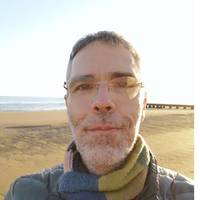
Fabio Cuzzolin
Fabio Cuzzolin was born in Jesolo, Italy.
He graduated magna cum laude with the University of Padua, was awarded a Ph.D. there in 2001 for a thesis entitled “Visions of a generalized probability theory", and worked in world-class institutions such as the Washington University in St. Louis, Politecnico di Milano, the University of California at Los Angeles. In 2006 he was awarded a Marie Curie Fellowship with INRIA Rhone-Alpes, France. In 2007 he classified second there in the Senior Researcher national recruitment.
He has been at Brookes since 2008, took up a Senior Lectureship there in July'11, and a Readership in October 2011.
He has been nominated Subject Coordinator for the new Master's course in Computer Vision which will be launched by the Department in September 2013.
He has taken on the role of Head of the Artificial Intelligence and Vision research group in September 2012, and has been awarded in October 2012 a Next 10 award by the Faculty of Technology, Design and Environment as one of its top emerging researchers.
He is currently supervising two Ph.D. students, an EPSRC-funded postdoc, two visiting students from Turkey and Italy. Two more Ph.D. students will join his group in 2014.
Dr Cuzzolin is a world expert in uncertainty theory and belief functions theory. He worked extensively on the mathematical foundations of belief calculus. His main contribution is his geometric approach to uncertainty measures, in which uncertainty measures are represented as points of a Cartesian space and there analyzed. His work on the field is in the process of being published in two separate monographs published by Springer-Verlag (“The geometry of uncertainty”) and Lambert Academic Publishing ( "Visions of a generalized probability theory").
He is also well known for his work in computer vision, mainly machine learning for human motion analysis, including tensorial models for identity recognition, metric learning for action recognition, and spectral techniques for articulated object segmentation and matching.
He is the author of some 90 peer-reviewed publications, published or under review, including two monographs, an edited Springer volume, 3 book chapters, 14 journals (+ 8 u/r), 9 chapters in collections.
He won awards for Best Paper at PRICAI'08, Poster Prize at the ISIPTA'11 Symposium on Imprecise Probabilities, Best Poster at the 2012 INRIA Summer School on Machine Learning and Visual Recognition, and was short-listed for prizes at the ECSQARU'11 and BMVC12 conferences, where he was given the Outstanding Reviewer Award.
Dr Cuzzolin is Associate Editor of the IEEE Transactions of Fuzzy Systems, Guest Editor for the International Journal of Approximate Reasoning, has been AE for “IEEE Transactions on Systems, Man, and Cybernetics C", Guest Editor for “Information Fusion", and collaborates as a reviewer with many other journals in both computer vision and imprecise probabilities, such as: Artificial Intelligence, the IEEE Transactions on Systems, Man, and Cybernetics - part B, the IEEE Transactions on Fuzzy Systems, Computer Vision and Image Understanding, Information Sciences, the Journal of Risk and Reliability; the International Journal on Uncertainty, Fuzziness, and Knowledge-Based Systems, Image and Vision Computing, the Annals of Operations Research.
Dr Cuzzolin has served in the TCP of around 50 international conferences, including BMVC, IPMU and SMC, and is Senior Program Committee member of Uncertainty in Artificial Intelligence (UAI).
He was the Program Chair and local organizer of the 3rd International Conference on the Theory of Belief Functions (BELIEF 2014), which was held in St. Hugh's College, Oxford, UK.
Phone: +44 (0)1865 484526
Address: Department of Computing and Communication Technologies
Faculty of Technology, Design and Environment
Oxford Brookes University
Wheatley campus, OX33 1HX, OXFORD, UK
He graduated magna cum laude with the University of Padua, was awarded a Ph.D. there in 2001 for a thesis entitled “Visions of a generalized probability theory", and worked in world-class institutions such as the Washington University in St. Louis, Politecnico di Milano, the University of California at Los Angeles. In 2006 he was awarded a Marie Curie Fellowship with INRIA Rhone-Alpes, France. In 2007 he classified second there in the Senior Researcher national recruitment.
