Papers by Yonatan Loewenstein
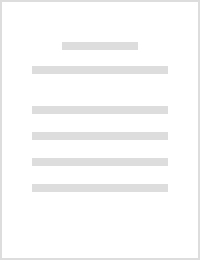
It is now widely believed that decisions are guided by a small number of internal subjective vari... more It is now widely believed that decisions are guided by a small number of internal subjective variables that determine choice preference. The process of learning manifests as a change in the state of these variables. It is not clear how to find the neural correlates of these variables, in particular because their state cannot be directly measured or controlled by the experimenter. Rather, these variables reflect the history of the subject’s actions and reward experience. We seek to construct a behavioral model that captures the dynamics of learning and decision making, such that the internal variables of this model will serve as a proxy for the subjective variables. We use the theory of reinforcement learning in order to find a behavioral model that best captures the learning dynamics of monkeys in a two-armed bandit reward schedule. We consider two families of learning algorithms: value function estimation and direct policy optimization. In the former, the values of the alternative ...
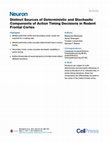
SUMMARYThe selection and timing of actions are subject to determinate influences such as sensory ... more SUMMARYThe selection and timing of actions are subject to determinate influences such as sensory cues and internal state as well as to effectively stochastic variability. Although stochastic choice mechanisms are assumed by many theoretical models, their origin and mechanisms remain poorly understood. Here we investigated this issue by studying how neural circuits in the frontal cortex determine action timing in rats performing a waiting task. Electrophysiological recordings from two regions necessary for this behavior, medial prefrontal cortex (mPFC) and secondary motor cortex (M2), revealed an unexpected functional dissociation. Both areas encoded deterministic biases in action timing, but only M2 neurons reflected stochastic trial-by-trial fluctuations. This differential coding was reflected in distinct timescales of neural dynamics in the two frontal cortical areas. These results suggest a two-stage model in which stochastic components of action timing decisions are injected by ...
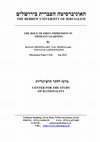
J Exp Psychol Gen., 2013
We quantified the effect of first experience on behavior in operant learning and studied its unde... more We quantified the effect of first experience on behavior in operant learning and studied its underlying computational principles. To that goal, we analyzed more than 200,000 choices in a repeated-choice experiment. We found that the outcome of the first experience has a substantial and lasting effect on participants' subsequent behavior, which we term outcome primacy. We found that this outcome primacy can account for much of the underweighting of rare events, where participants apparently underestimate small probabilities. We modeled behavior in this task using a standard, model-free reinforcement learning algorithm. In this model, the values of the different actions are learned over time and are used to determine the next action according to apredefined action-selection rule. We used a novel non-parametric method to characterize this action-selection rule and showed that the substantial effect of first experinece on behavior is consistent with the reinforcment learning model if we assume that the outcome of first experience resets the values of the experienced actions, but not if we assume arbitrary initial conditions. Moreover, the predictive power of our resetting model outperforms previouly published models regarding the aggregate choice behavior. These findings suggest that first experience has a disproportionately large effect on subsequent actions, similar to primacy effects in other fields of cognitive psychology. The mechanism of resetting of the initial conditions which underlies outcome primacy may thus also account for other forms of primacy.
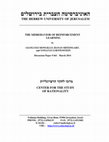
Proceedings of the IEEE, 2014
Organisms modify their behavior in response to its consequences, a phenomenon referred to as oper... more Organisms modify their behavior in response to its consequences, a phenomenon referred to as operant learning. The computational principles and neural mechanisms underlying operant learning are a subject of extensive experimental and theoretical investigations. Theoretical approaches largely rely on concepts and algorithms from Reinforcement Learning. The dominant view is that organisms maintain a value function, that is a set of estimates of the cumulative future rewards associated with the different behavioral options. These values are then used to select actions. Learning in this framework results from the update of these values depending on experience of the consequences of past actions. An alternative view questions the applicability of such a computational scheme to many real-life situations. Instead, it posits that organisms exploit the intrinsic variability in their action selection mechanism(s) to modify their behavior, e.g., via stochastic gradient ascent, without the need of an explicit representation of values. In this review, we compare these two approaches in terms of their computational power and flexibility, their putative neural correlates and, finally, in terms of their ability to account for behavior as observed in repeated-choice experiments. We discuss the successes and failures of these alternative approaches in explaining the observed patterns of choice behavior. We conclude by identifying some of the important challenges to a comprehensive theory of operant learning.
