Papers by abbas Al-khudafi
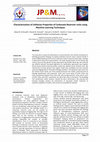
Journal of Petroleum and Mining Engineering, Mar 1, 2024
This study aims to assess the effectiveness of several decision tree machine techniques for ident... more This study aims to assess the effectiveness of several decision tree machine techniques for identifying formation lithology of complex carbonate reservoir rocks in Gamal oil field. A total of 20966 log data points from four wells were used to create the study's data. Lithology is determined using seven log parameters. The seven log parameters are the density log, neutron log, sonic log, gamma ray log, deep lateral log, shallow lateral log, and resistivity log. Different decision tree-based algorithms for classification approaches were applied. Several typical machine learning models, namely the, Random Forest. Random trees, J48, reduced-error pruning decision trees, logistic model trees, Hoeffding Tree were assessed using well logging data for formation lithology prediction. The obtained results show that the random forest model, out of the proposed decision tree models, performed best at lithology identification, with precession, recall, and F-score values of 0.913, 0.914, and 0.913, respectively. Random trees came next. with average precision, recall, and F1-score of 0.837, 0.84, and 0.837, respectively, the J48 model came in third place. The Hoeffding Tree classification model, however, showed the worst performance. We conclude that boosting strategies enhance the performance of tree-based models. Evaluation of prediction capability of models is also carried out using different datasets.

Earth Science Informatics, Dec 7, 2023
The estimation of a gas viscosity experimentally is often difficult. So, accurate determination o... more The estimation of a gas viscosity experimentally is often difficult. So, accurate determination of gas viscosity has been the main challenge in a gas reservoir development. There are many correlations to estimate this property. Often time, the results of these correlations do not agree with experimental data, thereby causing a considerable amount of error. The difficulty of these correlations can be propagated simply by tuning against some experimental data using artificial intelligent model. Currently, the achievements of artificial neural networks (ANN) techniques alone to predict gas viscosity open the door to use the hybrid system. In this model, the Particle Swarm Optimization (PSO) algorithm is employed to search for optimal connection weighs and thresholds for the neural networks (NN), then the back-propagation learning rule and training algorithm is used to adjust the final weights. A total of about 868 data points obtained from the laboratory measurements of gas viscosity were used. The data include measured gas viscosity, specific gas gravity, temperature, pressure, molecular weight, pseudo-critical temperature and pressure and non-hydrocarbon components (H2S, CO2, and N2). The performance of the PSONN model is compared with performance of ANN and other empirical model to show the most general and accurate model for predicting gas viscosity. From the results of this study, we found that the PSONN model is more reliable and accurate with the absolute present relative (APRE) error and mean square error (RMS) of 2.76 and 5.49 respectively.

AIP Conference Proceedings
Good estimation of gas compressibility factor (z-factor) of gas is an essential key in numerous g... more Good estimation of gas compressibility factor (z-factor) of gas is an essential key in numerous gas and oil calculations. In absence of data, the iterative methods were used to estimate z-factor. However, the accuracy of these correlations has become insufficient for estimating z-factor. Therefore, the objective of this study is to test the capability of various Fuzzy Logic (FL) model to predict z-factor in more accurate and precise. The FL model has three types: Fuzzy c-means (FCM), grid partition (GP), and sub-clustering (SC) Algorithms. These proposed FL models were compared with iterative methods to determine the best approach with the higher performance and reliability to predict z-factor. Around 6500 published and unpublished data points with a wide range of z-factor and reduced temperature and pressure were collected from several oil fields in the Middle East. The results display that the developed FL with various cluster techniques is more precise and trust than iterative methods and can be used in wide range of pseudoreduced pressure and temperature. The obtained results show that the FL with sub-cluster technique performs well with lower average relative percent error of 0.13% and higher accuracy (R2=1) than the others models.