He has been at Brookes since 2008, took up a Senior Lectureship there in July'11, and a Readership in October 2011.
He has been nominated Subject Coordinator for the new Master's course in Computer Vision which will be launched by the Department in September 2013.
He has taken on the role of Head of the Artificial Intelligence and Vision research group in September 2012, and has been awarded in October 2012 a Next 10 award by the Faculty of Technology, Design and Environment as one of its top emerging researchers.
He is currently supervising two Ph.D. students, an EPSRC-funded postdoc, two visiting students from Turkey and Italy. Two more Ph.D. students will join his group in 2014.
Dr Cuzzolin is a world expert in uncertainty theory and belief functions theory. He worked extensively on the mathematical foundations of belief calculus. His main contribution is his geometric approach to uncertainty measures, in which uncertainty measures are represented as points of a Cartesian space and there analyzed. His work on the field is in the process of being published in two separate monographs published by Springer-Verlag (“The geometry of uncertainty”) and Lambert Academic Publishing ( "Visions of a generalized probability theory").
He is also well known for his work in computer vision, mainly machine learning for human motion analysis, including tensorial models for identity recognition, metric learning for action recognition, and spectral techniques for articulated object segmentation and matching.
He is the author of some 90 peer-reviewed publications, published or under review, including two monographs, an edited Springer volume, 3 book chapters, 14 journals (+ 8 u/r), 9 chapters in collections.
He won awards for Best Paper at PRICAI'08, Poster Prize at the ISIPTA'11 Symposium on Imprecise Probabilities, Best Poster at the 2012 INRIA Summer School on Machine Learning and Visual Recognition, and was short-listed for prizes at the ECSQARU'11 and BMVC12 conferences, where he was given the Outstanding Reviewer Award.
Dr Cuzzolin is Associate Editor of the IEEE Transactions of Fuzzy Systems, Guest Editor for the International Journal of Approximate Reasoning, has been AE for “IEEE Transactions on Systems, Man, and Cybernetics C", Guest Editor for “Information Fusion", and collaborates as a reviewer with many other journals in both computer vision and imprecise probabilities, such as: Artificial Intelligence, the IEEE Transactions on Systems, Man, and Cybernetics - part B, the IEEE Transactions on Fuzzy Systems, Computer Vision and Image Understanding, Information Sciences, the Journal of Risk and Reliability; the International Journal on Uncertainty, Fuzziness, and Knowledge-Based Systems, Image and Vision Computing, the Annals of Operations Research.
Dr Cuzzolin has served in the TCP of around 50 international conferences, including BMVC, IPMU and SMC, and is Senior Program Committee member of Uncertainty in Artificial Intelligence (UAI).
He was the Program Chair and local organizer of the 3rd International Conference on the Theory of Belief Functions (BELIEF 2014), which was held in St. Hugh's College, Oxford, UK.
Phone: +44 (0)1865 484526
Address: Department of Computing and Communication Technologies
Faculty of Technology, Design and Environment
Oxford Brookes University
Wheatley campus, OX33 1HX, OXFORD, UK
less
Related Authors
Amy R. Bland
Manchester Metropolitan University
Sophia Armand
Columbia University
Nikolina Skandali
University of Cambridge
Thomas Zoëga Ramsøy
Copenhagen Business School, CBS
Alessandro Antonietti
Università Cattolica del Sacro Cuore (Catholic University of the Sacred Heart)
InterestsView All (67)
Uploads
Videos by Fabio Cuzzolin
Autonomous vehicles (AVs) employ a variety of sensors to identify roadside infrastructure and other road users, with much of the existing work focusing on scene understanding and robust object detection. Human drivers, however, approach the driving task in a more holistic fashion which entails, in particular, recognising and understanding the evolution of road events. Testing an AV’s capability to recognise the actions undertaken by other road agents is thus crucial to improve their situational awareness and facilitate decision making.
