several related tasks has recently been addressed with success by the so-... more The problem of learning several related tasks has recently been addressed with success by the so-called multitask formulation, that discovers underlying common structure between tasks. Metric Learning for Kernel Regression (MLKR) aims at finding the optimal linear subspace for reducing the squared error of a Nadaraya-Watson estimator. In this paper, we propose two Multi-Task extensions of MLKR. The first one is a direct application of multi-task formulation to MLKR algorithm and the second one, the so-called Hard-MT-MLKR, lets us learn same-complexity predictors with fewer parameters, reducing overfitting issues. We apply the proposed method to Action Unit (AU) intensity prediction as a response to the Facial Expression Recognition and Analysis challenge (FERA'15). Our system improves the baseline results on the test set by 24% in terms of Intraclass Correlation Coefficient (ICC).
2015 11th IEEE International Conference and Workshops on Automatic Face and Gesture Recognition (FG), 2015
This paper introduces behavioural features for automatic stress detection, and a person-specific ... more This paper introduces behavioural features for automatic stress detection, and a person-specific normalization to enhance the performance of our system. The presented features are all visual cues automatically extracted using video processing and depth data. In order to collect the necessary data, we conducted a lab study for stress elicitation using a time constrained arithmetic mental test. Then, we propose a set of body language features for stress detection. Experimental results using a SVM show that our model can detect stress with high accuracy (77%). Moreover, person specific normalization significantly improves classification results (from 67% to 77%). Also, the performance of each of the presented features is discussed.
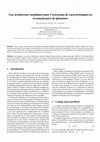
Dans ce papier, nous présentons une architecture appelée Modular Neural Predictive Coding (MNPC).... more Dans ce papier, nous présentons une architecture appelée Modular Neural Predictive Coding (MNPC). Elle est utilisée pour l'extraction de caractéristiques discriminantes. Cette architecture est conçue à l'aide de connaissances phonétiques. On estime les performances de cette architecture sur une tâche de reconnaissance de phonèmes extraits de la base Darpa-Timit. Une comparaison avec les méthodes de codage (LPC, MFCC et PLP) montrent une nette amélioration du taux de reconnaissance. Abstract -In this paper, we present an architecture called the Modular Neural Predictive Coding Architecture (MNPC). The Modular NPC is used for Discriminative Feature Extraction (DFE). It provides an architecture based on phonetics knowledge applied to phoneme recognition. The phonemes are extracted from the Darpa-Timit speech database. Comparisons with coding methods (LPC, MFCC, PLP) are presented: they put in obviousness an improvement of the recognition rates.
2015 11th IEEE International Conference and Workshops on Automatic Face and Gesture Recognition (FG), 2015
In this paper, we consider engagement in the context of Human-Robot Interaction (HRI). Previous s... more In this paper, we consider engagement in the context of Human-Robot Interaction (HRI). Previous studies in HRI relate engagement to emotion and attention independently from the context. We propose a model of engagement in Human-Robot Interaction depending on the context in which human and robot act. In our model, the mental and emotional states of the user related to engagement vary during the interaction according to the current context. Knowing the context of the interaction, the robot would know what to expect regarding the mental and the emotional state of the user and thus if it perceives a state that is not in accordance with its expectations, this might signal disengagement.
Uploads
Papers by Mohamed Chetouani