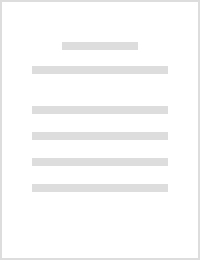
Archives of Advanced Engineering Science
Refinery off-gas is one of the major causes of air pollution, where its reuse, through channeling... more Refinery off-gas is one of the major causes of air pollution, where its reuse, through channeling into other plant setups to recover some of its constituent gases (via a steam reforming technique), is described as one of its best handling measures. A nominal off-gas containing 2.898% hydrogen (H2), 83.794% methane, 1.505% carbon dioxide, 1.103% nitrogen, 10.6% sulfur and 0.1% argon was fed to a plant to produce 37.52 kg of H2. To lift the performance of the multiple interconnected process units enhancing the generation of H2, temperature and pressure in the reactors were manipulated against the heat duty and H2 yield, where it was found that the two dependent variables are sensitive to changes made during Aspen Plus sensitivity analysis. Replacing some process units also shows significant improvement in the recovery of H2 gas. Adequate control of the synthesis process as exemplified in this work, will lead to smooth realization of the target end-product which is of high economic val...
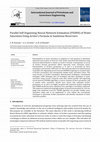
2023 International Conference on IT Innovation and Knowledge Discovery (ITIKD), 2022
Abstract
Determination of water saturation in sandstone is vital question to determine initial o... more Abstract
Determination of water saturation in sandstone is vital question to determine initial oil or gas in place in reservoir rocks. Water saturation determination using electrical measurements is mainly on Archie’s formula. Consequently, accuracy of Archie’s formula parameters affects rigorously water saturation values. Determination of Archie’s parameters a, m and n is proceeded by three techniques conventional, CAPE and 3-D. This work introduces the hybrid system of parallel self-organizing neural network (PSONN) targeting an accepted value of Archie’s parameters and consequently reliable water saturation values. This work focuses on Archie’s parameters determination techniques; conventional technique, CAPE technique and 3-D technique and then calculation of water saturation using current. Using the same data, hybrid parallel self-organizing neural network (PSONN) algorithm is used to estimate Archie’s parameters and to predict water saturation. Results have shown that estimated Arche’s parameters m, an and n are highly accepted with statistical analysis indicating that PSONN model has lower statistical error and higher correlation coefficient. This study was conducted using high number of measurement points for 144 core plugs from sandstone reservoir. PSONN algorithm can provide reliable water saturation values and it can supplement or even replace the conventional techniques to determine Archie’s parameters and thereby calculation of water saturation profiles.
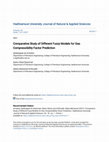
Good estimation of gas compressibility factor (z-factor) of gas is an essential key in numerous g... more Good estimation of gas compressibility factor (z-factor) of gas is an essential key in numerous gas and oil calculations. In the absence of experimental data, the iterative methods were run to estimate the z-factor. However, these methods are more complex and have a large number of factors, which require longer calculations. In addition, the accuracy of these correlations has become insufficient for the best estimations due to their limitations. The objective of this study is to test various Fuzzy Logic (FL) technique to develop a simple and robust approach. The FL has three types: Fuzzy c-means (FCM), grid partition (GP), and sub-clustering (SC) Algorithms. The proposed FL models were compared with iterative methods to test its performance and reliability to predict z-factor. Around 6500 published and unpublished data points with a wide range of z-factor and reduced temperature and pressure were collected from several fields in the Middle East used to develop FL models. It was foun...
Proceedings of the 2021 International Petroleum and Petrochemical Technology Conference, 2022
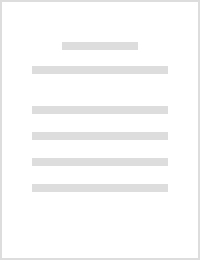
In this study, the laboratory data for different Yemeni crude oil sample are used to test accurac... more In this study, the laboratory data for different Yemeni crude oil sample are used to test accuracy of empirical correlations used for predicting crude oil equilibrium ratio. Statistical techniques were used to evaluate these correlations. Statistical comparison of currently published correlations shows that absolute relative error range between 548% and 748.97% An improved correlation for Yemeni crude oil is developed for predicting K-values. This correlation was obtained by multiple-linear regression analysis. The new K-factors correlation was found to be an improvement over most published correlation. Аннотация. В данной статье приводятся лабораторные данные для различных образцов Йеменской сырой нефти, которые используются для тестирования точности эмпирических связей, необходимых для прогнозирования коэффициента равновесия сырой нефти. Статистические методы были использованы для оценки этих связей. Статистическое сравнение представленных на данный момент связей показывает, что а...
