SULE'S PAPERS by Zenun Kastrati
Proceedings of the 2015 IEEE 9th International Conference on Semantic Computing (IEEE ICSC 2015), 2015
Papers by Zenun Kastrati
Lecture notes in networks and systems, Dec 31, 2022
Journal of intelligent information systems, Mar 22, 2024
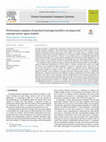
Future Generation Computer Systems, Jul 1, 2019
This paper provides a comprehensive performance analysis of parametric and non-parametric machine... more This paper provides a comprehensive performance analysis of parametric and non-parametric machine learning classifiers including a deep feed-forward multi-layer perceptron (MLP) network on two variants of improved Concept Vector Space (iCVS) model. In the first variant, a weighting scheme enhanced with the notion of concept importance is used to assess weight of ontology concepts. Concept importance shows how important a concept is in an ontology and it is automatically computed by converting the ontology into a graph and then applying one of the Markov based algorithms. In the second variant of iCVS, concepts provided by the ontology and their semantically related terms are used to construct concept vectors in order to represent the document into a semantic vector space. We conducted various experiments using a variety of machine learning classifiers for three different models of document representation. The first model is a baseline concept vector space (CVS) model that relies on an exact/partial match technique to represent a document into a vector space. The second and third model is an iCVS model that employs an enhanced concept weighting scheme for assessing weights of concepts (variant 1), and the acquisition of terms that are semantically related to concepts of the ontology for semantic document representation (variant 2), respectively. Additionally, a comparison between seven different classifiers is performed for all three models using precision, recall, and F1 score. Results for multiple configurations of deep learning architecture are obtained by varying the number of hidden layers and nodes in each layer, and are compared to those obtained with conventional classifiers. The obtained results show that the classification performance is highly dependent upon the choice of a classifier, and that the Random Forest, Gradient Boosting, and Multilayer Perceptron are among the classifiers that performed rather well for all three models.
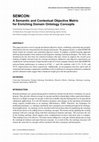
International Journal on Semantic Web and Information Systems, Apr 1, 2016
This paper presents a novel concept enrichment objective metric combining contextual and semantic... more This paper presents a novel concept enrichment objective metric combining contextual and semantic information of terms extracted from the domain documents. The proposed metric is called SEMCON which stands for semantic and contextual objective metric. It employs a hybrid learning approach utilizing functionalities from statistical and linguistic ontology learning techniques. The metric also introduced for the first time two statistical features that have shown to improve the overall score ranking of highly relevant terms for concept enrichment. Subjective and objective experiments are conducted in various domains. Experimental results (F1) from computer domain show that SEMCON achieved better performance in contrast to tf*idf, χ 2 and LSA methods, with 12.2%, 21.8%, and 24.5% improvement over them respectively. Additionally, an investigation into how much each of contextual and semantic components contributes to the overall task of concept enrichment is conducted and the obtained results suggest that a balanced weight gives the best performance.
IEEE Access
Internasjonalisering og Kvalitetsutvikling i høyere utdanning (DIKU) through the Curricula Develo... more Internasjonalisering og Kvalitetsutvikling i høyere utdanning (DIKU) through the Curricula Development and Capacity Building in Applied Computer Science for Pakistani Higher Education Institutions (CONNECT) Project under Grant NORPART-2021/10502.
Lecture Notes in Computer Science, 2022
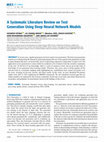
IEEE Access
In recent years, significant progress has been made in text generation. The latest text generatio... more In recent years, significant progress has been made in text generation. The latest text generation models are revolutionizing the domain by generating human-like text. It has gained wide popularity recently in many domains like news, social networks, movie scriptwriting, and poetry composition, to name a few. The application of text generation in various fields has resulted in a lot of interest from the scientific community in this area. To the best of our knowledge, there is a lack of extensive review and an up-to-date body of knowledge of text generation deep learning models. Therefore, this survey aims to bring together all the relevant work in a systematic mapping study highlighting key contributions from various researchers over the years, focusing on the past, present, and future trends. In this work, we have identified 90 primary studies from 2015 to 2021 employing the PRISMA framework. We also identified research gaps that are further needed to be explored by the research community. In the end, we provide some future directions for researchers and guidelines for practitioners based on the findings of this review.
