Papers by Panagiotis E Pintelas

Journal of Imaging
With the proliferation of image-based applications in various domains, the need for accurate and ... more With the proliferation of image-based applications in various domains, the need for accurate and interpretable image similarity measures has become increasingly critical. Existing image similarity models often lack transparency, making it challenging to understand the reasons why two images are considered similar. In this paper, we propose the concept of explainable image similarity, where the goal is the development of an approach, which is capable of providing similarity scores along with visual factual and counterfactual explanations. Along this line, we present a new framework, which integrates Siamese Networks and Grad-CAM for providing explainable image similarity and discuss the potential benefits and challenges of adopting this approach. In addition, we provide a comprehensive discussion about factual and counterfactual explanations provided by the proposed framework for assisting decision making. The proposed approach has the potential to enhance the interpretability, trust...

Electronics
Explainable machine learning is an emerging new domain fundamental for trustworthy real-world app... more Explainable machine learning is an emerging new domain fundamental for trustworthy real-world applications. A lack of trust and understanding are the main drawbacks of deep learning models when applied to real-world decision systems and prediction tasks. Such models are considered as black boxes because they are unable to explain the reasons for their predictions in human terms; thus, they cannot be universally trusted. In critical real-world applications, such as in medical, legal, and financial ones, an explanation of machine learning (ML) model decisions is considered crucially significant and mandatory in order to acquire trust and avoid fatal ML bugs, which could disturb human safety, rights, and health. Nevertheless, explainable models are more than often less accurate; thus, it is essential to invent new methodologies for creating interpretable predictors that are almost as accurate as black-box ones. In this work, we propose a novel explainable feature extraction and predict...
Algorithms
This article will summarize the works published in a Special Issue of Algorithms, entitled “Ensem... more This article will summarize the works published in a Special Issue of Algorithms, entitled “Ensemble Learning and/or Explainability”(https://www [...]
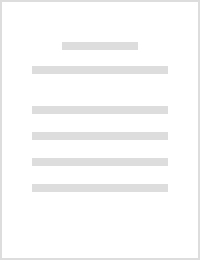
Virtual Reality (VR) technology has already entered into the area of the educational software and... more Virtual Reality (VR) technology has already entered into the area of the educational software and delivers systems where the trainees can use interactive virtual microworlds and benefit by transfer of experience, interacting directly with the learning domain. This paper describes the Virtual Multi Flow Graph (Virtual-MFG) graphical formal model and the Interaction Specification Workspace (ISW) software architecture for the interaction specification and design of VR applications with emphasis on educational software. The interaction designer specifies the interaction issues of the final system formally, using the tools of ISW. The virtual microworld’s objects database is updated with these interaction specifications which include both the virtual objects ’ dynamic properties and their tutoring capabilities. The model is validated by applying it on an existing VR educational software (EIKON). The Virtual-MFG graphs specifying a learning scenario of EIKON along with the application of ...
Algorithms, 2018
Semi-supervised learning algorithms have become a topic of significant research as an alternative... more Semi-supervised learning algorithms have become a topic of significant research as an alternative to traditional classification methods which exhibit remarkable performance over labeled data but lack the ability to be applied on large amounts of unlabeled data. In this work, we propose a new semi-supervised learning algorithm that dynamically selects the most promising learner for a classification problem from a pool of classifiers based on a self-training philosophy. Our experimental results illustrate that the proposed algorithm outperforms its component semi-supervised learning algorithms in terms of accuracy, leading to more efficient, stable and robust predictive models.

Machine Learning and Knowledge Extraction, 2019
Speech recognition has various applications including human to machine interaction, sorting of te... more Speech recognition has various applications including human to machine interaction, sorting of telephone calls by gender categorization, video categorization with tagging and so on. Currently, machine learning is a popular trend which has been widely utilized in various fields and applications, exploiting the recent development in digital technologies and the advantage of storage capabilities from electronic media. Recently, research focuses on the combination of ensemble learning techniques with the semi-supervised learning framework aiming to build more accurate classifiers. In this paper, we focus on gender recognition by voice utilizing a new ensemble semi-supervised self-labeled algorithm. Our preliminary numerical experiments demonstrate the classification efficiency of the proposed algorithm in terms of accuracy, leading to the development of stable and robust predictive models.