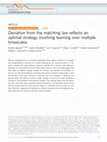
Nature Communications
Behavior deviating from our normative expectations often appears irrational. For example, even th... more Behavior deviating from our normative expectations often appears irrational. For example, even though behavior following the so-called matching law can maximize reward in a stationary foraging task, actual behavior commonly deviates from matching. Such behavioral deviations are interpreted as a failure of the subject; however, here we instead suggest that they reflect an adaptive strategy, suitable for uncertain, non-stationary environments. To prove it, we analyzed the behavior of primates that perform a dynamic foraging task. In such nonstationary environment, learning on both fast and slow timescales is beneficial: fast learning allows the animal to react to sudden changes, at the price of large fluctuations (variance) in the estimates of task relevant variables. Slow learning reduces the fluctuations but costs a bias that causes systematic behavioral deviations. Our behavioral analysis shows that the animals solved this bias-variance tradeoff by combining learning on both fast and slow timescales, suggesting that learning on multiple timescales can be a biologically plausible mechanism for optimizing decisions under uncertainty.
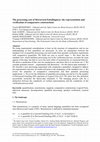
ZAS Papers in Linguistics
We bring experimental considerations to bear on the structure of comparatives and on ourunderstan... more We bring experimental considerations to bear on the structure of comparatives and on ourunderstanding of how quantifiers are processed. At issue are mismatches between thestandard view of quantifier processing cost and results from speeded verification experimentswith comparative quantifiers. We build our case in several steps: 1. We show that thestandard view, which attributes processing cost to the verification process, accounts for someaspects of the data, but fails to cover the main effect of monotonicity on measured behavior.We derive a prediction of this view for comparatives, and show that it is not borne out. 2. Weconsider potential reasons – experimental and theoretical – for this theory-data mismatch. 3.We describe a new processing experiment with comparative quantifiers, designed to addressthe experimental concerns. Its results still point to the inadequacy of the standard view. 4. Wereview the semantics of comparative constructions and their potential processingimplicati...
Current Opinion in Behavioral Sciences
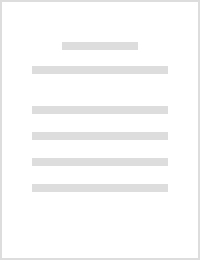
SUMMARYThe mounting evidence for the involvement of astrocytes in neuronal circuits function and ... more SUMMARYThe mounting evidence for the involvement of astrocytes in neuronal circuits function and behavior stands in stark contrast to the lack of detailed anatomical description of these cells and the neurons in their domains. To fill this void, we imaged >30,000 astrocytes in cleared hippocampi, and employed converging genetic, histological and computational tools to determine the elaborate structure, distribution and neuronal content of astrocytic domains. First, we characterized the spatial distribution of >19,000 astrocytes across CA1 lamina, and analyzed the detailed morphology of thousands of reconstructed domains. We then determined the excitatory content of CA1 astrocytes, averaging above 13 pyramidal neurons per domain and increasing towards CA1 midline. Finally, we discovered that somatostatin neurons are found in close proximity to astrocytes, compared to parvalbumin and VIP inhibitory neurons. This resource expands our understanding of fundamental hippocampal desig...
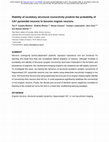
Neurons undergoing activity-dependent plasticity represent experience and are functional for lear... more Neurons undergoing activity-dependent plasticity represent experience and are functional for learning and recall thus they are considered cellular engrams of memory. Although increase in excitability and stability of structural synaptic connectivity have been implicated in the formation and persistance of engrams, the mechanisms bringing engrams into existence are still largely unknown. To investigate this issue, we tracked the dynamics of structural excitatory synaptic connectivity of hippocampal CA1 pyramidal neurons over two weeks using deep-brain two-photon imaging in live mice. We found that neurons that will prospectively become part of an engram display higher stability of connectivity than neurons that will not. A novel experience significantly stabilizes the connectivity of non-engram neurons. Finally, the density and survival of dendritic spines negatively correlates to freezing to the context but not to the tone in a trace fear conditioning learning paradigm.