Engineering Applications of Artificial Intelligence
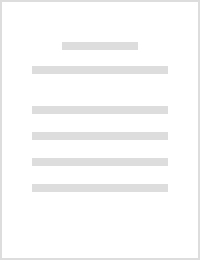
PLOS ONE
Low-resource languages are gaining much-needed attention with the advent of deep learning models ... more Low-resource languages are gaining much-needed attention with the advent of deep learning models and pre-trained word embedding. Though spoken by more than 230 million people worldwide, Urdu is one such low-resource language that has recently gained popularity online and is attracting a lot of attention and support from the research community. One challenge faced by such resource-constrained languages is the scarcity of publicly available large-scale datasets for conducting any meaningful study. In this paper, we address this challenge by collecting the first-ever large-scale Urdu Tweet Dataset for sentiment analysis and emotion recognition. The dataset consists of a staggering number of 1, 140, 821 tweets in the Urdu language. Obviously, manual labeling of such a large number of tweets would have been tedious, error-prone, and humanly impossible; therefore, the paper also proposes a weakly supervised approach to label tweets automatically. Emoticons used within the tweets, in addit...
Egyptian Informatics Journal
2023 4th International Conference on Computing, Mathematics and Engineering Technologies (iCoMET)
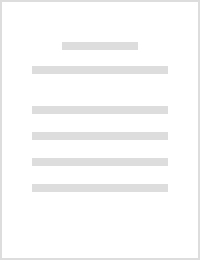
Proceedings of the 2019 5th International Conference on Computing and Artificial Intelligence
This paper proposes to use acoustic features employing deep neural network (DNN) and convolutiona... more This paper proposes to use acoustic features employing deep neural network (DNN) and convolutional neural network (CNN) models for classifying video lectures in a massive open online course (MOOC). The models exploit the voice pattern of the lecturer for identification and for classifying the video lecture according to the right speaker category. Filter bank and Mel frequency cepstral coefficient (MFCC) feature along with first and second order derivatives (Δ/ΔΔ) are used as input features to the proposed models. These features are extracted from the speech signal which is obtained from the video lectures by separating the audio from the video using FFmpeg. The deep learning models are evaluated using precision, recall, and F1 score and the obtained accuracy is compared for both acoustic features with traditional machine learning classifiers for speaker identification. A significant improvement of 3% to 7% classification accuracy is achieved over the DNN and twice to that of shallow machine learning classifiers for 2D-CNN with MFCC. The proposed 2D-CNN model with an F1 score of 85.71% for text-independent speaker identification makes it plausible to use speaker ID as a classification approach for organizing video lectures automatically in a MOOC setting.
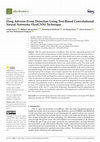
Electronics
With the rapid advancement in healthcare, there has been exponential growth in the healthcare rec... more With the rapid advancement in healthcare, there has been exponential growth in the healthcare records stored in large databases to help researchers, clinicians, and medical practitioner’s for optimal patient care, research, and trials. Since these studies and records are lengthy and time consuming for clinicians and medical practitioners, there is a demand for new, fast, and intelligent medical information retrieval methods. The present study is a part of the project which aims to design an intelligent medical information retrieval and summarization system. The whole system comprises three main modules, namely adverse drug event classification (ADEC), medical named entity recognition (MNER), and multi-model text summarization (MMTS). In the current study, we are presenting the design of the ADEC module for classification tasks, where basic machine learning (ML) and deep learning (DL) techniques, such as logistic regression (LR), decision tree (DT), and text-based convolutional neura...
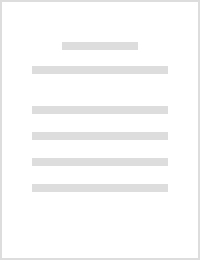
2021 19th International Conference on Information Technology Based Higher Education and Training (ITHET)
Many higher education institutions in the world have switched to online learning due to the ongoi... more Many higher education institutions in the world have switched to online learning due to the ongoing COVID-19 pandemic, which also has greatly contributed towards an increase of MOOCs enrollments across various disciplines. There are many factors that can influence the learning trajectory in MOOCs settings, and in order to gain a deeper understanding of learners' experience, we employ a quantitative research method, in which sentiment analysis and topic modeling are applied. In this perspective, learners' reviews from the learning platform Coursera are examined to identify the main topics associated with the course and the learners' attitudes and opinions towards these topics. For this purpose, a total of 28,281 reviews scraped from five courses within the field of data science are analyzed, and consequently nine course topics for which learners have commented on are found. The identified topics include: content, delivery, assessment, learning experience, tools, video material, teaching style, instructor skills and course provider. Next, each topic is assigned a sentiment score using a lexicon-based approach, and the topics which mostly affect the learning experience are finally determined and discussed.
Egyptian Informatics Journal
Uploads
SULE'S PAPERS by Zenun Kastrati
Papers by Zenun Kastrati