In this talk we introduce the ROad event Awareness Dataset (ROAD) for Autonomous Driving, to our knowledge the first of its kind. ROAD is explicitly designed to test the ability of an autonomous vehicle to detect road events.
The theory of belief functions, sometimes referred to as evidence theory or Dempster-Shafer theory, was first introduced by Arthur P. Dempster in the context of statistical inference, to be later developed by Glenn Shafer as a general framework for modelling epistemic uncertainty. The methodology is now well established as a general framework for reasoning with uncertainty, with well-understood connections to related frameworks such as probability, possibility, random set and imprecise probability theories.
This talk aims at bridging the gap between researchers in the field and the wider AI and Uncertainty Theory community, with the longer term goal of a more fruitful collaboration and dissemination of ideas.
Papers by Fabio Cuzzolin
Investments are a heavily regulated industry (MIFID II, UCITS IV and V, SM&CR, GDPR etc). Most regulations are intentionally technology-neutral. These regulations are legally binding (hard law). Recent years saw the emergence of regulatory and industry publications (soft laws) focusing specifically on AI. In this Article we analyse both hard law and soft law instruments.
The contributions of this work are: first, a review of key regulations applicable to AI in investment management (and oftentimes by extension to banking as well) from multiple jurisdictions; second, a framework and an analysis of key regulatory themes for AI.
Autonomous vehicles (AVs) employ a variety of sensors to identify roadside infrastructure and other road users, with much of the existing work focusing on scene understanding and robust object detection. Human drivers, however, approach the driving task in a more holistic fashion which entails, in particular, recognising and understanding the evolution of road events. Testing an AV’s capability to recognise the actions undertaken by other road agents is thus crucial to improve their situational awareness and facilitate decision making.
In this talk we introduce the ROad event Awareness Dataset (ROAD) for Autonomous Driving, to our knowledge the first of its kind. ROAD is explicitly designed to test the ability of an autonomous vehicle to detect road events.
The theory of belief functions, sometimes referred to as evidence theory or Dempster-Shafer theory, was first introduced by Arthur P. Dempster in the context of statistical inference, to be later developed by Glenn Shafer as a general framework for modelling epistemic uncertainty. The methodology is now well established as a general framework for reasoning with uncertainty, with well-understood connections to related frameworks such as probability, possibility, random set and imprecise probability theories.
This talk aims at bridging the gap between researchers in the field and the wider AI and Uncertainty Theory community, with the longer term goal of a more fruitful collaboration and dissemination of ideas.
Investments are a heavily regulated industry (MIFID II, UCITS IV and V, SM&CR, GDPR etc). Most regulations are intentionally technology-neutral. These regulations are legally binding (hard law). Recent years saw the emergence of regulatory and industry publications (soft laws) focusing specifically on AI. In this Article we analyse both hard law and soft law instruments.
The contributions of this work are: first, a review of key regulations applicable to AI in investment management (and oftentimes by extension to banking as well) from multiple jurisdictions; second, a framework and an analysis of key regulatory themes for AI.
Fabio will explain just how machines can be provided with this mind-reading ability.
The purpose of this talk, in its first part, is to understanding belief theory in the context of mathematical probability and its main interpretations, Bayesian and frequentist statistics, contrasting these three methodologies according to their treatment of uncertain data.
In the second part we recall the existing statistical views of belief function theory, due to the work by Dempster, Almond, Hummel and Landy, Zhang and Liu, Walley and Fine, among others.