Algorithms, 2018
Length of stay of hospitalized patients is generally considered to be a significant and critical ... more Length of stay of hospitalized patients is generally considered to be a significant and critical factor for healthcare policy planning which consequently affects the hospital management plan and resources. Its reliable prediction in the preadmission stage could further assist in identifying abnormality or potential medical risks to trigger additional attention for individual cases. Recently, data mining and machine learning constitute significant tools in the healthcare domain. In this work, we introduce a new decision support software for the accurate prediction of hospitalized patients’ length of stay which incorporates a novel two-level classification algorithm. Our numerical experiments indicate that the proposed algorithm exhibits better classification performance than any examined single learning algorithm. The proposed software was developed to provide assistance to the hospital management and strengthen the service system by offering customized assistance according to patien...
Next Generation Environments
A Virtual Learning Environment or Learning Management System (VLE or LMS) is a software system or... more A Virtual Learning Environment or Learning Management System (VLE or LMS) is a software system or integrated platform that contains a series of services and tools to support a number of activities and course management procedures (Ho, Higson, Dey & Xu, 2009). Nowadays numerous LMS are available in the market; a few more, like Moodle, Sakai, Atutor, Claroline, etc., are under development by the open source community. Another category of Learning Systems is the so called Learning Design systems (Britain, 2004). This category includes systems like LAMS (Dalziel, 2003) and Coppercore (Vogten et al., 2007). Despite the many tools and services offered by the

Proteins are constructed by the combination of a different number of amino acids and thus, have a... more Proteins are constructed by the combination of a different number of amino acids and thus, have a different structure and folding depending on chemical reactions and other aspects. The protein folding prediction can help in many healthcare scenarios to foretell and prevent diseases. The different elements that form a protein give the secondary structure. One of the most common algorithms used for secondary structure prediction constitutes the Chou-Fasman method. This technique divides and in following analyses each amino acid in three different elements, which are Open image in new window -helices, Open image in new window -sheets and turns based on already known protein structures. Its aim is to predict the probability for which each of these elements will be formed. In this paper, we have used Chou-Fasman algorithm for extracting the probabilities of a series of amino acids in FASTA format. We make an analysis given all probabilities for any length of a human protein without any r...

Sensors
Deep convolutional neural networks have shown remarkable performance in the image classification ... more Deep convolutional neural networks have shown remarkable performance in the image classification domain. However, Deep Learning models are vulnerable to noise and redundant information encapsulated into the high-dimensional raw input images, leading to unstable and unreliable predictions. Autoencoders constitute an unsupervised dimensionality reduction technique, proven to filter out noise and redundant information and create robust and stable feature representations. In this work, in order to resolve the problem of DL models’ vulnerability, we propose a convolutional autoencoder topological model for compressing and filtering out noise and redundant information from initial high dimensionality input images and then feeding this compressed output into convolutional neural networks. Our results reveal the efficiency of the proposed approach, leading to a significant performance improvement compared to Deep Learning models trained with the initial raw images.

Proceedings of the 8th International Conference on Software Development and Technologies for Enhancing Accessibility and Fighting Info-exclusion
Anxiety disorders are a type of mental disorders characterized by important feelings of fear and ... more Anxiety disorders are a type of mental disorders characterized by important feelings of fear and anxiety. Recently, the evolution of machine learning techniques has helped greatly to develop tools assisting doctors to predict mental disorders and support patient care. In this work, a comparative literature search was conducted on research for the prediction of speci c types of anxiety disorders, using machine learning techniques. Sixteen (16) studies were selected and examined, revealing that machine learning techniques can be used for e ectively predicting anxiety disorders. The accuracy of the results varies according to the type of anxiety disorder and the type of methods utilized for predicting the disorder. We can deduce that signi cant work has been done on the prediction of anxiety using machine learning techniques. However, in the future we may achieve higher accuracy scores and that could lead to a better treatment support for patients. CCS CONCEPTS • Theory of computation → Machine learning theory; • Applied computing → Health care information systems;
Modern ICT has evolved through the years and is now in position of delivering educational content... more Modern ICT has evolved through the years and is now in position of delivering educational content to specific target groups in remote locations. Advanced e-learning techniques are now used not only for delivering content to high school and university students, but can be used in lifelong training programs. Due to the target group most of these programs have (i.e. professionals with little time to spend, people of a certain age with reduced ICT skills, etc.), it is vital that organizations choose wisely among the many Learning Management Systems currently available. The purpose of this chapter is to describe and examine the features of such a platform. DIPLEK is a platform developed using service oriented architecture to enable easy access to educational content and activities for novice learners and instructors.
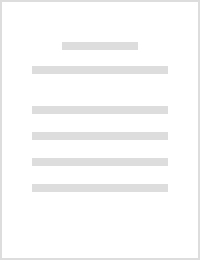
Advances in Virtual Reality (VR) Systems, Intelligent Tutoring Systems and Agent Technology make ... more Advances in Virtual Reality (VR) Systems, Intelligent Tutoring Systems and Agent Technology make it possible to design and develop Virtual Training Environments, where the trainees can immerse themselves and interact directly with the learning domain. This paper presents the Virtual Reality-MFG formal model for the specification of interaction and the design of Virtual Reality Agent-based Training Applications. VR-MFG gives the interaction designer the capability to apply user-centered design and adopt alternatively a task-based or a goal-oriented approach, for the design of a specific Virtual Training Environment (VE). Moreover, allows the designer to split the design effort for any VR Training Environment among a number of multiple micro-worlds that constitute the entire application. Finally, as a case study of VR-MFG application, the interaction aspects of DrIVE virtual environment are specified.