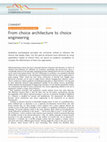
Nature Communications
Qualitative psychological principles are commonly utilized to influence the choices that people m... more Qualitative psychological principles are commonly utilized to influence the choices that people make. Can this goal be achieved more efficiently by using quantitative models of choice? Here, we launch an academic competition to compare the effectiveness of these two approaches. Influencing human choices has been a principal objective of parents and educators, as well as of salesmen and politicians for millennia. In economics, psychology and neuroscience, there is considerable interest in the principles underlying decision-making and in the ways in which they can be used to bias human choice. The 2017 Nobel prize in economics, was awarded to Richard Thaler for his contributions to the development of behavioral economics and its applications to policy-making. Thaler coined the term choice architecture to describe how insights from behavioral economics can be used to nudge choices without changing their objective values 1. Choice architecture utilizes qualitative psychological principles to shape behavior. Can this goal be more effectively achieved using quantitative models? In the natural sciences, quantitative models underlay the development of engineering. Therefore, we ask whether quantitative models can revolutionize the field of choice architecture into choice engineering, defined as the use of quantitative models to shape choice behavior. Both qualitative principles and quantitative models identify factors that affect behavior. The difference between them is that the latter, but not the former, quantitatively describe the magnitudes of the effects. Operant learning is a process, in which the strength or likelihood of a behavior is modified by rewards and punishments. We demonstrate the difference between choice architecture and choice engineering in the framework of an operant learning task. Consider an objective function of maximally biasing choices in favor of a predefined alternative (defined here as alternative 1) in a repeated, two-alternative forced-choice task with binary rewards (Fig. 1a). How should a choice architect and a choice engineer allocate the rewards in view of this goal? Thorndike's Law of Effect is a qualitative description of operant learning: "Of several responses made to the same situation, those which are accompanied or closely followed by satisfaction to the animal … will be more likely to recur" 2. In line with this law of behavior, a choice architect (and common-sense intuition) would recommend allocating all available rewards to the desired alternative 1 (Fig. 1b). Subtler principles of choice become necessary if we add constraints, e.g., that the number of rewards that can be allocated to alternative 1 is limited. For a real-life example, assume that you organize a seminar course, and invite each week a different guest lecturer. Having taught the course in the past you know in advance, which lectures will be more interesting. How should you distribute those
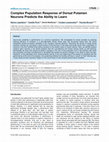
PLoS ONE, 2013
Day-to-day variability in performance is a common experience. We investigated its neural correlat... more Day-to-day variability in performance is a common experience. We investigated its neural correlate by studying learning behavior of monkeys in a two-alternative forced choice task, the two-armed bandit task. We found substantial session-to-session variability in the monkeys' learning behavior. Recording the activity of single dorsal putamen neurons we uncovered a dual function of this structure. It has been previously shown that a population of neurons in the DLP exhibits firing activity sensitive to the reward value of chosen actions. Here, we identify putative medium spiny neurons in the dorsal putamen that are cue-selective and whose activity builds up with learning. Remarkably we show that session-to-session changes in the size of this population and in the intensity with which this population encodes cue-selectivity is correlated with session-to-session changes in the ability to learn the task. Moreover, at the population level, dorsal putamen activity in the very beginning of the session is correlated with the performance at the end of the session, thus predicting whether the monkey will have a "good" or "bad" learning day. These results provide important insights on the neural basis of inter-temporal performance variability. Citation: Laquitaine S, Piron C, Abellanas D, Loewenstein Y, Boraud T (2013) Complex Population Response of Dorsal Putamen Neurons Predicts the Ability to Learn. PLoS ONE 8(11): e80683.
Nature Neuroscience, 2006
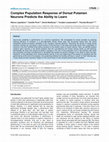
PLoS ONE, 2013
Day-to-day variability in performance is a common experience. We investigated its neural correlat... more Day-to-day variability in performance is a common experience. We investigated its neural correlate by studying learning behavior of monkeys in a two-alternative forced choice task, the two-armed bandit task. We found substantial session-to-session variability in the monkeys' learning behavior. Recording the activity of single dorsal putamen neurons we uncovered a dual function of this structure. It has been previously shown that a population of neurons in the DLP exhibits firing activity sensitive to the reward value of chosen actions. Here, we identify putative medium spiny neurons in the dorsal putamen that are cue-selective and whose activity builds up with learning. Remarkably we show that session-to-session changes in the size of this population and in the intensity with which this population encodes cue-selectivity is correlated with session-to-session changes in the ability to learn the task. Moreover, at the population level, dorsal putamen activity in the very beginning of the session is correlated with the performance at the end of the session, thus predicting whether the monkey will have a "good" or "bad" learning day. These results provide important insights on the neural basis of inter-temporal performance variability. Citation: Laquitaine S, Piron C, Abellanas D, Loewenstein Y, Boraud T (2013) Complex Population Response of Dorsal Putamen Neurons Predicts the Ability to Learn. PLoS ONE 8(11): e80683.
Uploads
Papers by Yonatan Loewenstein