Finally, we outline a research programme for the development of a fully-fledged theory of statistical inference with random sets. In particular, we discuss the notion of generalised lower and upper likelihoods, the formulation of a framework for logistic regression with belief functions, the generalisation of the classical total probability theorem to belief functions, the formulation of parametric models based of random sets, and the development of a theory of random variables and processes in which the underlying probability space is replaced by a random set space.
to the spatiotemporal localisation (detection)
and classification of multiple concurrent actions
within temporally untrimmed videos. Our
framework is composed of three stages. In stage
1, a cascade of deep region proposal and detection
networks are employed to classify regions
of each video frame potentially containing an
action of interest. In stage 2, appearance and
motion cues are combined by merging the detection
boxes and softmax classification scores
generated by the two cascades. In stage 3, sequences
of detection boxes most likely to be associated
with a single action instance, called action
tubes, are constructed by solving two optimisation
problems via dynamic programming.
The tutorial is very comprehensive (468 slides), covering:
(i) a review of mathematical probability and its interpretations (Bayesian and frequentist);
(ii) the rational for going beyond standard probability: it's all about the data!
(iii) the basis notions of the theory of belief functions;
(iv) reasoning with belief functions: inference, combination/conditioning, graphical models, decision making;
(v) using belief functions for classification, regression, estimation, etc;
(vi) dealing with computational issues and extending belief measures to real numbers;
(vii) the main frameworks derived from belief theory, and its relationship with other theories of uncertainty;
(viii) a number of example applications;
(ix) new horizons, from the formulation of limit theorems for random sets, generalising the notion of likelihood and logistic regression for rare event estimation, climatic change modelling and new foundations for machine learning based on random set theory, a geometry of uncertainty.
Tutorial slides are downloadable at http://cms.brookes.ac.uk/staff/FabioCuzzolin/files/IJCAI2016.pdf
- probabilities do not represent well ignorance and lack of data;
- evidence is normally limited, rather than infinite as assumed by (frequentist) probability;
- expert knowledge needs often to be combined with hard evidence;
- in extreme cases (rare events or far-future predictions) very little data;
- bottom line: not enough evidence to determine the actual probability describing the problem.
An early debate on the rationale of belief functions gave a strong contribution to the growth and success of the UAI community and series of conference in the Eighties and Nineties, thanks to the contribution of scientists of the caliber of Glenn Shafer, Judea Pearl, Philippe Smets and Prakash Shenoy, among others. Ever since the UAI and BELIEF community have somewhat diverged, and the proposers’ effort has been recently directed towards going back to a closer relationships and exchange of ideas between the two communities. This was one of the aims of the recent BELIEF 2014 International Conference of which the proposers were General Chair and member of the Steering Committee, respectively. A number of books are being published on the subject as we speak, and the impact of the belief function approach to uncertainty is growing.
The tutorial aims at bridging the gap between researchers in the field and the wider AI and Uncertainty Theory community, with the longer term goal of a more fruitful collaboration and dissemination of ideas.
In the chapters in Part I, Theories of Uncertainty, the author offers an extensive recapitulation of the state of the art in the mathematics of uncertainty. This part of the book contains the most comprehensive summary to date of the whole of belief theory, with Chap. 4 outlining for the first time, and in a logical order, all the steps of the reasoning chain associated with modelling uncertainty using belief functions, in an attempt to provide a self-contained manual for the working scientist. In addition, the book proposes in Chap. 5 what is possibly the most detailed compendium available of all theories of uncertainty. Part II, The Geometry of Uncertainty, is the core of this book, as it introduces the author’s own geometric approach to uncertainty theory, starting with the geometry of belief functions: Chap. 7 studies the geometry of the space of belief functions, or belief space, both in terms of a simplex and in terms of its recursive bundle structure; Chap. 8 extends the analysis to Dempster’s rule of combination, introducing the notion of a conditional subspace and outlining a simple geometric construction for Dempster’s sum; Chap. 9 delves into the combinatorial properties of plausibility and commonality functions, as equivalent representations of the evidence carried by a belief function; then Chap. 10 starts extending the applicability of the geometric approach to other uncertainty measures, focusing in particular on possibility measures (consonant belief functions) and the related notion of a consistent belief function. The chapters in Part III, Geometric Interplays, are concerned with the interplay of uncertainty measures of different kinds, and the geometry of their relationship, with a particular focus on the approximation problem. Part IV, Geometric Reasoning, examines the application of the geometric approach to the various elements of the reasoning chain illustrated in Chap. 4, in particular conditioning and decision making. Part V concludes the book by outlining a future, complete statistical theory of random sets, future extensions of the geometric approach, and identifying high-impact applications to climate change, machine learning and artificial intelligence.