International Journal of Production Economics, 2013
The performance of alternative forecasting methods that use hierarchical and direct forecasting s... more The performance of alternative forecasting methods that use hierarchical and direct forecasting strategies for predicting spare parts demand depends on the demand features. This paper uses data obtained from the South Korean Navy to identify the demand features of the spare parts that influence on the relative performance of the alternative forecasting methods. A logistic regression classification model for predicting the relative performance of the alternative forecasting methods for the spare parts demand by multivariate demand features was developed. This classification model minimised forecasting errors and inventory costs.

International Journal of Innovation and Applied Studies, Aug 2, 2014
Fluid classification is a critical factor in decision of reservoir and production problems. Reser... more Fluid classification is a critical factor in decision of reservoir and production problems. Reservoir fluid can be classified into five types according to laboratory and production data as black oil, volatile oil, gas condensate, wet gas and dry gas. In this work a novel application of Neural Networks (ANN) is presented. Based on production and laboratory data neural networks model is developed for automatic classification of reservoir FLUID. More than 450 samples of five types of reservoir fluids are used to develop the neural network model. About 70 % of data are accepted for neural network training, 15 % for validation and 15 % are used as test set. The importance of different input fluid properties in classification was studied. The different types of architectures for different groups of input data were tested to select the optimal neural network architecture by fitness criteria. The optimized neural network model was capable of classifying the reservoir fluids with high accuracy. The performance of ANNs models was determined by classification quality index and network error. The model has been applied successfully to classification of Yemeni fluids using different range of parameters. The results show that the proposed novel ANN model can achieve high accuracy.

University of Aden Journal of Natural and Applied Sciences
Reservoir description is a process of describing various reservoir characteristics using all the ... more Reservoir description is a process of describing various reservoir characteristics using all the available data. The nature of the description of reservoir properties is related to the availability of sample data and geologic complexity of reservoir. Reservoir characterization is needed for effective reservoir management studies. Reservoir rock properties can be estimated by several methods. Rock properties are determined by performing laboratory analyses on cores extracted from the reservoir. However, obtaining the properties from core analysis or well logging is time consuming and an expensive operation. For that, in this work, new models for estimating rock properties (porosity, permeability) are developed by adapting Artificial Neural network model (ANN). Models were successfully demonstrated for predicting reservoir rock properties (porosity and permeability) forBiyad formation of Kharir oil field. The models were tested against properties yielded from core laboratories using s...
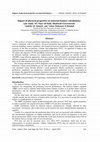
University of Aden Journal of Natural and Applied Sciences, 2022
The accuracy of many petroleum engineering calculations (e.g., material balance calculation... more The accuracy of many petroleum engineering calculations (e.g., material balance calculations, reserve estimation, well test analysis, advanced production data analysis, nodal analysis, surface network modeling, surface separation, and numerical reservoir simulations) largely depends on the accuracy of the data on pressure, volume, and temperature (PVT). An approach to improve the material balance calculation method is presented in this study. To achieve the objective, PVTP and MBAL Software programs were applied. PVT model was built by different methods. Software was implemented to calculate stock-tank oil, initially in place (STOIIP), using different approaches. Sensitive analysis was performed to investigate the effect of different parameters on material balance calculation. Illustration of the proposed approach was done on the data obtained from AL-Nasr oil field. The Average absolute error of PVT properties such as bubble-point pressure, relative volume, oil formati...
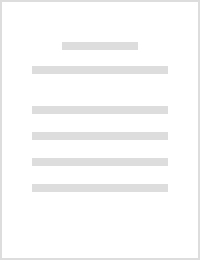
2019 First International Conference of Intelligent Computing and Engineering (ICOICE), 2019
Accurate prediction of choke size is important for successful production design and flow rate est... more Accurate prediction of choke size is important for successful production design and flow rate estimation. Many empirical correlations have been used to estimate choke size. The accuracy of these correlations has become inadequate for the best estimation. Recent achievements of Artificial Intelligence (AI) in petroleum engineering applications alone encourage the scientists to apply the hybrid model in order to improve AI results. In this study, a Particle Swarm Optimization (PSO) algorithm was developed to optimize neural network (NN) weights in order to improve their performance (PSONN). The Hybrid PSONN model compared with existed Fuzzy logic (FL) model which considered as the best AI model, Khamis [15]. Around 2445 and 766 data sets of the volatile and black oil reservoir from Middle East region respectively were selected. The comparative results confirmed that the hybrid model PSONN is performed better with lower relative errors and higher accuracy than the FL model. Based upon the results, we conclude that the hybrid models show a robust capability for the estimation of choke sizes that will help to flow rate estimation and choke design purposes. In future, the PSONN model can be combined with any simulator to improve the accuracy of oil flow rate and production design calculation.