Mathematics
Complex networks constitute a new field of scientific research that is derived from the observati... more Complex networks constitute a new field of scientific research that is derived from the observation and analysis of real-world networks, for example, biological, computer and social ones. An important subset of complex networks is the biological, which deals with the numerical examination of connections/associations among different nodes, namely interfaces. These interfaces are evolutionary and physiological, where network epidemic models or even neural networks can be considered as representative examples. The investigation of the corresponding biological networks along with the study of human diseases has resulted in an examination of networks regarding medical supplies. This examination aims at a more profound understanding of concrete networks. Fuzzy logic is considered one of the most powerful mathematical tools for dealing with imprecision, uncertainties and partial truth. It was developed to consider partial truth values, between completely true and completely false, and aims...
Engineering Applications of Neural Networks

IFIP Advances in Information and Communication Technology
In last decade, cryptocurrency has emerged in financial area as a key factor in businesses and fi... more In last decade, cryptocurrency has emerged in financial area as a key factor in businesses and financial market opportunities. Accurate predictions can assist cryptocurrency investors towards right investing decisions and lead to potential increased profits. Additionally, they can also support policy makers and financial researchers in studying cryptocurrency markets behavior. Nevertheless, cryptocurrency price prediction is considered a very challenging task, due to its chaotic and very complex nature. In this study we evaluate some of the most successful and widely used deep learning algorithms forecasting cryptocurrency prices. The results obtained, provide significant evidence that deep learning models are not able to solve this problem efficiently and effectively. Conducting detailed experimentation and results analysis, we conclude that it is essential to invent and incorporate new techniques, strategies and alternative approaches such as: more sophisticated prediction algorithms, advanced ensemble methods, feature engineering techniques and other validation metrics.

Electronics
Nowadays, cryptocurrencies are established and widely recognized as an alternative exchange curre... more Nowadays, cryptocurrencies are established and widely recognized as an alternative exchange currency method. They have infiltrated most financial transactions and as a result cryptocurrency trade is generally considered one of the most popular and promising types of profitable investments. Nevertheless, this constantly increasing financial market is characterized by significant volatility and strong price fluctuations over a short-time period therefore, the development of an accurate and reliable forecasting model is considered essential for portfolio management and optimization. In this research, we propose a multiple-input deep neural network model for the prediction of cryptocurrency price and movement. The proposed forecasting model utilizes as inputs different cryptocurrency data and handles them independently in order to exploit useful information from each cryptocurrency separately. An extensive empirical study was performed using three consecutive years of cryptocurrency dat...
Medical & Biological Engineering & Computing

Journal of Imaging
Image classification is a very popular machine learning domain in which deep convolutional neural... more Image classification is a very popular machine learning domain in which deep convolutional neural networks have mainly emerged on such applications. These networks manage to achieve remarkable performance in terms of prediction accuracy but they are considered as black box models since they lack the ability to interpret their inner working mechanism and explain the main reasoning of their predictions. There is a variety of real world tasks, such as medical applications, in which interpretability and explainability play a significant role. Making decisions on critical issues such as cancer prediction utilizing black box models in order to achieve high prediction accuracy but without provision for any sort of explanation for its prediction, accuracy cannot be considered as sufficient and ethnically acceptable. Reasoning and explanation is essential in order to trust these models and support such critical predictions. Nevertheless, the definition and the validation of the quality of a ...
Uploads
Papers by Panagiotis E Pintelas