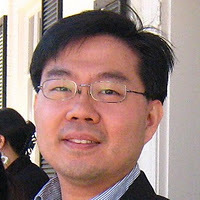
Francis Huang
Related Authors
Arnab Bhattacharya
IIT Kanpur
E. Wayne Ross
University of British Columbia
Jo Tondeur
Vrije Universiteit Brussel
Gregory Sadler
Milwaukee Institute of Art and Design
Daniel Cassany
Pompeu Fabra University
David Seamon
Kansas State University
Lisa Yamagata-Lynch
University of Tennessee Knoxville
Willeke Wendrich
University of California, Los Angeles
Carlo Bianchini
University of Pavia
Stéphane Moyson
Université catholique de Louvain
Uploads
Papers by Francis Huang
Findings indicate a downward trend in redshirting rates (3.5% in fall of 2012), and redshirted students were consistently more
likely to be White boys who were not economically disadvantaged. Students with disabilities were also more likely to be redshirted.
Of the redshirted students, the majority were born in the summer months (>70%). Rates have been stable and lower
than previously reported national estimates, suggesting that the practice is not as widespread as feared.
can yield consistent and unbiased estimates and standard errors (given the apropriate conditions), and can be performed using a variety of commercially- and freely-available statistical software. I analyze a subsample of the High School and Beyond (HSB) dataset using MLM, regression using TSL, and ordinary least squares regression and compare results. In addition, 12,000 random samples are drawn from the HSB dataset of varying level-one and level-two sample sizes in order to compute biases in standard errors based on the different conditions. Sample R and SAS syntax showing how
to run regressions using TSL are provided.
Findings indicate a downward trend in redshirting rates (3.5% in fall of 2012), and redshirted students were consistently more
likely to be White boys who were not economically disadvantaged. Students with disabilities were also more likely to be redshirted.
Of the redshirted students, the majority were born in the summer months (>70%). Rates have been stable and lower
than previously reported national estimates, suggesting that the practice is not as widespread as feared.
can yield consistent and unbiased estimates and standard errors (given the apropriate conditions), and can be performed using a variety of commercially- and freely-available statistical software. I analyze a subsample of the High School and Beyond (HSB) dataset using MLM, regression using TSL, and ordinary least squares regression and compare results. In addition, 12,000 random samples are drawn from the HSB dataset of varying level-one and level-two sample sizes in order to compute biases in standard errors based on the different conditions. Sample R and SAS syntax showing how
to run regressions using TSL are provided.