The book is suitable for researchers in artificial intelligence, statistics, and applied science engaged with theories of uncertainty. The book is supported with the most comprehensive bibliography on belief and uncertainty theory.
visual skills humans and animals are provided by Nature, allowing them to interact effortlessly
with complex, dynamic environments. Designing automated visual recognition and sensing systems
typically involves tackling a number of challenging tasks, and requires an impressive variety of sophisticated
mathematical tools. In most cases, the knowledge a machine has of its surroundings is at best
incomplete – missing data is a common problem, and visual cues are affected by imprecision. The need
for a coherent mathematical ‘language’ for the description of uncertain models and measurements then
naturally arises from the solution of computer vision problems.
The theory of evidence (sometimes referred to as ‘evidential reasoning’, ‘belief theory’ or ‘Dempster-
Shafer theory’) is, perhaps, one of the most successful approaches to uncertainty modelling, as arguably
the most straightforward and intuitive approaches to a generalized probability theory. Emerging in the
last Sixties from a profound criticism of the more classical Bayesian theory of inference and modelling
of uncertainty, it stimulated in the last decades an extensive discussion of the epistemic nature of both
subjective ‘degrees of beliefs’ and frequentist ‘chances’ or relative frequencies. More recently, a renewed
interest in belief functions, the mathematical generalization of probabilities which are the object of study
of the theory of evidence, has seen a blossoming of applications to a variety of fields of applied science.
In this Book we are going to show how, indeed, the fruitful interaction of computer vision and evidential
reasoning is able stimulate a number of advances in both fields. From a methodological point of
view, novel theoretical advances concerning the geometric and algebraic properties of belief functions as
mathematical objects will be illustrated in some detail in Part II, with a focus on a perspective ‘geometric
approach’ to uncertainty and an algebraic solution of the issue of conflicting evidence. In Part III we will
illustrate how these new perspectives on the theory of belief functions arise from important computer vision
problems, such as articulated object tracking, data association and object pose estimation, to which
in turn the evidential formalism can give interesting new solutions. Finally, some initial steps towards
a generalization of the notion of total probability to belief functions will be taken, in the perspective of
endowing the theory of evidence with a complete battery of estimation and inference tools to the benefit
of scientists and practitioners.
This short talk abstracted from an upcoming half-day tutorial at IJCAI 2016 is designed to introduce to non-experts the principles and rationale of random sets and belief function theory, review its rationale in the context of frequentist and Bayesian interpretations of probability but also in relationship with the other main approaches to non-additive probability, survey the key elements of the methodology and the most recent developments, discuss current trends in both its theory and applications. Finally, a research program for the future is outlined, which include a robustification of Vapnik' statistical learning theory for an Artificial Intelligence 'in the wild'.
make detecting smaller objects (that is, objects that occupy a small pixel area in the input image) a truly challenging task for machines and a wide open research field.
This study explores ways in which the popular YOLOv5 object detector can be modified to improve its performance in detecting smaller objects, with a particular focus on its application to autonomous racing. To achieve this, we investigate how replacing certain structural elements of the
model (as well as their connections and other parameters) can affect performance and inference time. In doing so, we propose a series of models at different scales, which we name ‘YOLO-Z’, and which display an improvement of up to 6.9% in mAP when detecting smaller objects at 50% IOU, at a cost of just a 3ms increase in inference time compared to the original YOLOv5.
Our objective is not only to inform future research on the potential of adjusting a popular detector such as YOLOv5 to address specific tasks, but also to provide insights on how specific changes can impact small object detection. Such findings, applied to the wider context of autonomous vehicles, could increase the amount of contextual information available to such systems.