Predicting the relationship between the flow rate and the pressure drop performance in the reserv... more Predicting the relationship between the flow rate and the pressure drop performance in the reservoir is very important for continuous production optimization in the field. The inflow performance relationship (IPR) describes the relationship between the flow rate of the well (q) and the following pressure of that well (P wf). Different inflow performance relationship correlations exist today in the petroleum industry with the most commonly used models are that of Vogel, Wiggins, Klins and Majher, Fetkovich and Sukarno and Wisnogroho. The goal of this work is to develop a new model to predict the inflow © Electronic scientific journal "Oil and Gas Business". 2019. №2 http://ogbus.ru Для сравнения используются средние процентные абсолютные погрешности между фактическими данными расхода и вычисленными данными расхода новой модели, предложенной в этой работе. Показано, что новая разработанная модель характеризуется самой низкой средней абсолютной процентной погрешностью: 8,81 %, в то время как модель Фетковича имеет среднюю абсолютную погрешность, равную 11,61 %.
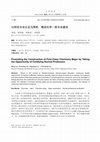
Day 2 Tue, October 19, 2021, 2021
Gas viscosity is an important physical property that controls and influences the flow of gas thro... more Gas viscosity is an important physical property that controls and influences the flow of gas through porous media and pipe networks. An accurate gas viscosity model is essential for use with reservoir and process simulators. The objective of this study is to assess the predictability of gas viscosity of Yemeni gas fields using machine learning techniques. Performance of some machine learning techniques in the prediction of gas viscosity investigated in this work. The techniques include K-nearest neighbors (KNN), Random Forest (RF), Multiple Linear Regression (MLR), and Decision Tree (DT). About 440 data points were collected from different Yemeni gas fields were used to develop the machine-learning model. The input data used in the training include pressure, temperature, gas density, specific gravity, gas formation volume factor, gas deviation factor, gas molecular weight, pseudo-reduced temperature and pressure, pseudo-critical temperature and pressure, and non-hydrocarbon gas comp...
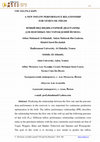
Oil and Gas Business, 2019
Predicting the relationship between the flow rate and the pressure drop performance in the reserv... more Predicting the relationship between the flow rate and the pressure drop performance in the reservoir is very important for continuous production optimization in the field. The inflow performance relationship (IPR) describes the relationship between the flow rate of the well (q) and the following pressure of that well (P wf). Different inflow performance relationship correlations exist today in the petroleum industry with the most commonly used models are that of Vogel, Wiggins, Klins and Majher, Fetkovich and Sukarno and Wisnogroho. The goal of this work is to develop a new model to predict the inflow © Electronic scientific journal "Oil and Gas Business". 2019. №2 http://ogbus.ru Для сравнения используются средние процентные абсолютные погрешности между фактическими данными расхода и вычисленными данными расхода новой модели, предложенной в этой работе. Показано, что новая разработанная модель характеризуется самой низкой средней абсолютной процентной погрешностью: 8,81 %, в то время как модель Фетковича имеет среднюю абсолютную погрешность, равную 11,61 %.
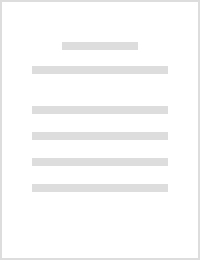
International Journal of Innovation and Applied Studies, 2013
Equilibrium constant has many applications in solving problems in reservoir engineering and petro... more Equilibrium constant has many applications in solving problems in reservoir engineering and petroleum processing. Various correlations are available for estimating K- values for heptanes plus fractions. These correlations can be classified into simple and complicated. However these correlations are not able to predict K values adequately for a wide range of conditions. They lose validity in specific range of pressure and temperature and exhibit some error. In this work neuro-fuzzy modeling techniques (ANFIS) is developed to predict K- values for heavy fractions. A large collection of K- values data points (more than 1340 data points) were extracted from experimental 570 PVT reports using the principal of material balance are used in developing the neuro- fuzzy model. 80% of the data points were used to train ANFIS model and 20% of data sets were used to validate, and test the model. Statistical analysis (average absolute percent error, correlation coefficient, standard deviation, ma...
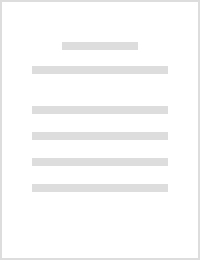
Day 2 Tue, October 19, 2021
Gas viscosity is an important physical property that controls and influences the flow of gas thro... more Gas viscosity is an important physical property that controls and influences the flow of gas through porous media and pipe networks. An accurate gas viscosity model is essential for use with reservoir and process simulators. The objective of this study is to assess the predictability of gas viscosity of Yemeni gas fields using machine learning techniques. Performance of some machine learning techniques in the prediction of gas viscosity investigated in this work. The techniques include K-nearest neighbors (KNN), Random Forest (RF), Multiple Linear Regression (MLR), and Decision Tree (DT). About 440 data points were collected from different Yemeni gas fields were used to develop the machine-learning model. The input data used in the training include pressure, temperature, gas density, specific gravity, gas formation volume factor, gas deviation factor, gas molecular weight, pseudo-reduced temperature and pressure, pseudo-critical temperature and pressure, and non-hydrocarbon gas comp...
Uploads
Papers by abbas Al-khudafi
Determination of water saturation in sandstone is vital question to determine initial oil or gas in place in reservoir rocks. Water saturation determination using electrical measurements is mainly on Archie’s formula. Consequently, accuracy of Archie’s formula parameters affects rigorously water saturation values. Determination of Archie’s parameters a, m and n is proceeded by three techniques conventional, CAPE and 3-D. This work introduces the hybrid system of parallel self-organizing neural network (PSONN) targeting an accepted value of Archie’s parameters and consequently reliable water saturation values. This work focuses on Archie’s parameters determination techniques; conventional technique, CAPE technique and 3-D technique and then calculation of water saturation using current. Using the same data, hybrid parallel self-organizing neural network (PSONN) algorithm is used to estimate Archie’s parameters and to predict water saturation. Results have shown that estimated Arche’s parameters m, an and n are highly accepted with statistical analysis indicating that PSONN model has lower statistical error and higher correlation coefficient. This study was conducted using high number of measurement points for 144 core plugs from sandstone reservoir. PSONN algorithm can provide reliable water saturation values and it can supplement or even replace the conventional techniques to determine Archie’s parameters and thereby calculation of water saturation profiles.
Determination of water saturation in sandstone is vital question to determine initial oil or gas in place in reservoir rocks. Water saturation determination using electrical measurements is mainly on Archie’s formula. Consequently, accuracy of Archie’s formula parameters affects rigorously water saturation values. Determination of Archie’s parameters a, m and n is proceeded by three techniques conventional, CAPE and 3-D. This work introduces the hybrid system of parallel self-organizing neural network (PSONN) targeting an accepted value of Archie’s parameters and consequently reliable water saturation values. This work focuses on Archie’s parameters determination techniques; conventional technique, CAPE technique and 3-D technique and then calculation of water saturation using current. Using the same data, hybrid parallel self-organizing neural network (PSONN) algorithm is used to estimate Archie’s parameters and to predict water saturation. Results have shown that estimated Arche’s parameters m, an and n are highly accepted with statistical analysis indicating that PSONN model has lower statistical error and higher correlation coefficient. This study was conducted using high number of measurement points for 144 core plugs from sandstone reservoir. PSONN algorithm can provide reliable water saturation values and it can supplement or even replace the conventional techniques to determine Archie’s parameters and thereby calculation of water saturation profiles.